Dissecting cell state transitions by causal inference
bioRxiv (Cold Spring Harbor Laboratory)(2023)
摘要
Abstract Trajectory inference methods are frequently used for cell fate analysis, however, most of them are similarity-based and lack an understanding of the causality underlying differentiation processes. Here, we present CIBER, a C ausal I nference– B ased framework for the E valuation of feature effects and the R econstruction of cellular differentiation networks. CIBER provides a novel paradigm for dissecting cell state transitions other than trajectory inference and differential analysis. It is a versatile framework that can be applied to various types of data, including transcriptomic, epigenomic and microarray data. It can identify both known and potential cell-lineage structures with minimal prior knowledge. By integrating the CIBER-learned network with structural causal model and applying in silico perturbation as inventions, we generated an effect matrix that quantifies the impact of different features on each differentiation branch. Using this effect matrix, CIBER can identify crucial features involved in haematopoiesis, even if these features show no significant difference in expression between lineages. Moreover, CIBER can predict novel regulation associations and provide insight into the potential mechanism underlying the influence of transcription factors on biological processes. To validate CIBER’s capabilities, we conducted in vivo experiments on Bcl11b , a non-differentially expressed transcription factor identified by CIBER. Our results demonstrate that dysfunction of Bcl11b leads to a bias towards myeloid lineage differentiation at the expense of lymphoid lineage, which is consistent with our predictions.
更多查看译文
关键词
cell state transitions,causal inference
AI 理解论文
溯源树
样例
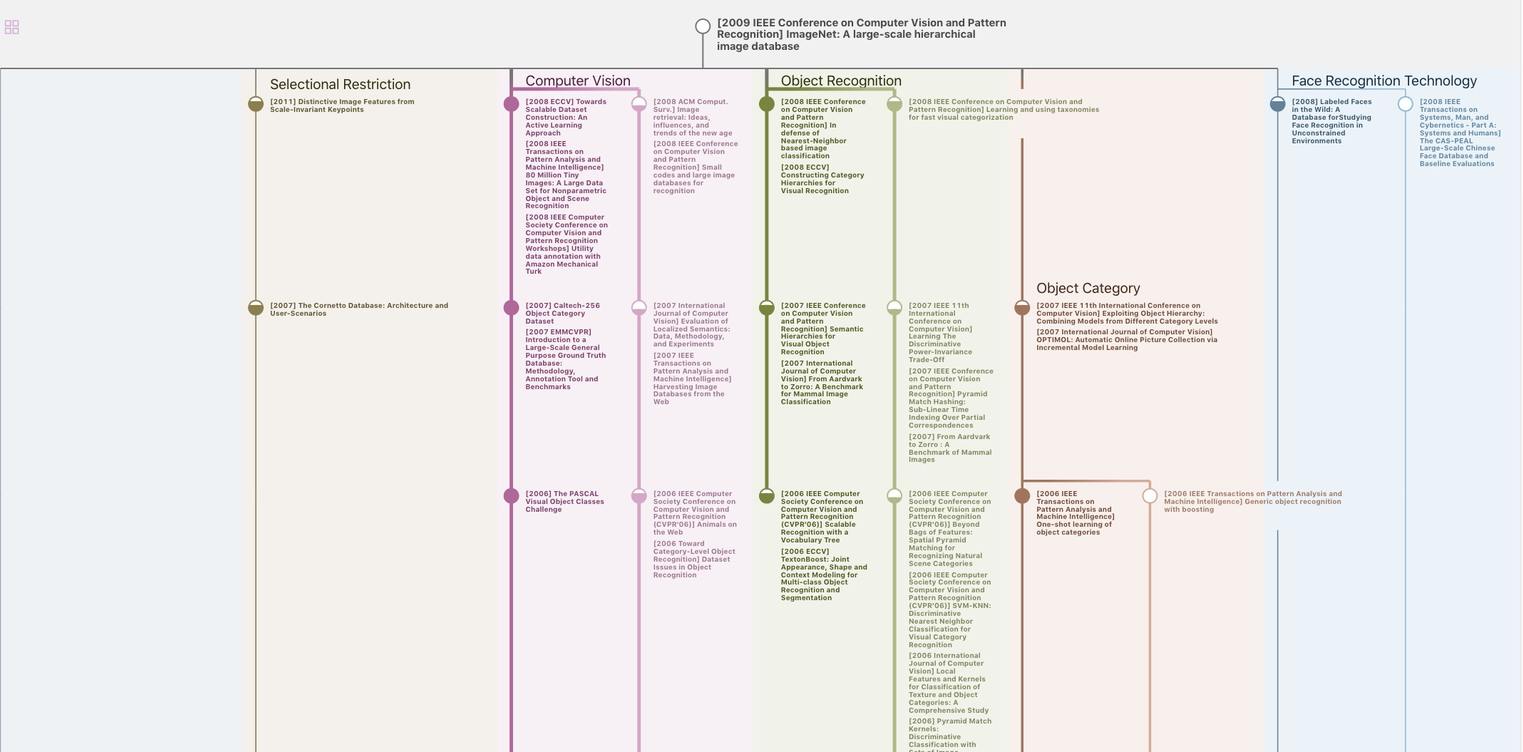
生成溯源树,研究论文发展脉络
Chat Paper
正在生成论文摘要