Hierarchical Bayesian Causality Network to Extract High-Level Semantic Information in Visual Cortex
INTERNATIONAL JOURNAL OF NEURAL SYSTEMS(2024)
摘要
Functional MRI (fMRI) is a brain signal with high spatial resolution, and visual cognitive processes and semantic information in the brain can be represented and obtained through fMRI. In this paper, we design single-graphic and matched/unmatched double-graphic visual stimulus experiments and collect 12 subjects' fMRI data to explore the brain's visual perception processes. In the double-graphic stimulus experiment, we focus on the high-level semantic information as "matching", and remove tail-to-tail conjunction by designing a model to screen the matching-related voxels. Then, we perform Bayesian causal learning between fMRI voxels based on the transfer entropy, establish a hierarchical Bayesian causal network (HBcausalNet) of the visual cortex, and use the model for visual stimulus image reconstruction. HBcausalNet achieves an average accuracy of 70.57% and 53.70% in single- and double-graphic stimulus image reconstruction tasks, respectively, higher than HcorrNet and HcasaulNet. The results show that the matching-related voxel screening and causality analysis method in this paper can extract the "matching" information in fMRI, obtain a direct causal relationship between matching information and fMRI, and explore the causal inference process in the brain. It suggests that our model can effectively extract high-level semantic information in brain signals and model effective connections and visual perception processes in the visual cortex of the brain.
更多查看译文
关键词
Cognitive computing,visual cognition,semantic information,fMRI,Bayesian network,hierarchical Bayesian causality network
AI 理解论文
溯源树
样例
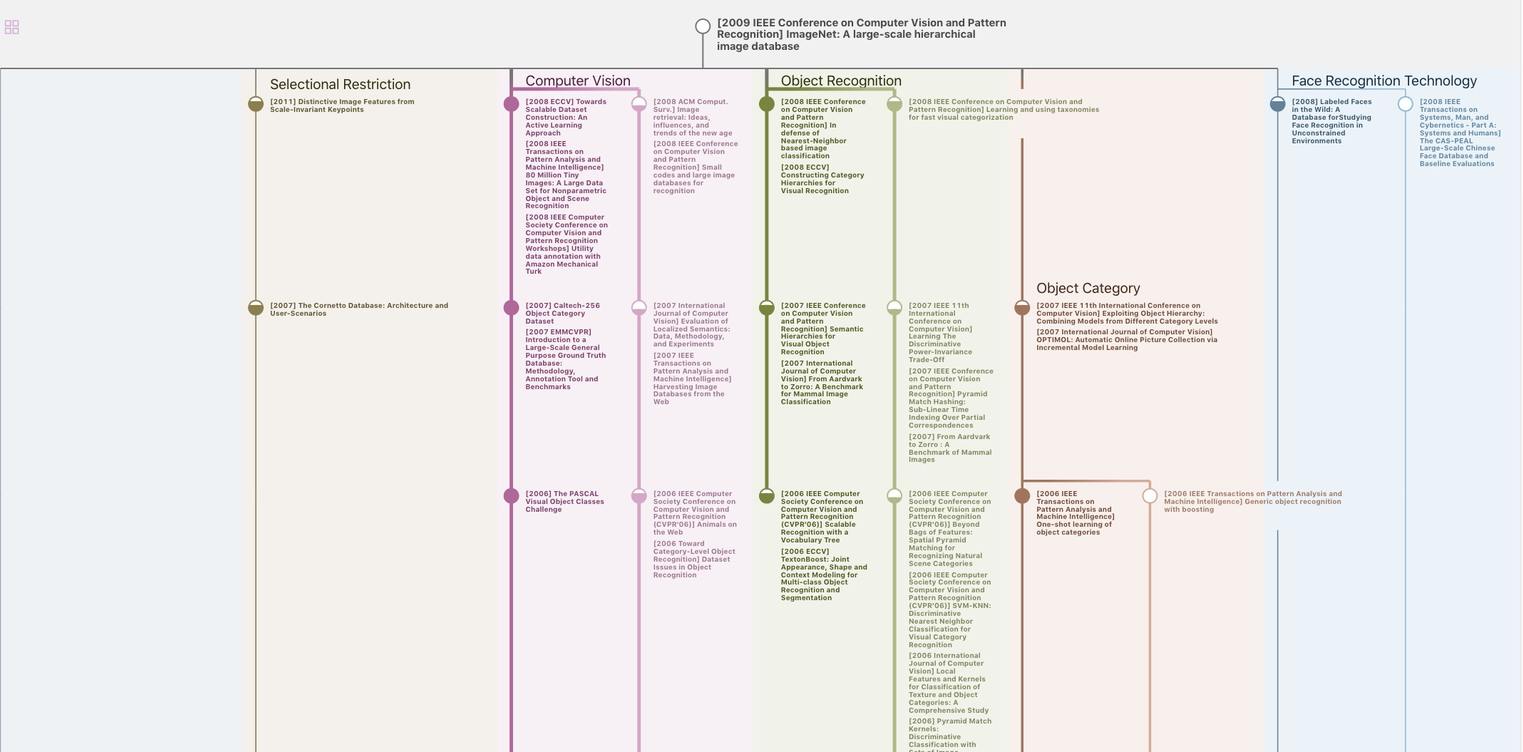
生成溯源树,研究论文发展脉络
Chat Paper
正在生成论文摘要