Post-hoc Explanation for Twitter List Recommendation
Research Square (Research Square)(2023)
摘要
Abstract Twitter List recommender systems have the ability to generate accurate recommendations, but since they utilize heterogeneous user and List information on Twitter and usually apply complex hybrid prediction models, they cannot provide user-friendly intrinsic explanations. In this paper, we propose an explanation model to provide post-hoc explanations for recommended Twitter Lists based on the user’s own actions; and consequently benefits to improve recommendation acceptance by end users. The proposed model includes two main components: (1) candidate explanation generation in which the most semantically related actions of a user on Twitter to the recommended List are retrieved as candidate explanations; and (2) explanation ranking to re-rank candidates based on relatedness to the List and their informativeness. Through experiments on a real-world Twitter dataset, we demonstrate that the proposed explanation model can effectively generate related, informative and useful post-hoc explanations for the recommended Lists to users, while maintaining parity in recommendation performance.
更多查看译文
关键词
twitter list recommendation,post-hoc
AI 理解论文
溯源树
样例
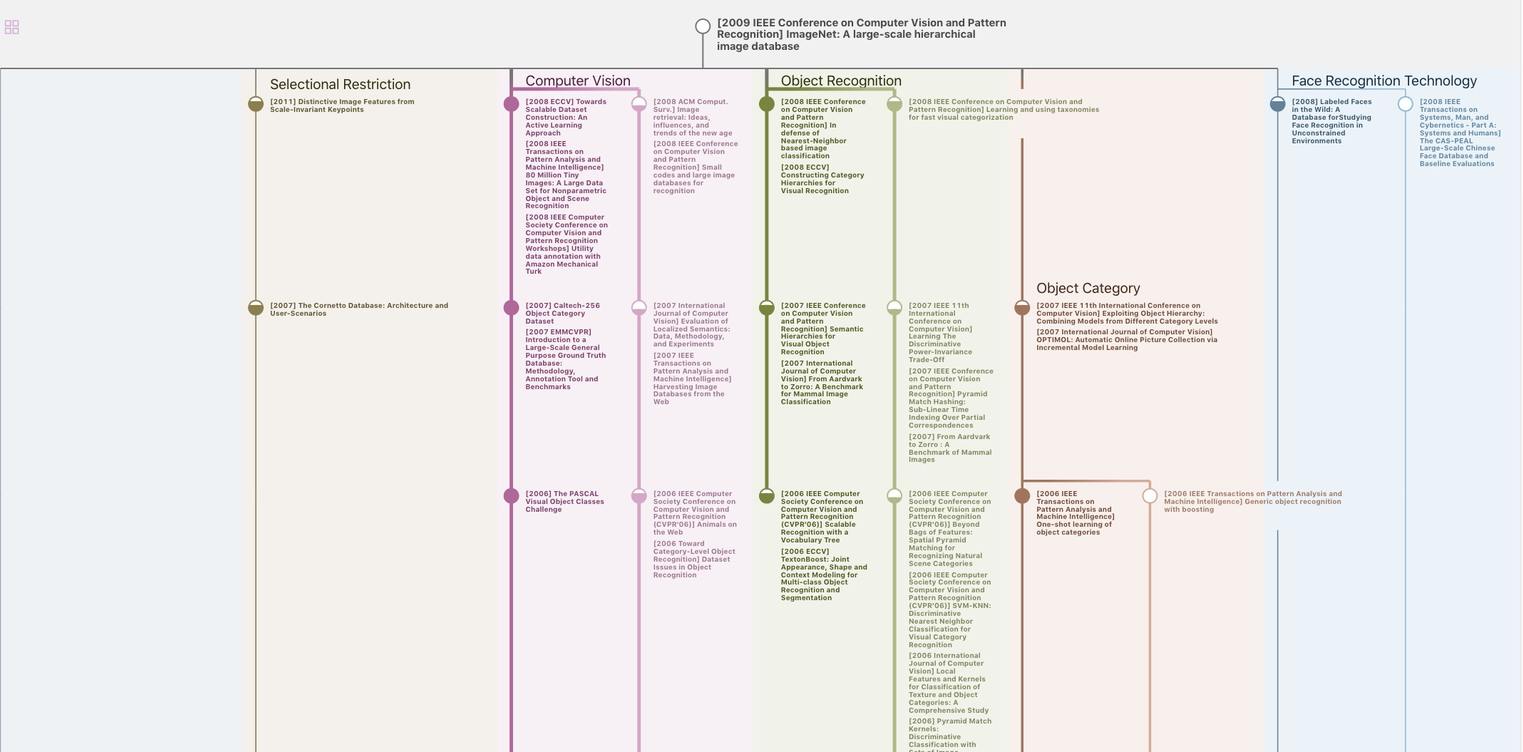
生成溯源树,研究论文发展脉络
Chat Paper
正在生成论文摘要