Deep learning prediction of enzyme optimum pH
bioRxiv (Cold Spring Harbor Laboratory)(2023)
摘要
Abstract The relationship between pH and enzyme catalytic activity, as well as the optimal pH (pH opt ) at which enzymes function, is crucial for biotechnological applications. Consequently, computational methods that predict pH opt would significantly benefit enzyme discovery and design by facilitating accurate identification of enzymes that function optimally at a specific pH, and by promoting a better understanding of how sequence affects enzyme function in relation to pH. In this study, we present EpHod (Enzyme pH optimum prediction with deep learning), which is a deep semi-supervised language model for predicting enzyme pH opt directly from the protein sequence. By evaluating various machine learning methods with extensive hyperparameter optimization (training over 4,000 models in total), we find that semi-supervised methods that utilize language model embeddings, including EpHod, achieve the lowest error in predicting pH opt . From sequence data alone, EpHod learns structural and biophysical features that relate to pH opt , including proximity of residues to the catalytic center and the accessibility of solvent molecules. Overall, EpHod presents a promising advancement in pH opt prediction and could potentially speed up the development of improved enzyme technologies.
更多查看译文
关键词
deep learning,enzyme,ph,prediction
AI 理解论文
溯源树
样例
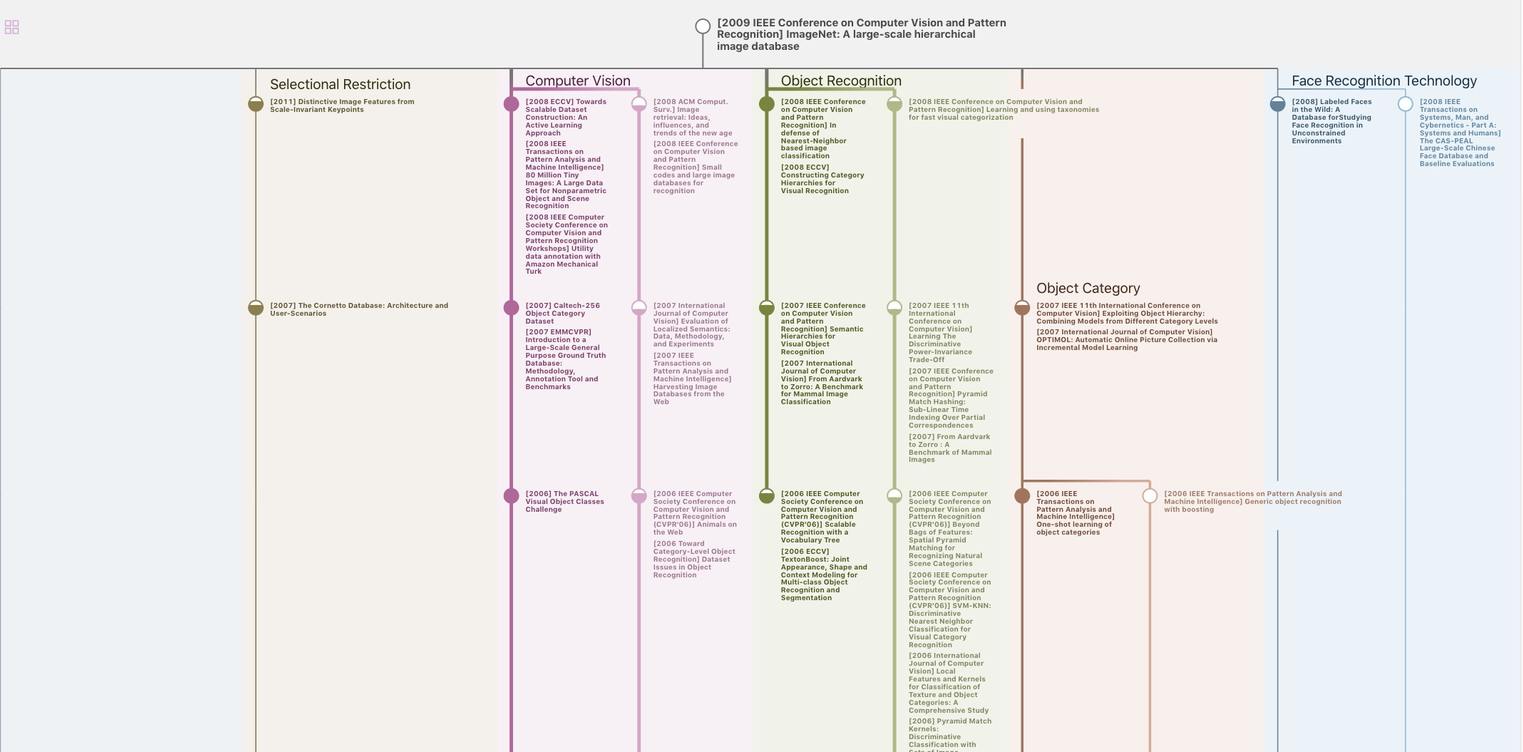
生成溯源树,研究论文发展脉络
Chat Paper
正在生成论文摘要