Multi Sequential Embedding-based Cross-Domain Recommendation
Research Square (Research Square)(2023)
摘要
In the real world, users interact with various items in a sequential and multi-behavior manner. Cross-domain recommendations (CDRs) usually transfer knowledge across different domains to improve their performance in a target domain. We propose a novel Multi Sequential Embedding for Cross-Domain Recommendation named MSECDR. Exploiting Doc2Vec, it reflects the sequentiality of user-item interactions in multiple domains on user and item modeling. In particular, this approach enables us to learn three types of item relationships (named intra-/inter- and other interaction sequences) simultaneously for representation learning. In addition, a deep neural network structure is introduced to combine the linearity and non-linearity of user-item interactions.Extensive experiments with six domain datasets from two real-world services (Amazon and TripAdvisor) demonstrate that MSECDR outperforms the state-of-the-art for single and cross-domain recommendations. Furthermore, experimental results show that MSECDR can effectively and efficiently adapt to CDRs fusing more domains.
更多查看译文
关键词
recommendation,embedding-based,cross-domain
AI 理解论文
溯源树
样例
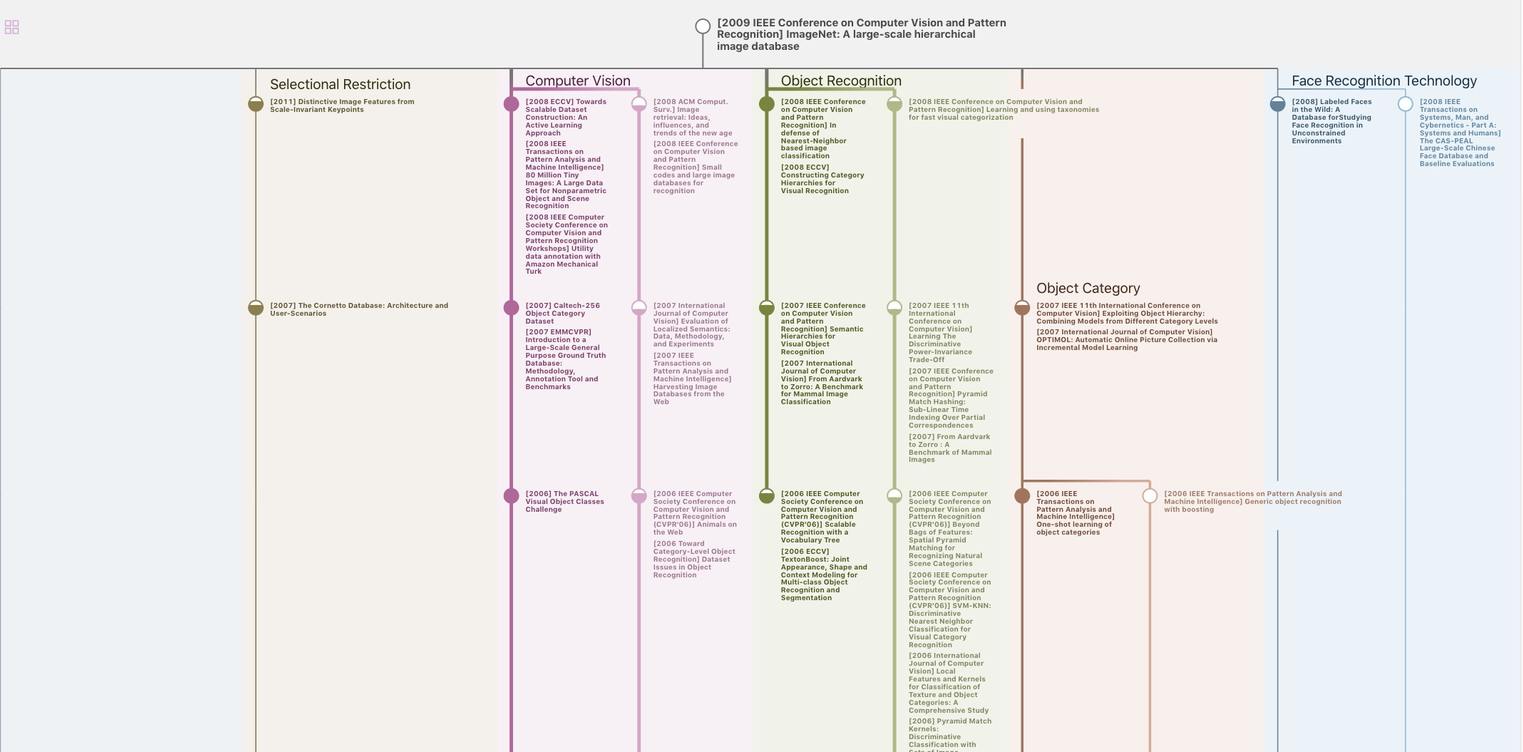
生成溯源树,研究论文发展脉络
Chat Paper
正在生成论文摘要