Sharpness and well-conditioning of nonsmooth convex formulations in statistical signal recovery
arXiv (Cornell University)(2023)
摘要
We study a sample complexity vs. conditioning tradeoff in modern signal recovery problems where convex optimization problems are built from sampled observations. We begin by introducing a set of condition numbers related to sharpness in $\ell_p$ or Schatten-p norms ($p\in[1,2]$) based on nonsmooth reformulations of a class of convex optimization problems, including sparse recovery, low-rank matrix sensing, covariance estimation, and (abstract) phase retrieval. In each of the recovery tasks, we show that the condition numbers become dimension independent constants once the sample size exceeds some constant multiple of the recovery threshold. Structurally, this result ensures that the inaccuracy in the recovered signal due to both observation noise and optimization error is well-controlled. Algorithmically, such a result ensures that a new first-order method for solving the class of sharp convex functions in a given $\ell_p$ or Schatten-p norm, when applied to the nonsmooth formulations, achieves nearly-dimension-independent linear convergence.
更多查看译文
关键词
statistical signal recovery,nonsmooth convex formulations,well-conditioning
AI 理解论文
溯源树
样例
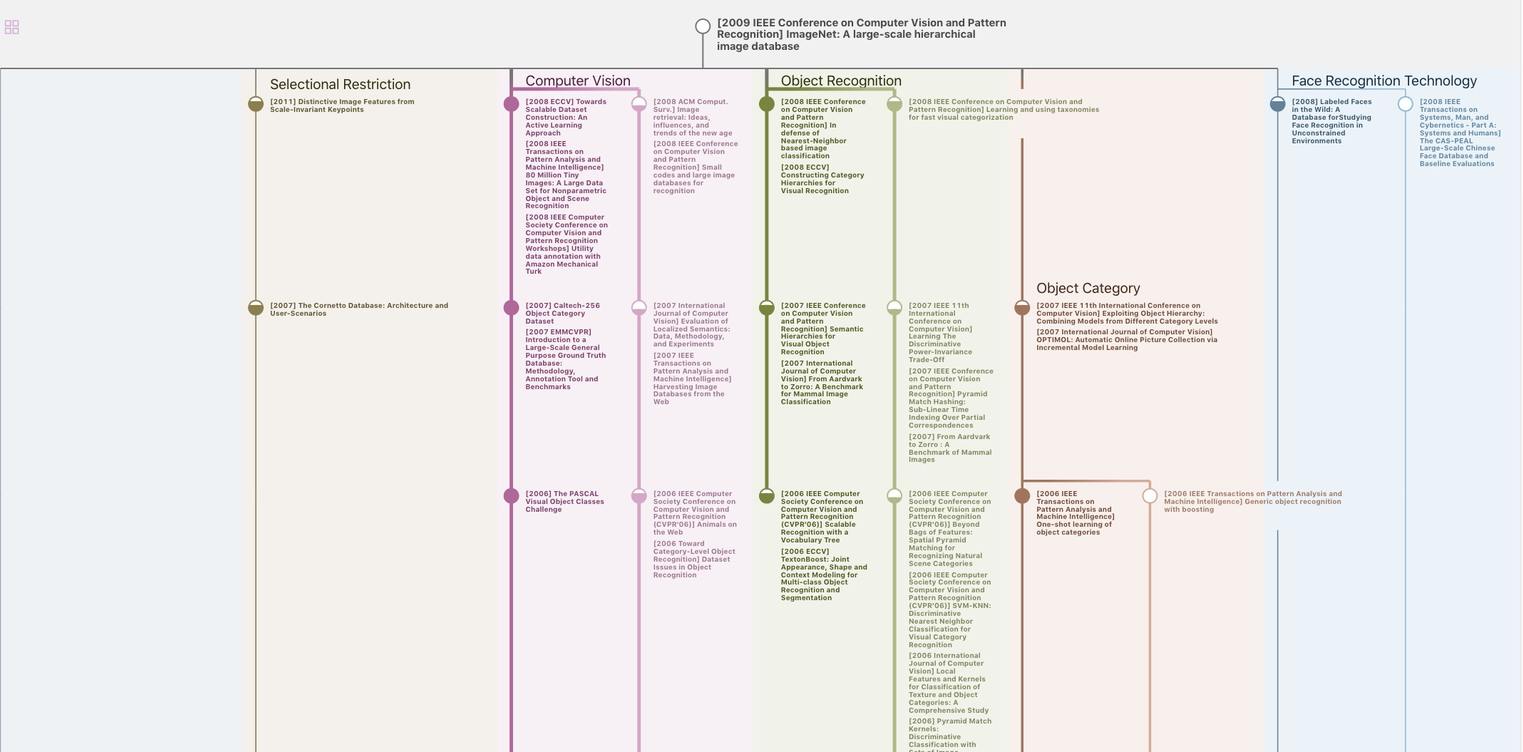
生成溯源树,研究论文发展脉络
Chat Paper
正在生成论文摘要