Differentiating Metropolis-Hastings to Optimize Intractable Densities
arXiv (Cornell University)(2023)
摘要
We develop an algorithm for automatic differentiation of Metropolis-Hastings samplers, allowing us to differentiate through probabilistic inference, even if the model has discrete components within it. Our approach fuses recent advances in stochastic automatic differentiation with traditional Markov chain coupling schemes, providing an unbiased and low-variance gradient estimator. This allows us to apply gradient-based optimization to objectives expressed as expectations over intractable target densities. We demonstrate our approach by finding an ambiguous observation in a Gaussian mixture model and by maximizing the specific heat in an Ising model.
更多查看译文
关键词
metropolis-hastings
AI 理解论文
溯源树
样例
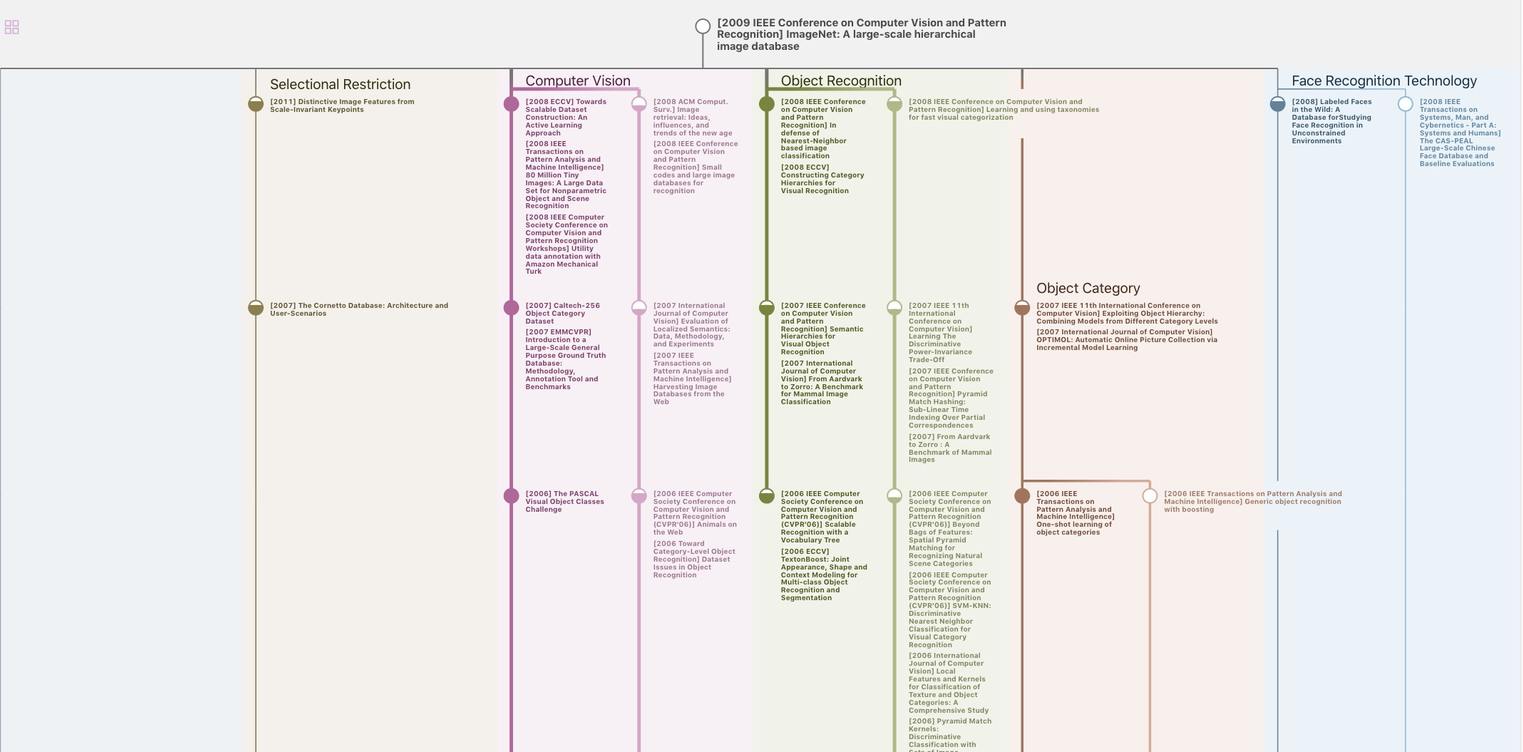
生成溯源树,研究论文发展脉络
Chat Paper
正在生成论文摘要