PEARL: Preprocessing Enhanced Adversarial Robust Learning of Image Deraining for Semantic Segmentation
arXiv (Cornell University)(2023)
摘要
In light of the significant progress made in the development and application of semantic segmentation tasks, there has been increasing attention towards improving the robustness of segmentation models against natural degradation factors (e.g., rain streaks) or artificially attack factors (e.g., adversarial attack). Whereas, most existing methods are designed to address a single degradation factor and are tailored to specific application scenarios. In this work, we present the first attempt to improve the robustness of semantic segmentation tasks by simultaneously handling different types of degradation factors. Specifically, we introduce the Preprocessing Enhanced Adversarial Robust Learning (PEARL) framework based on the analysis of our proposed Naive Adversarial Training (NAT) framework. Our approach effectively handles both rain streaks and adversarial perturbation by transferring the robustness of the segmentation model to the image derain model. Furthermore, as opposed to the commonly used Negative Adversarial Attack (NAA), we design the Auxiliary Mirror Attack (AMA) to introduce positive information prior to the training of the PEARL framework, which improves defense capability and segmentation performance. Our extensive experiments and ablation studies based on different derain methods and segmentation models have demonstrated the significant performance improvement of PEARL with AMA in defense against various adversarial attacks and rain streaks while maintaining high generalization performance across different datasets.
更多查看译文
关键词
adversarial robust learning,semantic segmentation,deraining
AI 理解论文
溯源树
样例
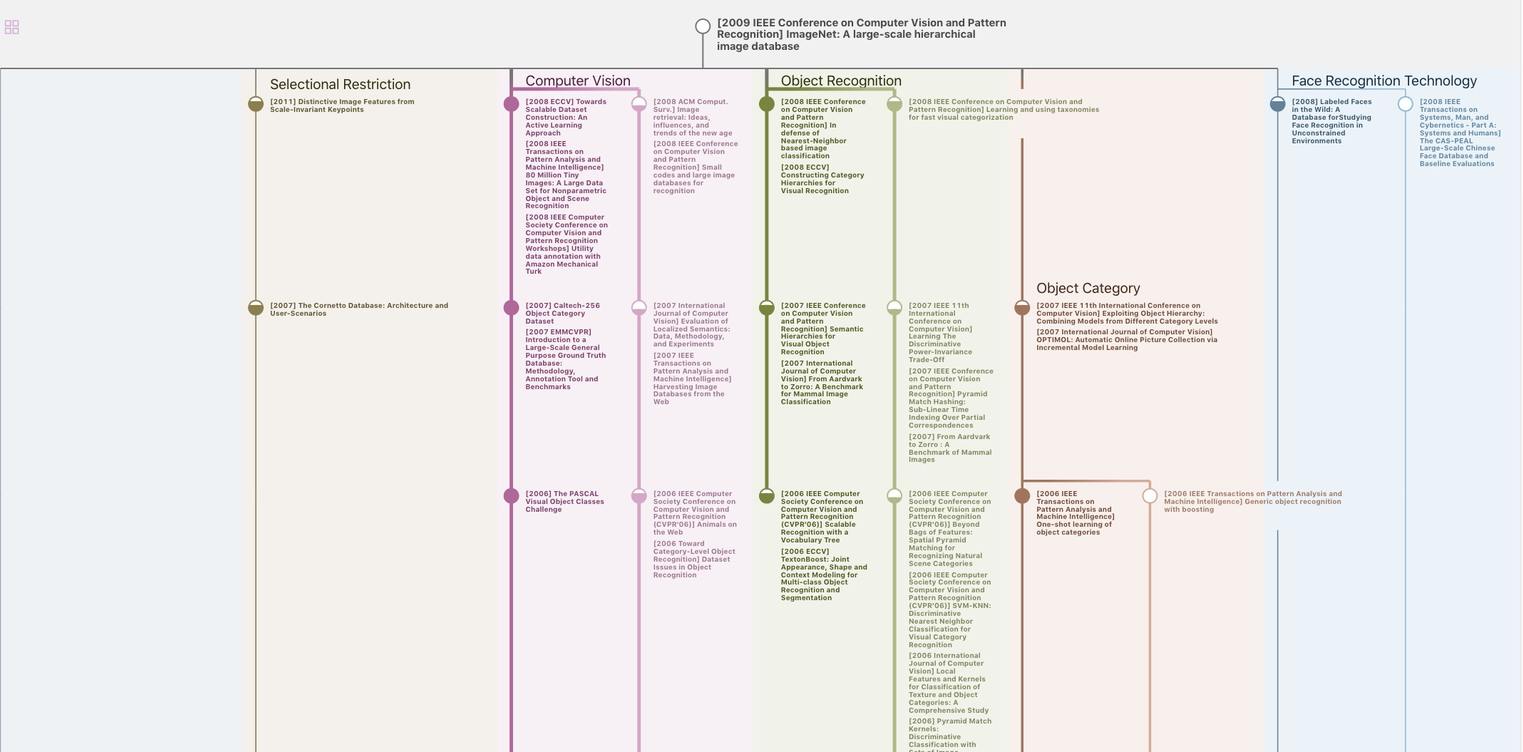
生成溯源树,研究论文发展脉络
Chat Paper
正在生成论文摘要