Priors are Powerful: Improving a Transformer for Multi-camera 3D Detection with 2D Priors
arXiv (Cornell University)(2023)
摘要
Transfomer-based approaches advance the recent development of multi-camera 3D detection both in academia and industry. In a vanilla transformer architecture, queries are randomly initialised and optimised for the whole dataset, without considering the differences among input frames. In this work, we propose to leverage the predictions from an image backbone, which is often highly optimised for 2D tasks, as priors to the transformer part of a 3D detection network. The method works by (1). augmenting image feature maps with 2D priors, (2). sampling query locations via ray-casting along 2D box centroids, as well as (3). initialising query features with object-level image features. Experimental results shows that 2D priors not only help the model converge faster, but also largely improve the baseline approach by up to 12% in terms of average precision.
更多查看译文
关键词
priors,2d,detection,transformer,multi-camera
AI 理解论文
溯源树
样例
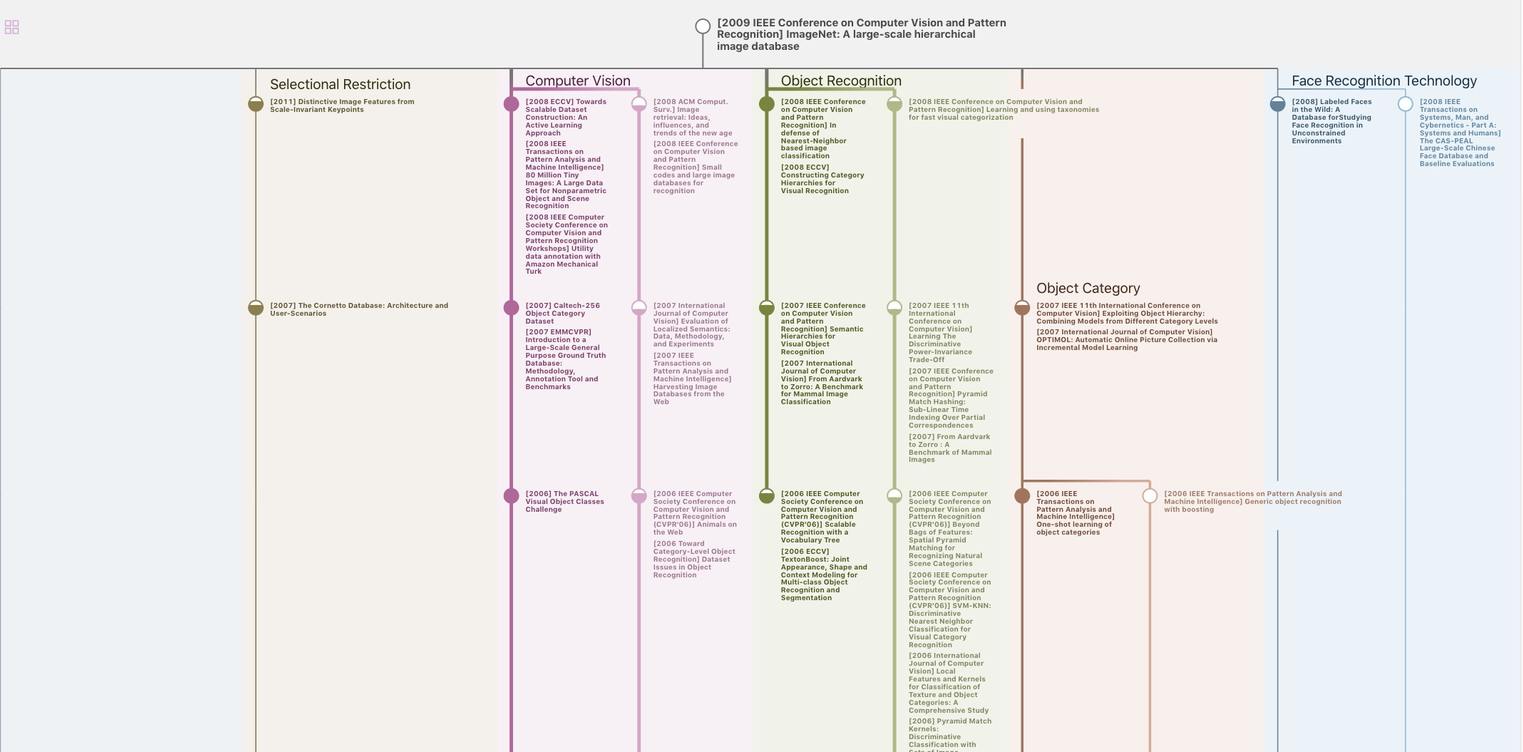
生成溯源树,研究论文发展脉络
Chat Paper
正在生成论文摘要