Learning Nonlinear Reduced Models from Data with Operator Inference
ANNUAL REVIEW OF FLUID MECHANICS(2024)
Abstract
This review discusses Operator Inference, a nonintrusive reduced modeling approach that incorporates physical governing equations by defining a structured polynomial form for the reduced model, and then learns the corresponding reduced operators from simulated training data. The polynomial model form of Operator Inference is sufficiently expressive to cover a wide range of nonlinear dynamics found in fluid mechanics and other fields of science and engineering, while still providing efficient reduced model computations. The learning steps of Operator Inference are rooted in classical projection-based model reduction; thus, some of the rich theory of model reduction can be applied to models learned with Operator Inference. This connection to projection-based model reduction theory offers a pathway toward deriving error estimates and gaining insights to improve predictions. Furthermore, through formulations of Operator Inference that preserve Hamiltonian and other structures, important physical properties such as energy conservation can be guaranteed in the predictions of the reduced model beyond the training horizon. This review illustrates key computational steps of Operator Inference through a large-scale combustion example.
MoreTranslated text
Key words
data-driven modeling,scientific machine learning,structure preservation,nonlinear model reduction,Operator Inference
AI Read Science
Must-Reading Tree
Example
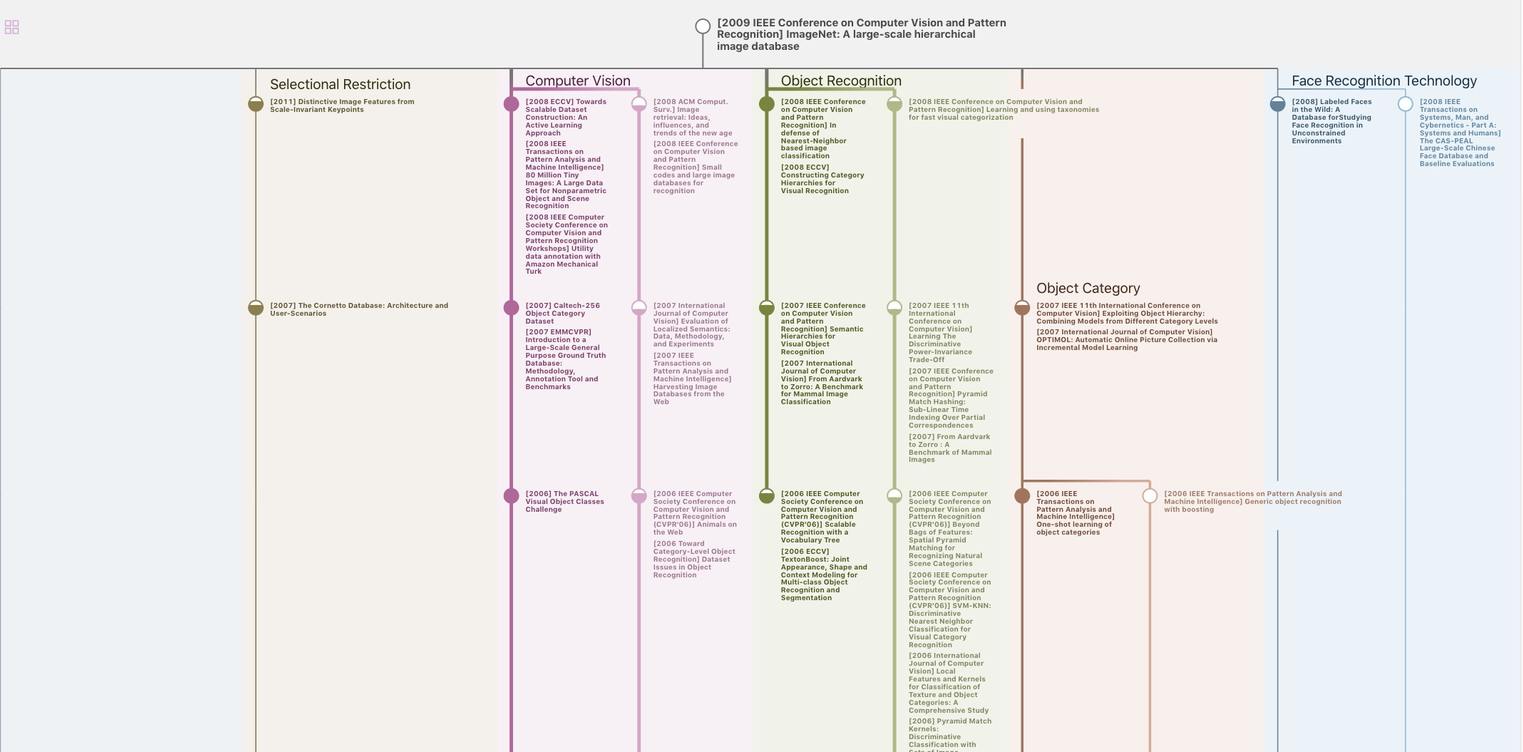
Generate MRT to find the research sequence of this paper
Chat Paper
Summary is being generated by the instructions you defined