Who is Speaking Actually? Robust and Versatile Speaker Traceability for Voice Conversion
arXiv (Cornell University)(2023)
摘要
Voice conversion (VC), as a voice style transfer technology, is becoming increasingly prevalent while raising serious concerns about its illegal use. Proactively tracing the origins of VC-generated speeches, i.e., speaker traceability, can prevent the misuse of VC, but unfortunately has not been extensively studied. In this paper, we are the first to investigate the speaker traceability for VC and propose a traceable VC framework named VoxTracer. Our VoxTracer is similar to but beyond the paradigm of audio watermarking. We first use unique speaker embedding to represent speaker identity. Then we design a VAE-Glow structure, in which the hiding process imperceptibly integrates the source speaker identity into the VC, and the tracing process accurately recovers the source speaker identity and even the source speech in spite of severe speech quality degradation. To address the speech mismatch between the hiding and tracing processes affected by different distortions, we also adopt an asynchronous training strategy to optimize the VAE-Glow models. The VoxTracer is versatile enough to be applied to arbitrary VC methods and popular audio coding standards. Extensive experiments demonstrate that the VoxTracer achieves not only high imperceptibility in hiding, but also nearly 100% tracing accuracy against various types of audio lossy compressions (AAC, MP3, Opus and SILK) with a broad range of bitrates (16 kbps - 128 kbps) even in a very short time duration (0.74s). Our speech demo is available at https://anonymous.4open.science/w/DEMOofVoxTracer.
更多查看译文
关键词
versatile speaker traceability,conversion
AI 理解论文
溯源树
样例
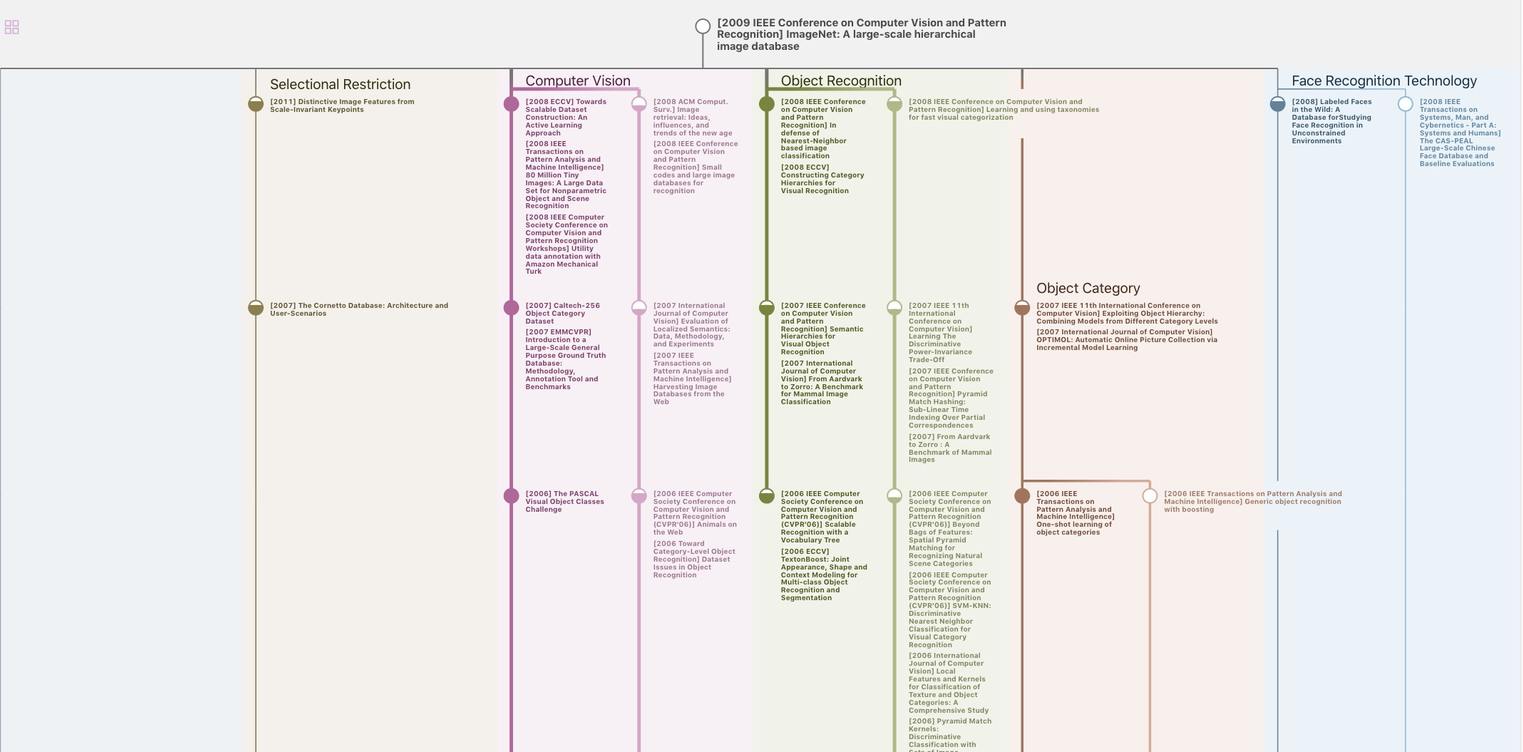
生成溯源树,研究论文发展脉络
Chat Paper
正在生成论文摘要