A new retinal OCT‐angiography diabetic retinopathy dataset for segmentation and DR grading
Journal of Biophotonics(2023)
Abstract
Abstract Purpose Diabetic retinopathy (DR) is one of the most common diseases caused by diabetes and can lead to vision loss or even blindness. The wide‐field optical coherence tomography (OCT) angiography is non‐invasive imaging technology and convenient to diagnose DR. Methods A newly constructed R etinal O CT‐ A ngiography D iabetic retinopathy (ROAD) dataset is utilized for segmentation and grading tasks. It contains 1200 normal images, 1440 DR images, and 1440 ground truths for DR image segmentation. To handle the problem of grading DR, we propose a novel and effective framework, named projective map attention‐based convolutional neural network (PACNet). Results The experimental results demonstrate the effectiveness of our PACNet. The accuracy of the proposed framework for grading DR is 87.5% on the ROAD dataset. Conclusions The information on ROAD can be viewed at URL https://mip2019.github.io/ROAD . The ROAD dataset will be helpful for the development of the early detection of DR field and future research. Translational Relevance The novel framework for grading DR is a valuable research and clinical diagnosis method.
MoreTranslated text
Key words
diabetic retinopathy dataset,<scp>oct‐angiography</scp>,segmentation
AI Read Science
Must-Reading Tree
Example
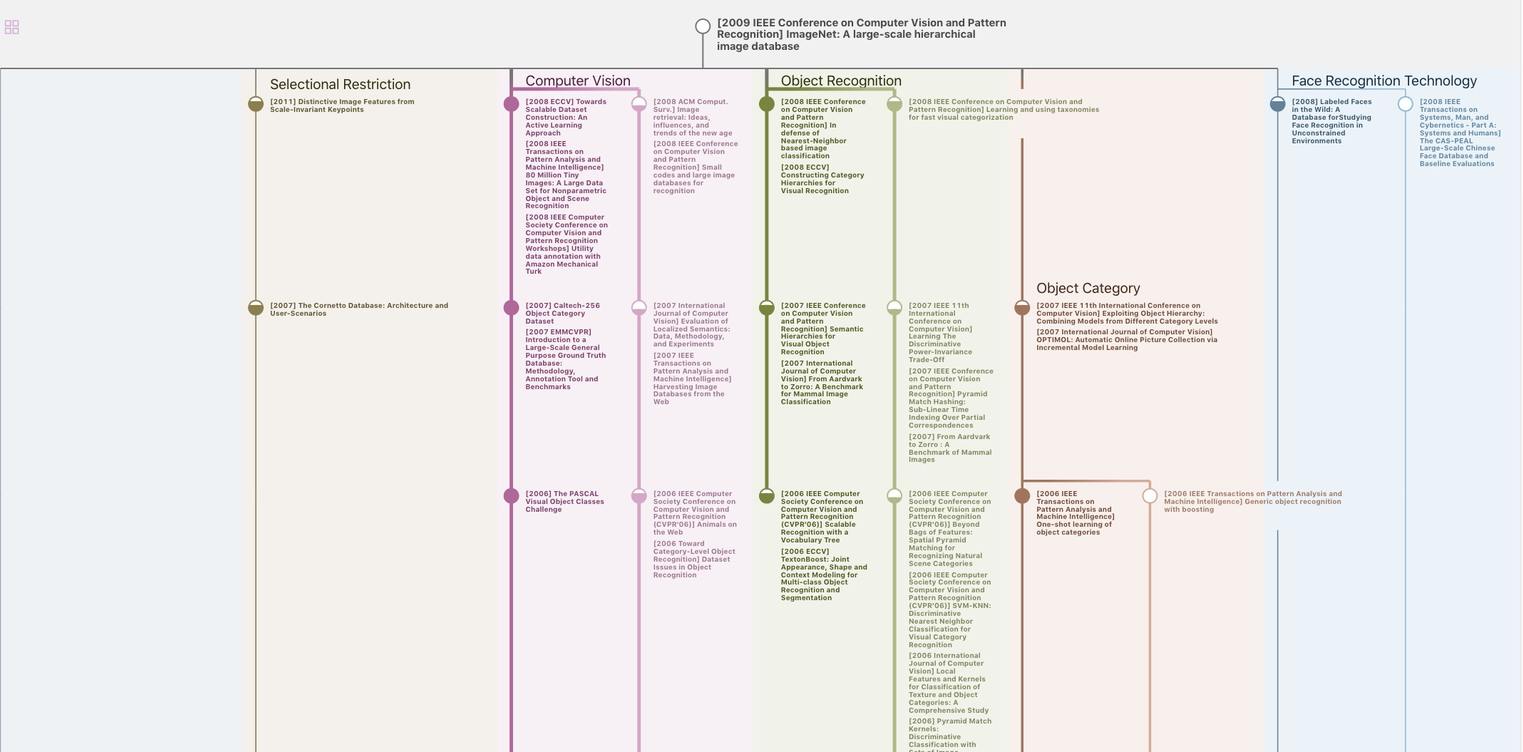
Generate MRT to find the research sequence of this paper
Chat Paper
Summary is being generated by the instructions you defined