Machine Learning Approach for Screening Cochlear Implant Candidates: Comparing With the 60/60 Guideline
Otology & Neurotology(2023)
摘要
To develop a machine learning-based referral guideline for patients undergoing cochlear implant candidacy evaluation (CICE) and to compare with the widely used 60/60 guideline.Retrospective cohort.Tertiary referral center.772 adults undergoing CICE from 2015 to 2020.Variables included demographics, unaided thresholds, and word recognition score. A random forest classification model was trained on patients undergoing CICE, and bootstrap cross-validation was used to assess the modeling approach's performance.The machine learning-based referral tool was evaluated against the 60/60 guideline based on ability to identify CI candidates under traditional and expanded criteria.Of 587 patients with complete data, 563 (96%) met candidacy at our center, and the 60/60 guideline identified 512 (87%) patients. In the random forest model, word recognition score; thresholds at 3000, 2000, and 125; and age at CICE had the largest impact on candidacy (mean decrease in Gini coefficient, 2.83, 1.60, 1.20, 1.17, and 1.16, respectively). The 60/60 guideline had a sensitivity of 0.91, a specificity of 0.42, and an accuracy of 0.89 (95% confidence interval, 0.86-0.91). The random forest model obtained higher sensitivity (0.96), specificity (1.00), and accuracy (0.96; 95% confidence interval, 0.95-0.98). Across 1,000 bootstrapped iterations, the model yielded a median sensitivity of 0.92 (interquartile range [IQR], 0.85-0.98), specificity of 1.00 (IQR, 0.88-1.00), accuracy of 0.93 (IQR, 0.85-0.97), and area under the curve of 0.96 (IQR, 0.93-0.98).A novel machine learning-based screening model is highly sensitive, specific, and accurate in predicting CI candidacy. Bootstrapping confirmed that this approach is potentially generalizable with consistent results.
更多查看译文
关键词
screening cochlear implant candidates
AI 理解论文
溯源树
样例
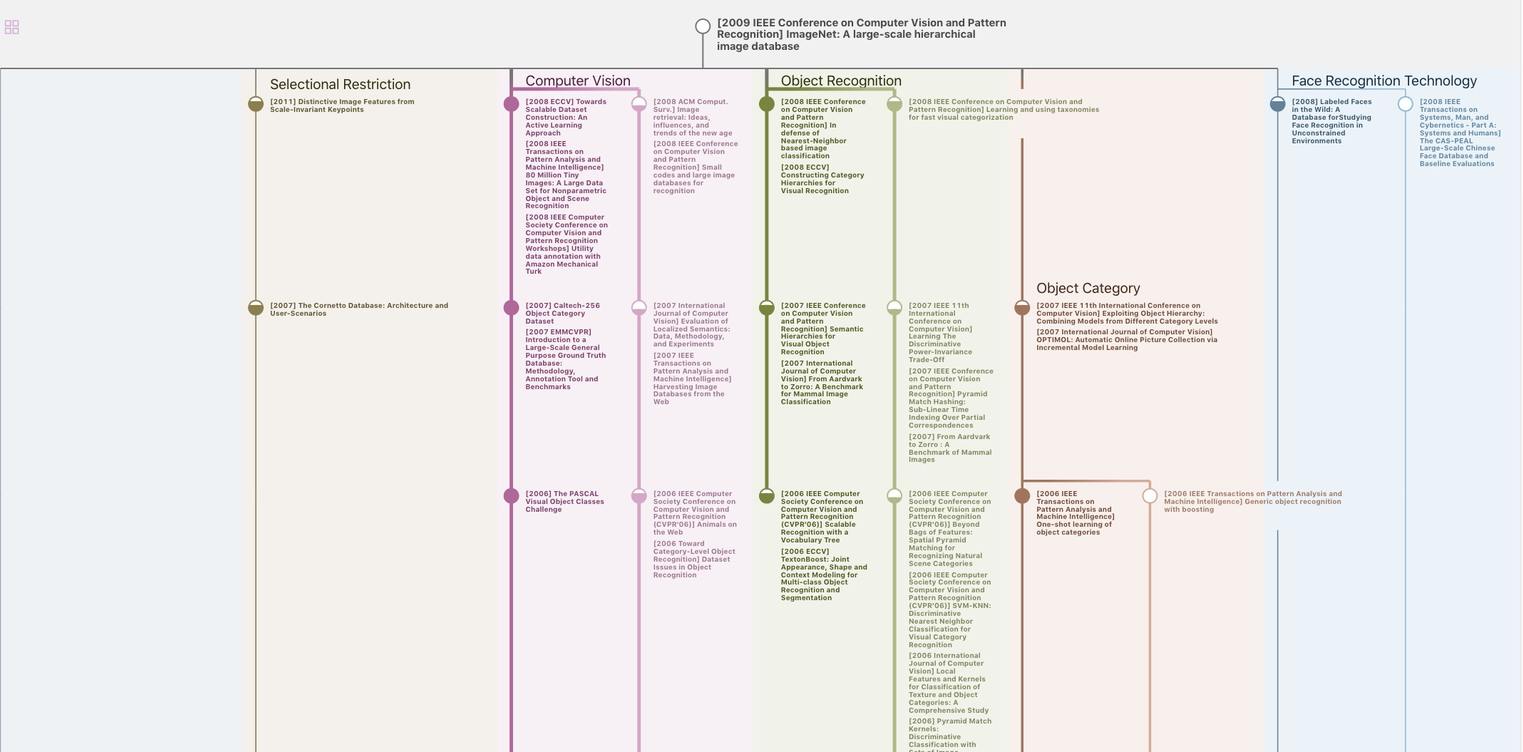
生成溯源树,研究论文发展脉络
Chat Paper
正在生成论文摘要