Estimating protein complex model accuracy based on ultrafast shape recognition and deep learning in CASP15
Authorea (Authorea)(2023)
摘要
This article reports and analyzes the results of protein complex model accuracy estimation by our methods (DeepUMQA3 and GraphGPSM) in the 15 Critical Assessment of techniques for protein Structure Prediction (CASP15). The new deep learning-based multimeric complex model accuracy estimation methods are proposed based on the ensemble of three level features coupling with deep residual/graph neural networks. For the input multimeric complex model, we describe it from three levels: overall complex features, intra-monomer features, and inter-monomer features. We designed an overall ultrafast shape recognition (USR) to characterize the relationship between local residues and the overall complex topology, and an inter-monomer USR to characterize the relationship between the residues of one monomer and the topology of other monomers. On the 39 complex targets of CASP15, DeepUMQA3 (Group name: GuijunLab-RocketX) ranked first in the assessment of interface residue accuracy. The Pearson correlation coefficient (PCC) between the interface residues lDDT predicted by DeepUMQA3 and the real lDDT is 0.570, and DeepUMQA3 achieved the highest PCC on 29 out of 39 targets. GraphGPSM (Group name: GuijunLab-PAthreader) had a TM-score PCC>0.9 on 14 targets, showing a good ability to estimate the overall fold accuracy.
更多查看译文
关键词
ultrafast shape recognition,complex model accuracy,deep learning,protein
AI 理解论文
溯源树
样例
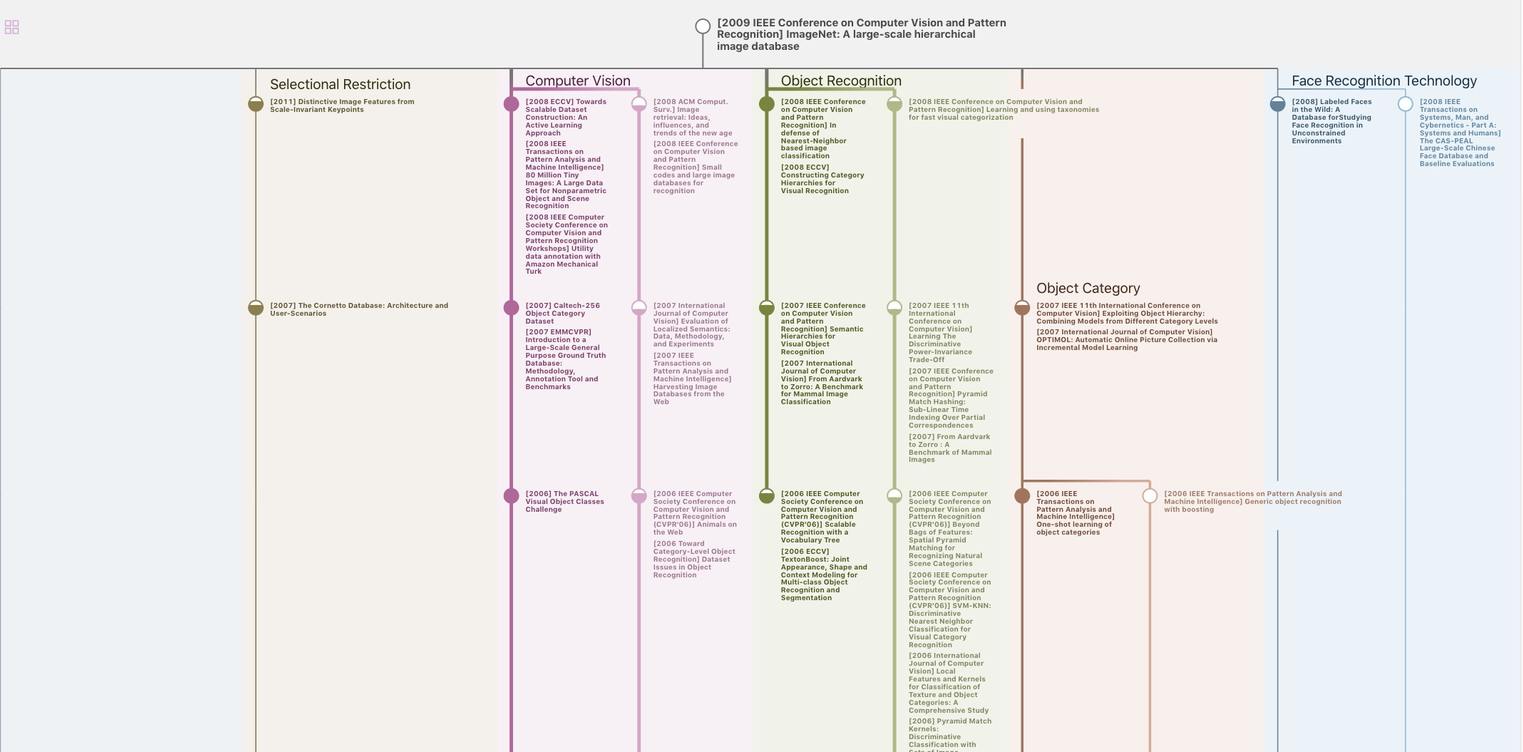
生成溯源树,研究论文发展脉络
Chat Paper
正在生成论文摘要