Computerized Adaptive Testing Without IRT for Flexible Measurement and Prediction
Methodology of educational measurement and assessment(2023)
摘要
Abstract In education, testing procedures can be lengthy. The long duration takes up precious time and affects the quality of responses, possibly resulting in a biased diagnosis or wrong treatment. The problem can be reduced using computer adaptive testing (CAT). However, three issues prevent the use of traditional CAT: (1) the type of tests and questionnaires we focus on do not allow for the construction of large item banks, (2) the test data are usually not (approximately) unidimensional, and (3) the aim of the researchers may not only be measurement but also prediction. We propose a flexible generalization of CAT to accommodate these three issues, coined FlexCAT. First, FlexCAT estimates the (discrete) density of item-score vectors (denoted p ) using any convenient model that provides a good description of p ; this need not be an IRT model. Second, FlexCAT estimates test scores from $$ \hat{\mathbf{p}} $$ p ̂ . In contrast to traditional CAT, the test score need not be a latent trait but can also be the total score, ordinal scores such as percentiles, or external criteria that the test aims to predict. We introduce FlexCAT for the case that a latent class model is used to estimate p , and the total score is used as a test score. Using a real-data example, we compare the accuracy of FlexCAT and traditional CAT. Finally, we discuss the challenges FlexCAT still faces.
更多查看译文
关键词
testing,flexible measurement,irt,prediction
AI 理解论文
溯源树
样例
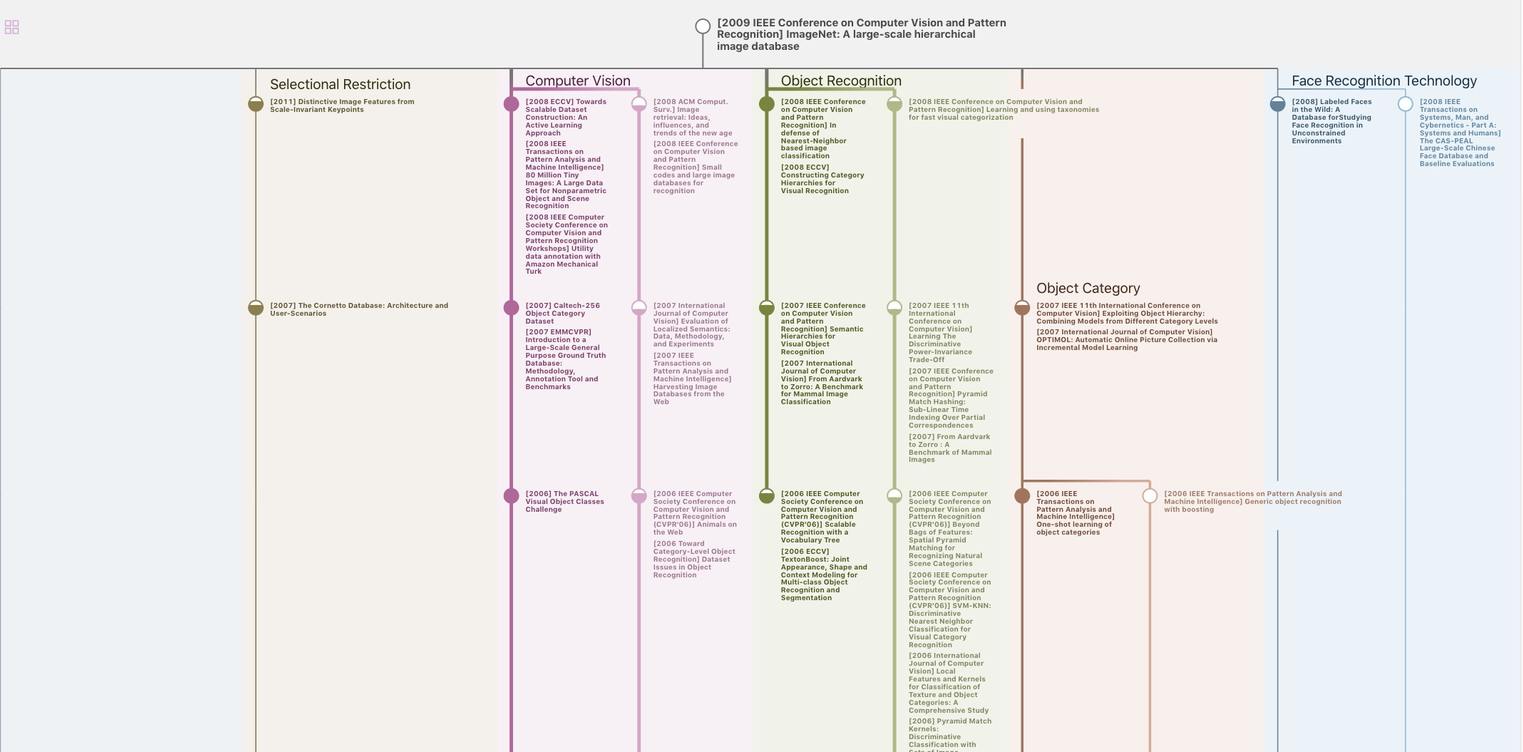
生成溯源树,研究论文发展脉络
Chat Paper
正在生成论文摘要