CoreScore: a machine learning approach to assess legacy core condition
Geological Society, London, Special Publications(2023)
摘要
Abstract Today's geoscience challenges often require repurposing of data and samples from legacy boreholes. Collection of new deep core is expensive; maximizing this investment is vital. However, the condition of legacy cores varies due to factors including recovery, sampling, lithology, and storage. Rock Quality Designation analysis is often undertaken on new core but this only provides a snapshot of core condition and will not be indicative of subsequent condition. Poor core condition can make destructive analytical techniques impossible and also impacts non-destructive techniques including core scanning. Since 2011, BGS have systematically collected 125 000 core images. This study investigates if core condition of this archive can be assessed using automated analysis by machine learning. A neural network-based approach was used to segment these images. By differentiating imaged core from their background, properties such as number of fragments and total rock area were determined and used to assess core condition. Analysis of outputs demonstrates that with minimal input data, core condition can be rapidly assessed. This allows users to better understand and visualize core. This can be used to qualitatively assess non-destructive data, improve success of destructive sampling through targeted sampling and reduce the time and effort spent interacting with physical material.
更多查看译文
关键词
corescore,machine learning,machine learning approach
AI 理解论文
溯源树
样例
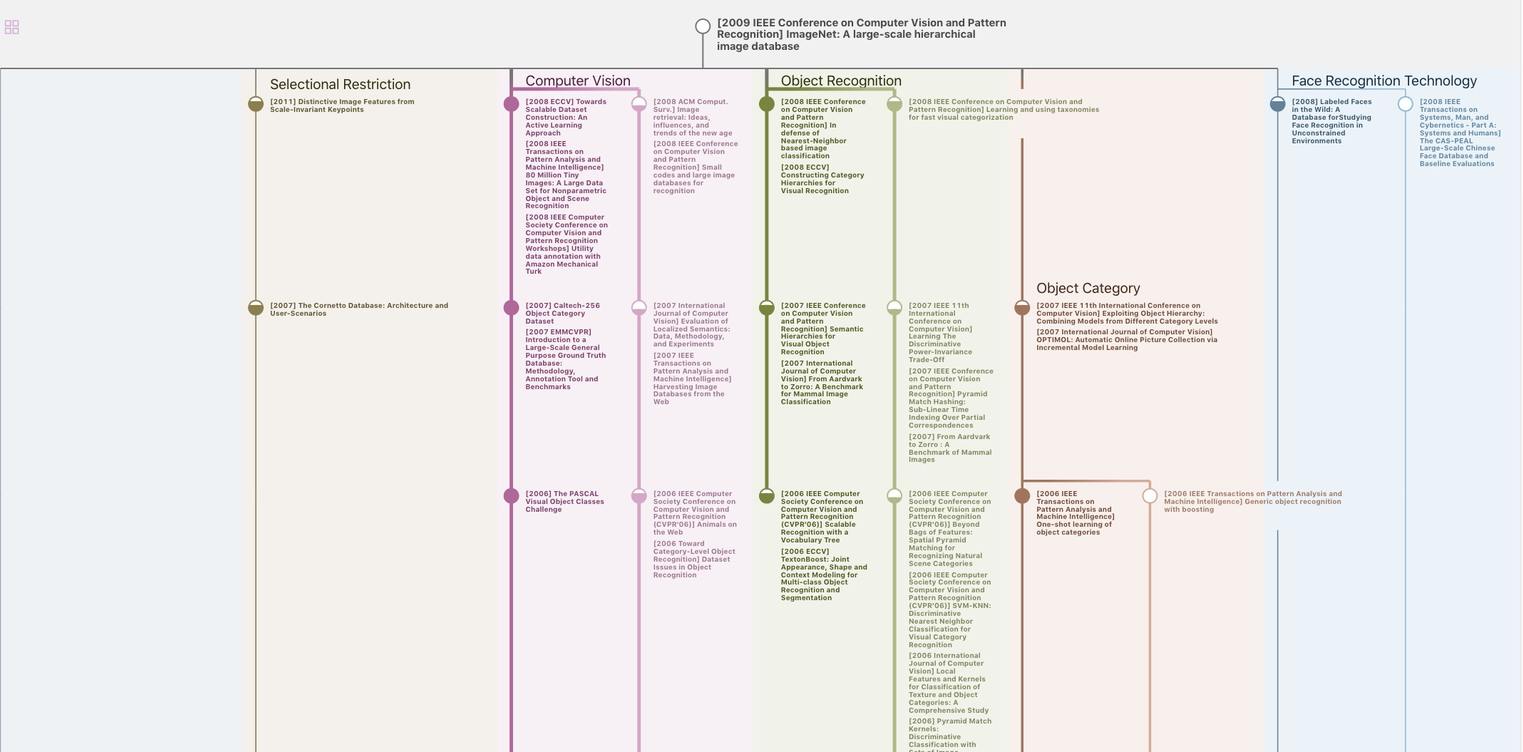
生成溯源树,研究论文发展脉络
Chat Paper
正在生成论文摘要