Utility of language model and physics-based approaches in modifying MHC Class-I immune-visibility for the design of vaccines and therapeutics
bioRxiv (Cold Spring Harbor Laboratory)(2023)
Abstract
Protein therapeutics already have an arsenal of applications that include disrupting protein interactions, acting as potent vaccines, and replacing genetically deficient proteins. Therapeutics must avoid triggering unwanted immune responses towards the therapeutic protein or viral vector proteins. In contrast, vaccines must support a robust immune reaction targeting a broad range of pathogen variants. Therefore, computational methods modifying proteins' immune-visibility without disrupting function are needed. Many immune sub-systems interact with disease to detect destruction targets, and here we focus on well-understood cytotoxic T-lymphocytes that recognize short peptides presented via the MHC Class I pathway. To explore the limits of modifying MHC-I immune-visibility within the distribution of naturally occurring sequences, we developed a novel machine learning technique, CAPE-XVAE, that combines a language model with reinforcement learning to modify a protein's immune-visibility. Our results show that CAPE-XVAE effectively modifies the MHC-I immune-visibility of the HIV Nef protein. We contrast CAPE-XVAE to CAPE-Packer, a physics-based method we also developed, and observe that while the machine learning approach generates more natural appearing sequences, the physics-based approach can reach greater extremes of immune-visibility. Compared to CAPE-Packer, CAPE-XVAE suggests sequences that draw upon local sequence similarities in the training set. This is beneficial for vaccine development, where the synthetic sequence should be representative of the real viral population. Additionally, the language model approach holds promise for preserving both known and unknown functional constraints, which are essential for the immune-modulation of therapeutic proteins. In contrast, CAPE-Packer, emphasizes preserving the protein's overall fold, falling short of capturing the diversity of viral variants available to learn from.
MoreTranslated text
Key words
vaccines,language model,physics-based,immune-visibility
AI Read Science
Must-Reading Tree
Example
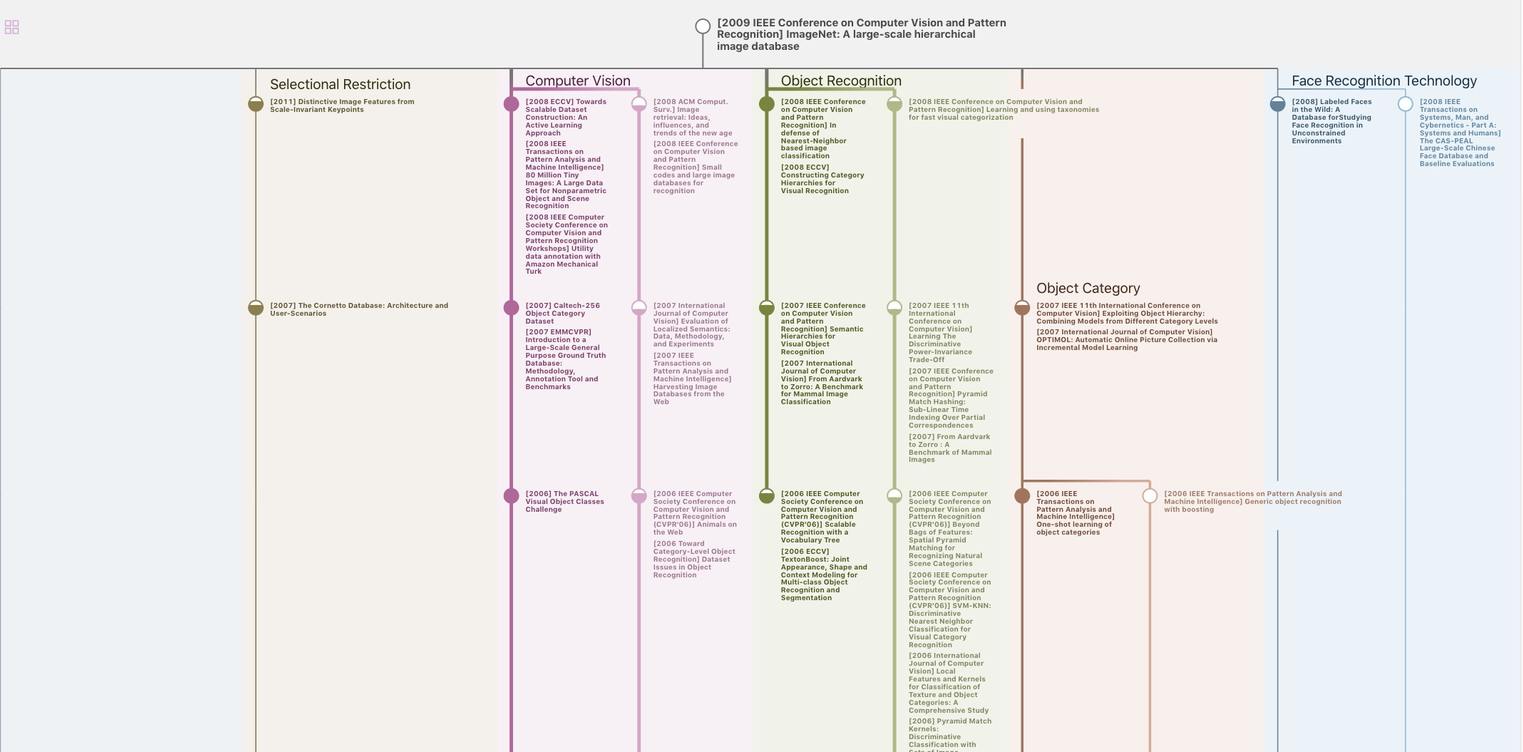
Generate MRT to find the research sequence of this paper
Chat Paper
Summary is being generated by the instructions you defined