Dual Vision Transformer
IEEE Transactions on Pattern Analysis and Machine Intelligence(2023)
摘要
Recent advances have presented several strategies to mitigate the computations of self-attention mechanism with high-resolution inputs. Many of these works consider decomposing the global self-attention procedure over image patches into regional and local feature extraction procedures that each incurs a smaller computational complexity. Despite good efficiency, these approaches seldom explore the holistic interactions among all patches, and are thus difficult to fully capture the global semantics. In this paper, we propose a novel Transformer architecture that elegantly exploits the global semantics for self-attention learning, namely Dual Vision Transformer (Dual-ViT). The new architecture incorporates a critical semantic pathway that can more efficiently compress token vectors into global semantics with reduced order of complexity. Such compressed global semantics then serve as useful prior information in learning finer local pixel level details, through another constructed pixel pathway. The semantic pathway and pixel pathway are integrated together and are jointly trained, spreading the enhanced self-attention information in parallel through both of the pathways. Dual-ViT is henceforth able to capitalize on global semantics to boost self-attention learning without compromising much computational complexity. We empirically demonstrate that Dual-ViT provides superior accuracy than SOTA Transformer architectures with comparable training complexity.
更多查看译文
关键词
dual,vision
AI 理解论文
溯源树
样例
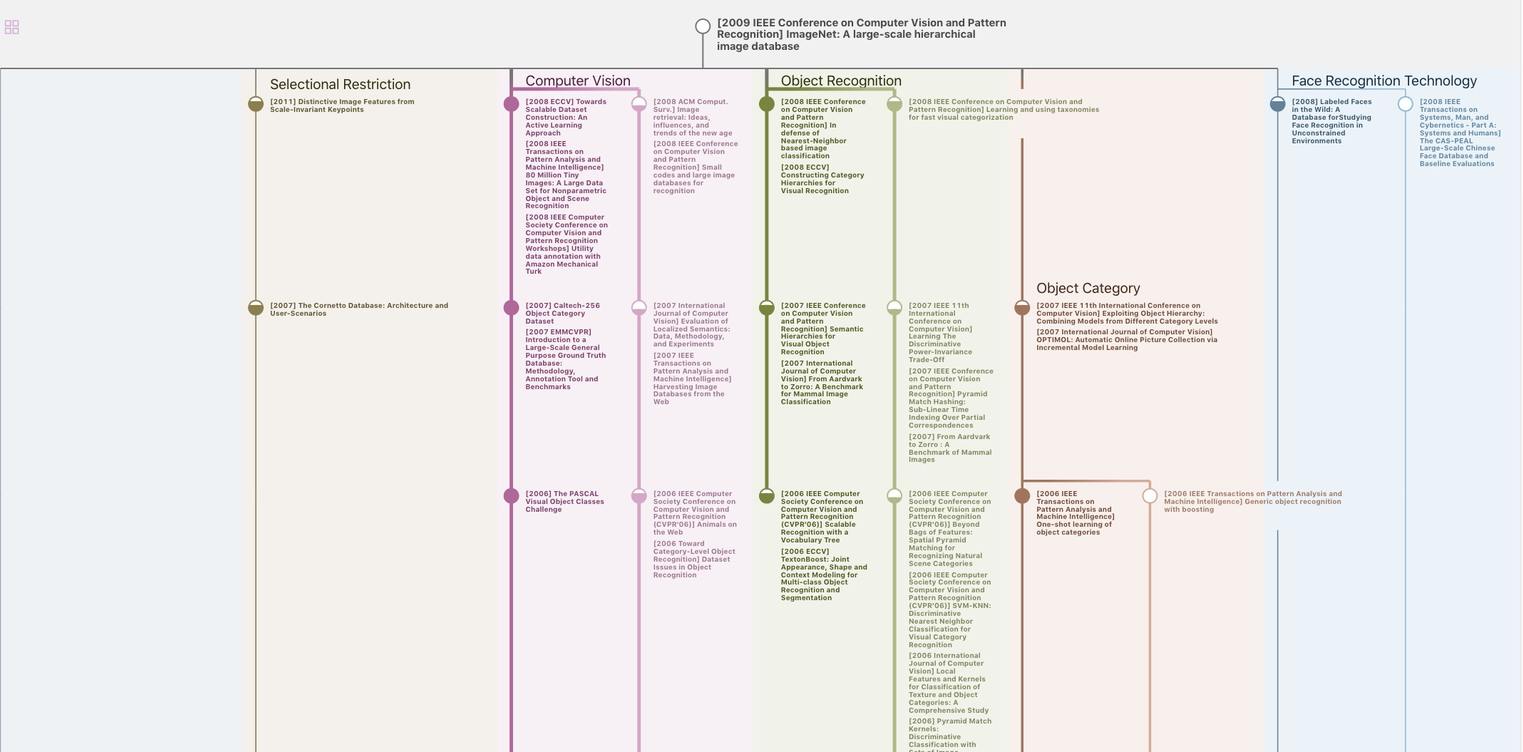
生成溯源树,研究论文发展脉络
Chat Paper
正在生成论文摘要