Multi-View Ensemble Federated Learning for Efficient Prediction of Consumer Electronics Applications in Fog Networks.
IEEE Trans. Consumer Electron.(2024)
摘要
Federated Learning (FL) collaboratively trains a model while preserving privacy and providing intelligence. This makes it ideal for Consumer Electronics (CE) applications, involving continuous streaming of data. However, the existence of a single central server for orchestrating the entire process and the participation of an abundant number of clients introduce major bottlenecks like a single-point failure of the server and communication inefficiency. Additionally, in most CE applications, local data is used by the clients as a single dataset, which fails to retrieve deep insights from data features for better decision-making. In this light, we propose a multi-view ensemble FL framework for CE applications in fog networks, namely EnsembleFed. The proposed approach derives multiple views from raw data to gain deeper insights from it and trains a model for each view. Later, the output of these models is ensembled for robust predictions. The distributed fog nodes are incorporated into the FL framework to combat single-point failure risk and improve communication efficiency. Empirical results demonstrate that the proposed approach outperforms state-of-the-art techniques on a real-time dataset in terms of increased accuracy by 4.02%, 4.32%, and 5.27%, compared to FedYogi, FedAdam, and FedAvg, respectively.
更多查看译文
关键词
Consumer Electronics,Federated Learning,Fog Computing,Multi-view Ensemble,Communication Efficiency
AI 理解论文
溯源树
样例
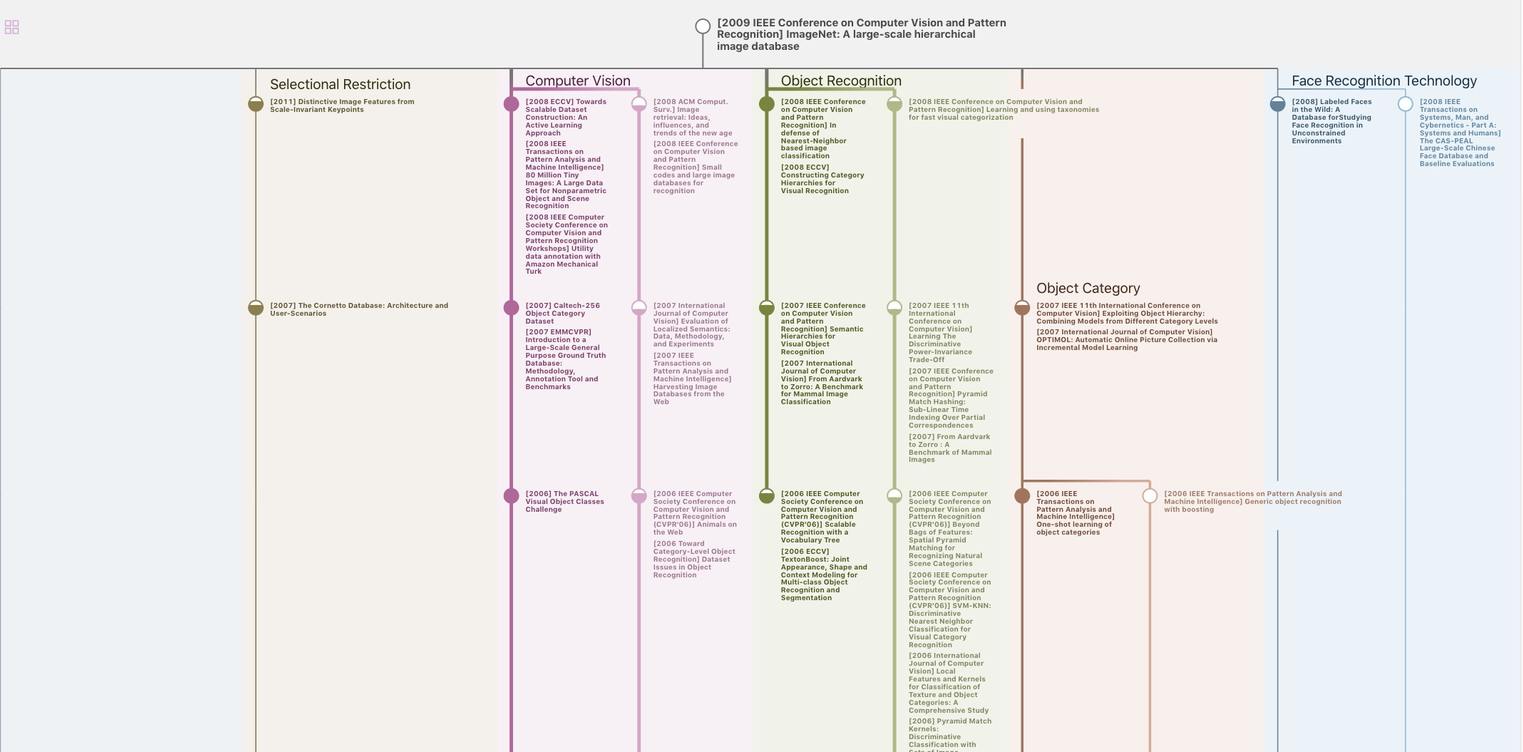
生成溯源树,研究论文发展脉络
Chat Paper
正在生成论文摘要