Intensity Filters
Studies in big data(2023)
摘要
This chapter deals with problems in which the number of targets is a time-varying random process to be estimated. The estimate is in terms of a target intensity function that specifies the expected number of targets per unit state space. When this function is integrated over a subset of the state space, one obtains the expected number of targets in that subset. In Chap. 4 , the goal was to estimate a Bayesian probability distribution on the multiple target state. In this chapter the goal shifts to developing a Bayesian estimate of the target intensity function. To bridge this viewpoint shift, we introduce finite point processes as a new model of multiple target state. We use Poisson point processes as our model for multitarget intensity functions and present a number of their useful properties. The chapter provides a straight-forward Bayesian proof of the Intensity Filter (iFilter) recursion, which is a simple recursion that computes (approximately) the multitarget intensity function. The chapter concludes with an example that applies the iFilter recursion to “track” four targets which appear and disappear at different times during the period considered by the example.
更多查看译文
关键词
intensity,filters
AI 理解论文
溯源树
样例
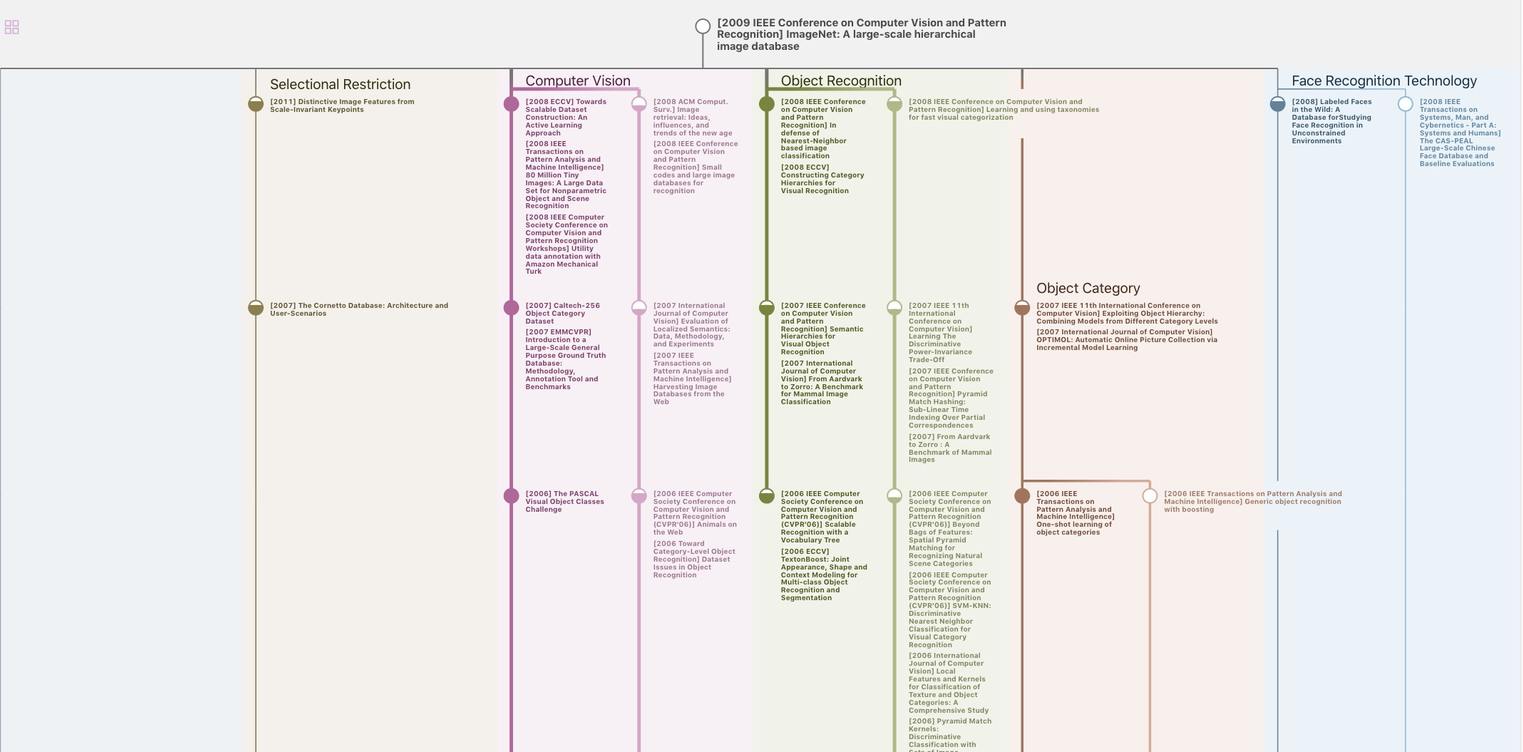
生成溯源树,研究论文发展脉络
Chat Paper
正在生成论文摘要