Masked Autoencoders with Multi-Window Local-Global Attention Are Better Audio Learners
arXiv (Cornell University)(2023)
摘要
In this work, we propose a Multi-Window Masked Autoencoder (MW-MAE) fitted with a novel Multi-Window Multi-Head Attention (MW-MHA) module that facilitates the modelling of local-global interactions in every decoder transformer block through attention heads of several distinct local and global windows. Empirical results on ten downstream audio tasks show that MW-MAEs consistently outperform standard MAEs in overall performance and learn better general-purpose audio representations, along with demonstrating considerably better scaling characteristics. Investigating attention distances and entropies reveals that MW-MAE encoders learn heads with broader local and global attention. Analyzing attention head feature representations through Projection Weighted Canonical Correlation Analysis (PWCCA) shows that attention heads with the same window sizes across the decoder layers of the MW-MAE learn correlated feature representations which enables each block to independently capture local and global information, leading to a decoupled decoder feature hierarchy. Code for feature extraction and downstream experiments along with pre-trained models will be released publically.
更多查看译文
关键词
audio learners,masked autoencoders,attention,multi-window,local-global
AI 理解论文
溯源树
样例
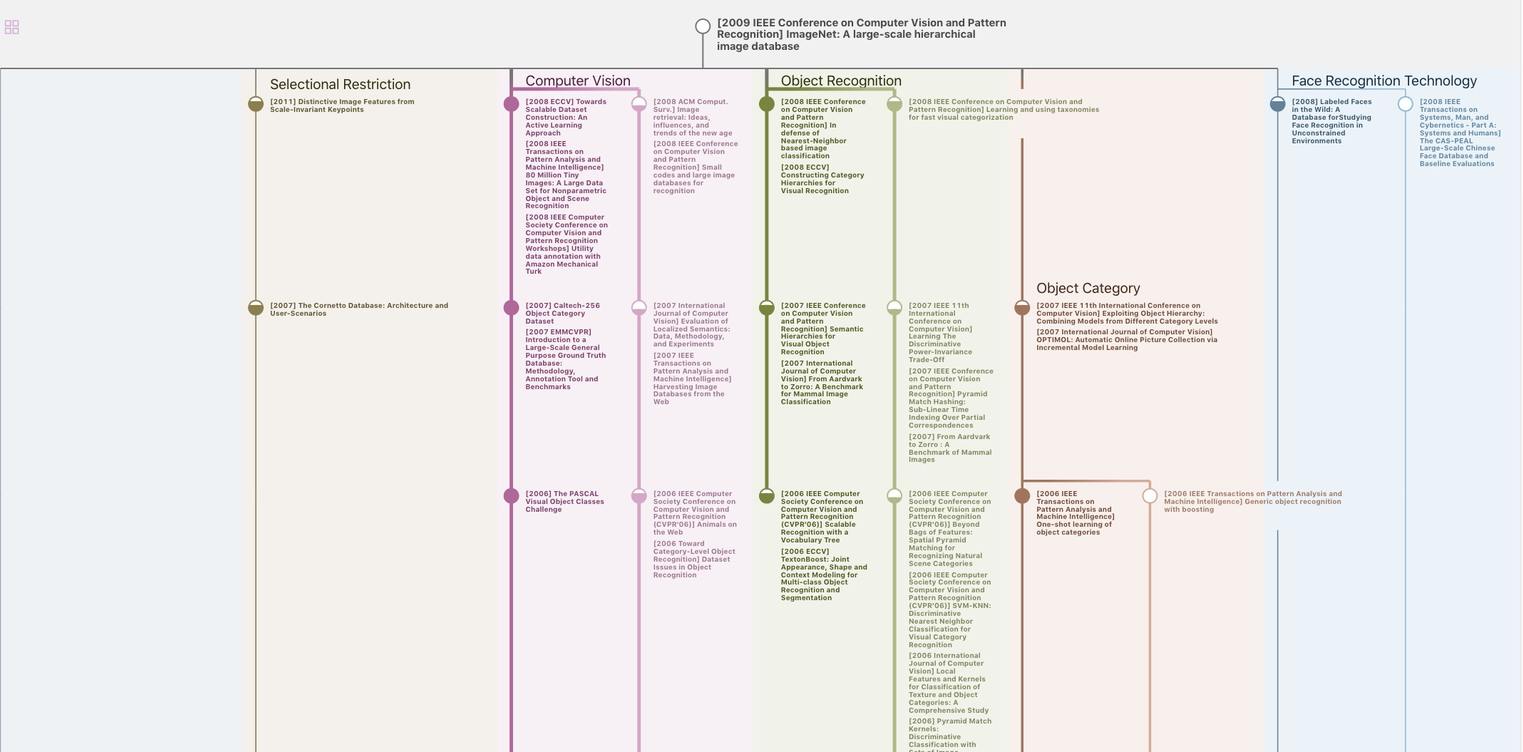
生成溯源树,研究论文发展脉络
Chat Paper
正在生成论文摘要