LESS: Label-efficient multi-scale learning for cytological whole slide image screening
MEDICAL IMAGE ANALYSIS(2024)
摘要
In computational pathology, multiple instance learning (MIL) is widely used to circumvent the computational impasse in giga-pixel whole slide image (WSI) analysis. It usually consists of two stages: patch -level feature extraction and slide -level aggregation. Recently, pretrained models or self -supervised learning have been used to extract patch features, but they suffer from low effectiveness or inefficiency due to overlooking the task -specific supervision provided by slide labels. Here we propose a weakly -supervised Label -Efficient WSI Screening method, dubbed LESS, for cytological WSI analysis with only slide -level labels, which can be effectively applied to small datasets. First, we suggest using variational positive -unlabeled (VPU) learning to uncover hidden labels of both benign and malignant patches. We provide appropriate supervision by using slide -level labels to improve the learning of patch -level features. Next, we take into account the sparse and random arrangement of cells in cytological WSIs. To address this, we propose a strategy to crop patches at multiple scales and utilize a cross -attention vision transformer (CrossViT) to combine information from different scales for WSI classification. The combination of our two steps achieves task -alignment, improving effectiveness and efficiency. We validate the proposed label -efficient method on a urine cytology WSI dataset encompassing 130 samples (13,000 patches) and a breast cytology dataset FNAC 2019 with 212 samples (21,200 patches). The experiment shows that the proposed LESS reaches 84.79%, 85.43%, 91.79% and 78.30% on the urine cytology WSI dataset, and 96.88%, 96.86%, 98.95%, 97.06% on the breast cytology high -resolution -image dataset in terms of accuracy, AUC, sensitivity and specificity. It outperforms state-of-the-art MIL methods on pathology WSIs and realizes automatic cytological WSI cancer screening.
更多查看译文
关键词
Computational pathology,Whole slide image,Multiple instance learning
AI 理解论文
溯源树
样例
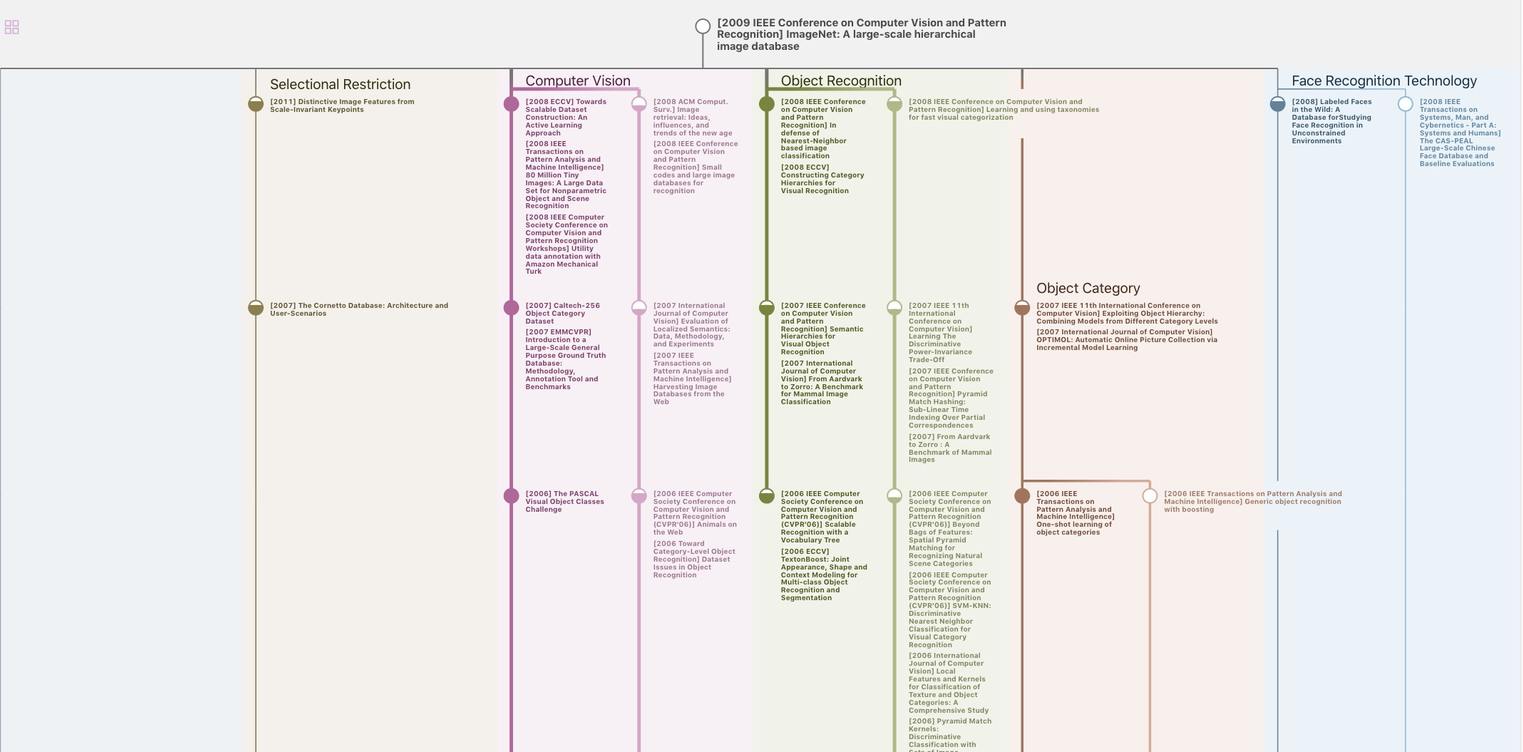
生成溯源树,研究论文发展脉络
Chat Paper
正在生成论文摘要