Complementing a continuum thermodynamic approach to constitutive modeling with symbolic regression
JOURNAL OF THE MECHANICS AND PHYSICS OF SOLIDS(2023)
摘要
An interpretable machine learning method, physics-informed genetic programming-based sym-bolic regression (P-GPSR), is integrated into a continuum thermodynamic approach to devel-oping constitutive models. The proposed strategy for combining a thermodynamic analysis with P-GPSR is demonstrated by generating a yield function for an idealized material with voids, i.e., the Gurson yield function. First, a thermodynamic-based analysis is used to derive model requirements that are exploited in a custom P-GPSR implementation as fitness criteria or are strongly enforced in the solution. The P-GPSR implementation improved accuracy, generalizability, and training time compared to the same GPSR code without physics-informed fitness criteria. The yield function generated through the P-GPSR framework is in the form of a composite function that describes a class of materials and is characteristically more interpretable than GPSR-derived equations. The physical significance of the input functions learned by P-GPSR within the composite function is acquired from the thermodynamic analysis. Fundamental explanations of why the implemented P-GPSR capabilities improve results over a conventional GPSR algorithm are provided.
更多查看译文
关键词
Physics-informed machine learning,Constitutive modeling,Symbolic regression,Thermodynamics,Porous material,Multi-tree GPSR,Multi-objective optimization
AI 理解论文
溯源树
样例
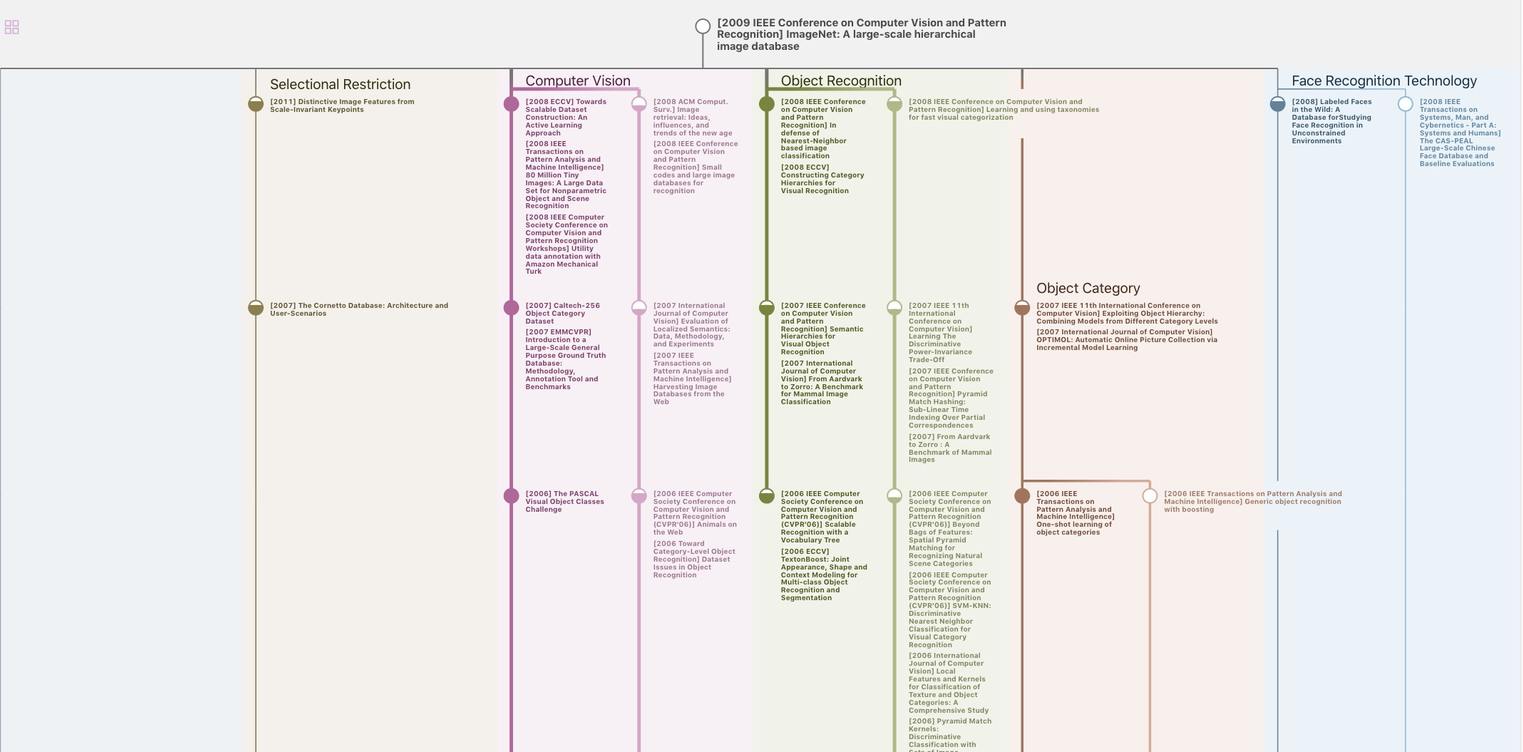
生成溯源树,研究论文发展脉络
Chat Paper
正在生成论文摘要