Modeling Multiple Aesthetic Views for Series Photo Selection
IEEE TRANSACTIONS ON MULTIMEDIA(2024)
摘要
Numerous photos are taken in daily life, and sorting them is laborious and time consuming. The large number of similar images exacerbates the difficulty of album management, under this scenario, serial photo selection (SPS) emerges. As an important branch of image aesthetic quality assessment, it focuses on identifying the best image among a series of almost identical photos. Currently, most existing SPS methods focus only on extracting features from the original image, while neglecting the fact that multiple views of the image can provide much more detailed aesthetic information. In this article, we propose a Siamese network structure called SPSNet to enhance the representation learning of multi-view features by acquiring the depth, generic, and handcrafted features of images. In specific, we implement a parallel structure to extract deep and shallow features, fusing local and global representations at different resolutions interactively. The aggregation of multiple views of image via a self-attentive module with adaptive weights enables the model to discriminate the importance of each view. Moreover, we employ a graph neural network to construct the relationships among the multi-view features. Our proposed method, which is trained by a Siamese network, can effectively distinguish the nuances of similar images, and thus, select the best one from a series of almost identical photos. Extensive experiments conducted on the aesthetic dataset demonstrate that our method outperforms other state-of-the-art SPS methods, which achieves the 75.36% accuracy on the Phototriage dataset. Besides, our model is up to 3.04% better than the baseline methods in terms of the average accuracy.
更多查看译文
关键词
Feature extraction,Transformers,Convolutional neural networks,Quality assessment,Visualization,Task analysis,Representation learning,Image aesthetic assessment,series photo selection,multi-view learning,visual attention,graph neural network
AI 理解论文
溯源树
样例
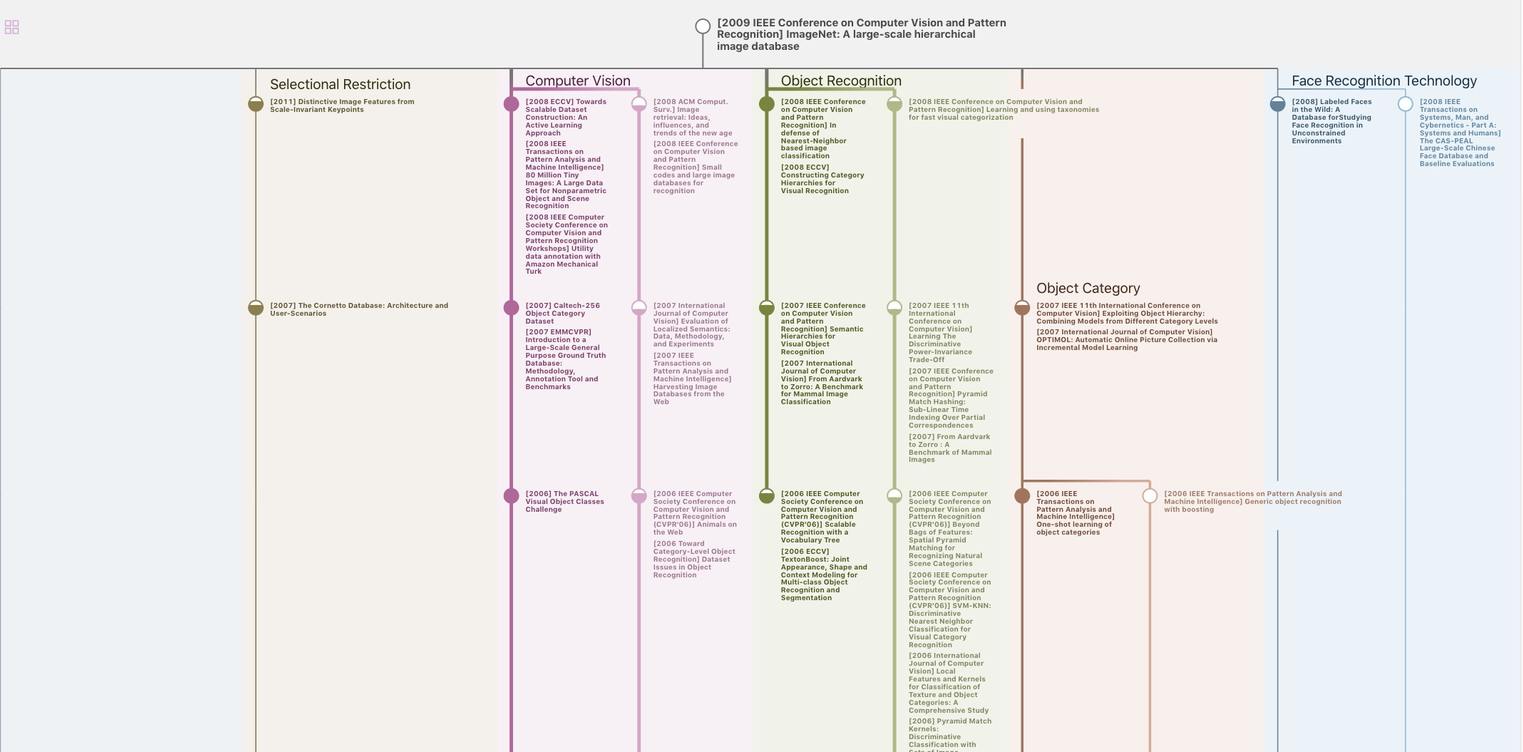
生成溯源树,研究论文发展脉络
Chat Paper
正在生成论文摘要