RedMotion: Motion Prediction via Redundancy Reduction
arXiv (Cornell University)(2023)
摘要
Predicting the future motion of traffic agents is vital for self-driving vehicles to ensure their safe operation. We introduce RedMotion, a transformer model for motion prediction that incorporates two types of redundancy reduction. The first type of redundancy reduction is induced by an internal transformer decoder and reduces a variable-sized set of road environment tokens, such as road graphs with agent data, to a fixed-sized embedding. The second type of redundancy reduction is a self-supervised learning objective and applies the redundancy reduction principle to embeddings generated from augmented views of road environments. Our experiments reveal that our representation learning approach can outperform PreTraM, Traj-MAE, and GraphDINO in a semi-supervised setting. Our RedMotion model achieves results that are competitive with those of Scene Transformer or MTR++. We provide an open source implementation that is accessible via GitHub (https://github.com/kit-mrt/red-motion) and Colab (https://colab.research.google.com/drive/1Q-Z9VdiqvfPfctNG8oqzPcgm0lP3y1il).
更多查看译文
关键词
redmotion prediction,redundancy
AI 理解论文
溯源树
样例
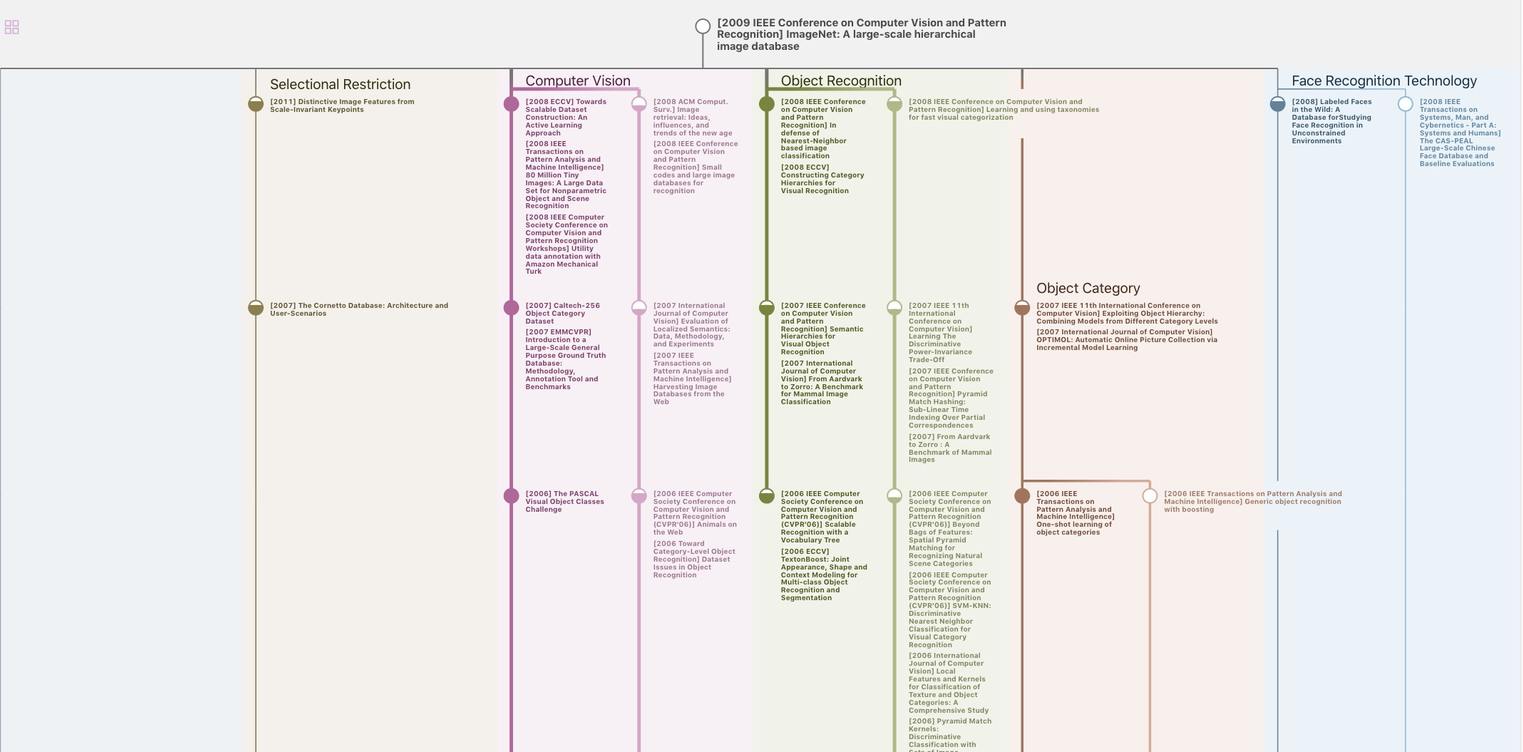
生成溯源树,研究论文发展脉络
Chat Paper
正在生成论文摘要