Learning Semantics-Guided Representations for Scoring Figure Skating
IEEE TRANSACTIONS ON MULTIMEDIA(2024)
摘要
This paper explores semantic-aware representations for scoring figure skating videos. Most existing approaches to sports video analysis only focus on reasoning action scores based on visual input, limiting their ability to depict high-level semantic representations. Here, we propose a teacher-student-based network with an attention mechanism to realize an adaptive knowledge transfer from the semantic domain to the visual domain, which is termed semantics-guided network (SGN). Specifically, we use a set of learnable atomic queries in the student branch to mimic the semantic-aware distribution in the teacher branch, which is represented by the visual and semantic inputs. In addition, we propose three auxiliary losses to align features in different domains. With aligned feature representations, the adapted teacher is capable of transferring the semantic knowledge to the student. To verify the effectiveness of our method, we collect a new dataset OlympicFS for scoring figure skating. Besides action scores, OlympicFS also provides professional comments on actions for learning semantic representations. By evaluating four challenging datasets, our method achieves state-of-the-art performance.
更多查看译文
关键词
Figure skating videos,sports video analysis,multi-modality representation learning,teacher-student network,action quality assessment
AI 理解论文
溯源树
样例
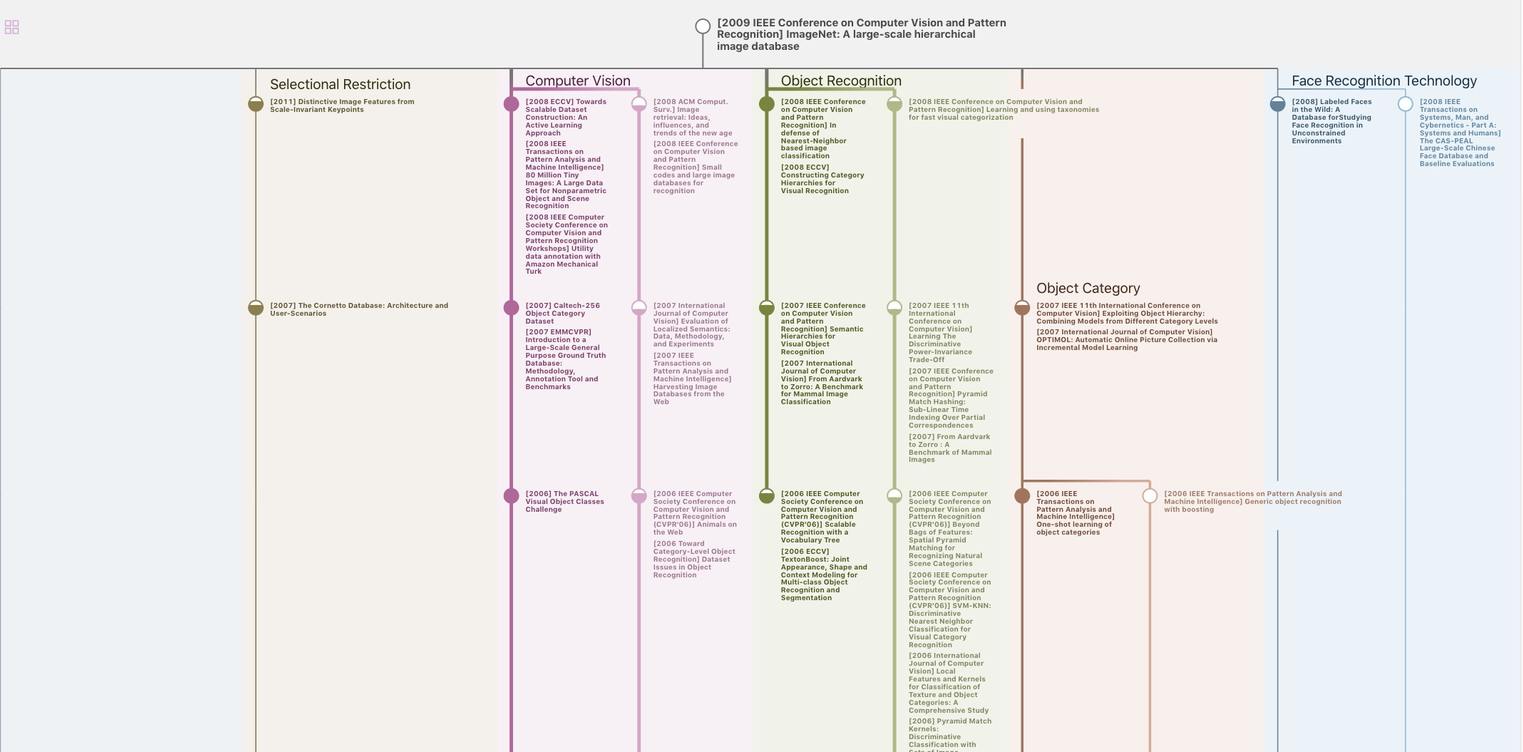
生成溯源树,研究论文发展脉络
Chat Paper
正在生成论文摘要