FairShap: A Data Re-weighting Approach for Algorithmic Fairness based on Shapley Values
arXiv (Cornell University)(2023)
摘要
Algorithmic fairness is of utmost societal importance, yet the current trend in large-scale machine learning models requires training with massive datasets that are typically biased. In this context, pre-processing methods that focus on modeling and correcting bias in the data emerge as valuable approaches. In this paper, we propose FairShap, a novel pre-processing (re-weighting) method for fair algorithmic decision-making through data valuation by means of Shapley Values. Our approach is model agnostic and easily interpretable, as it measures the contribution of each training data point to a predefined fairness metric. We empirically validate FairShap on several state-of-the-art datasets of different nature, with a variety of training scenarios and models and show how it outperforms other methods, yielding fairer models with higher or similar levels of accuracy. We also illustrate FairShap's interpretability by means of histograms and latent space visualizations. We believe that this work represents a promising direction in interpretable and model-agnostic approaches to algorithmic fairness that yield competitive accuracy even when only biased datasets are available.
更多查看译文
关键词
algorithmic fairness,fairshap,re-weighting
AI 理解论文
溯源树
样例
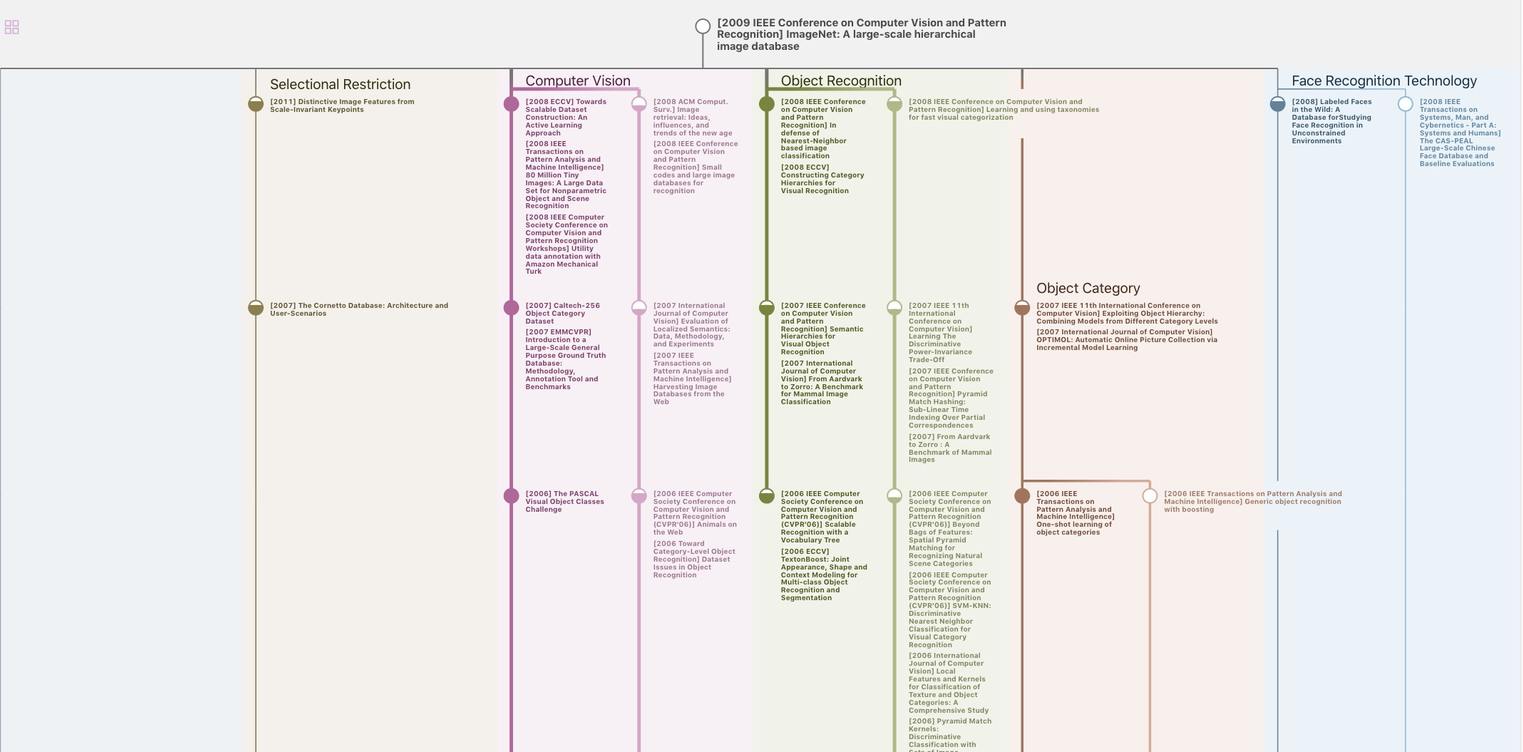
生成溯源树,研究论文发展脉络
Chat Paper
正在生成论文摘要