Fast characterization of biodiesel via a combination of ATR-FTIR and machine learning models
Fuel(2023)
摘要
This study proposed a fast characterization method for biodiesel based on attenuated total reflection flourier-transform infrared spectroscopy and machine learning models. The concerning characteristics of biodiesel include unsaturated group content, O content, and contents of four representative esters. A total of 71 biodiesel samples were produced from a lab-scale reactor. Their spectral data and characteristics were collected and used as training data for machine learning models. The established model framework consisted of two data compression sections, a classification section, and a regression section, all of which use machine learning models, such as principal component analysis, support vector machine, artificial neural network, and random forest. The accuracy, correlation, and sensitivity of the proposed method were evaluated and optimized. Furthermore, the interpretation of the models was discussed. The results showed that the principal component analysis model was a satisfactory preprocessing procedure for the downstream classification and regression models. Under the optimal model parameters, the integrated framework could reach an average accuracy rate of 93.18% and a Pearson correlation coefficient of 0.92. Principal component number 4 and 5 showed the highest sensitivity towards the predicting results, implying that their highly weighted wavenumber ranges and the correlated functional groups played the most important role in the predicting process. The findings of this study could lead to a simple and efficient approach for characterizing the properties of biodiesel, which in turn could promote the development of similar biomass-derived liquid fuels.
更多查看译文
关键词
biodiesel,machine learning,fast characterization,atr-ftir
AI 理解论文
溯源树
样例
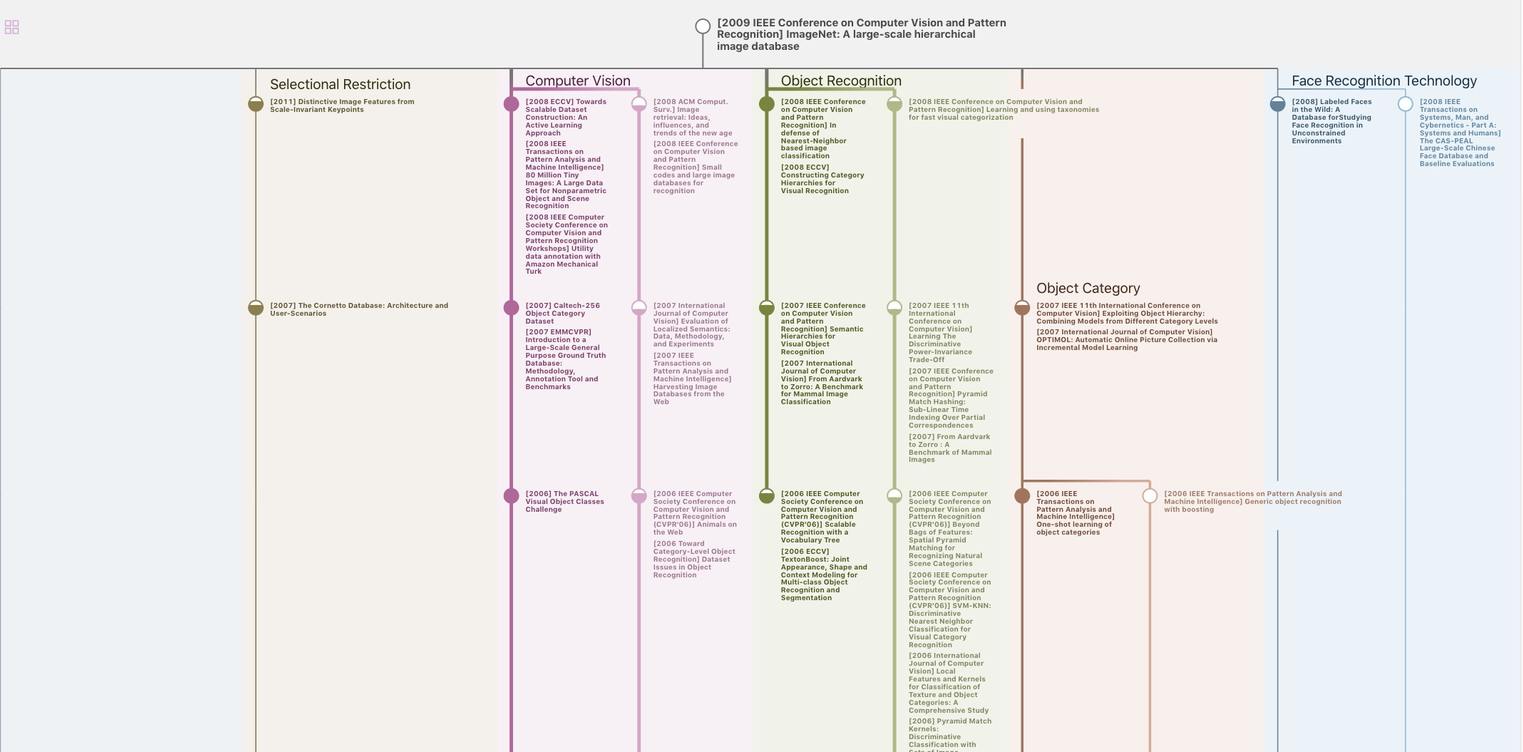
生成溯源树,研究论文发展脉络
Chat Paper
正在生成论文摘要