Prediction of late atrial fibrillation recurrence after catheter ablation using machine learning
European Heart Journal(2023)
摘要
Abstract Background Late recurrence of atrial fibrillation (LRAF) occurring 3 up to 12 months following catheter ablation is considered a relevant clinical problem (rate of occurrence estimated at 20%-45%). Hence, it is significant to define LRAF predictors which would allow for a more suitable selection of patients susceptible to AF recurrence and which could increase the effectiveness of the ablation procedure. Purpose The aim of the present study is to develop a machine-learning model of arrhythmic recurrence within one year from catheter ablation. Methods This study group comprised 201 patients with paroxysmal, persistent and long standing persistent AF who underwent pulmonary vein isolation performed by cryoballoon ablation and radiofrequency ablation using the CARTO-mapping (122 cryoablation and 79 radiofrequency) in a single center study between May 2016 and March 2018. The biomarker concentrations were measured at baseline and after ablation in a 24-h follow-up. First, five different supervised machine-learning models (decision tree, logistic regression, random forest, XGBoost, support vector machines) were developed for predicting AF recurrence within one year following catheter ablation. Next, SHapley Additive exPlanations (SHAP) were derived to explain the predictions using 82 parameters from clinical, laboratory and procedural variables collected from each patient. The models were trained and validated using stratified 5-fold cross-validation and a feature selection was performed with the permutation importance. Results During the 1-year follow-up LRAF occurred in 57 patients (28.3%). The respective receiver operating characteristic (ROC) curves for this model calculated for the Validation set are presented in Figure 1. It is observed that the XGBoost model with the 12 variables achieves the highest AUC of 0.75 (95% confidence interval: 0.7395, 0.7653). The high values of the top 2 features (early recurrence of atrial fibrillation (ERAF) and TSH) contribute to predicting LRAF most, while low values of the top 3 features (RBC, hs-Troponin T, HDL cholesterol) contribute positively to this class (Figure 2). Conclusion The machine learning model predicting the 1-year risk of atrial fibrillation recurrence after catheter ablation has achieved good predictive ability. The above model based on the easily available clinical and laboratory data will support the clinical decision-making for selecting good responders to the ablation procedure.
更多查看译文
关键词
late atrial fibrillation recurrence,atrial fibrillation,catheter ablation,machine learning
AI 理解论文
溯源树
样例
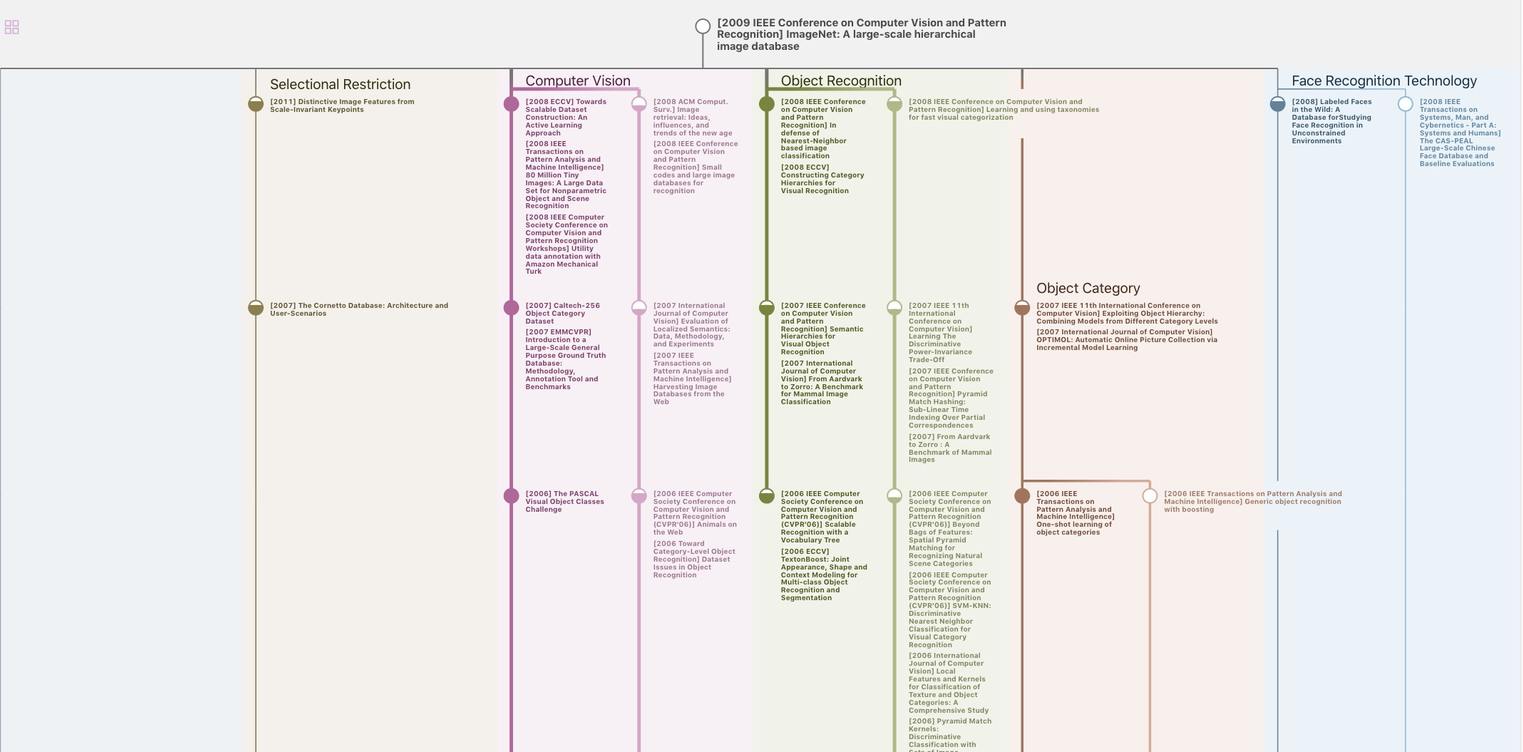
生成溯源树,研究论文发展脉络
Chat Paper
正在生成论文摘要