Global and Local Spatio-Temporal Encoder for 3D Human Pose Estimation.
IEEE Trans. Multim.(2024)
摘要
Transformers have been used for 3D human pose estimation with excellent performance; however, most transformers focus on encoding the global spatio-temporal correlation of all joints in the human body and there are few studies on the local Spatio-temporal correlation of each joint in the human body. In this paper, we propose a Global and Local Spatio-Temporal Encoder (GLSTE) to model the Spatio-temporal correlation. Specifically, a Global Spatial Encoder (GSE) and a Global Temporal Encoder (GTE) are constructed to capture the global spatial information of all joints in a single frame and the global temporal information of all frames, respectively. A Local Spatio-Temporal Encoder (LSTE) is constructed to capture the spatial and temporal information of each joint in the local N frames. Furthermore, we propose a parallel attention module with weight sharing to better incorporate spatial and temporal information into each node simultaneously. Extensive experiments show that GLSTE outperforms state-of-the-art methods with fewer parameters and less computational overhead on two challenging datasets: Human3.6M and MPI-INF-3DHP. Especially in the evaluation of Human3.6M dataset, the results of our method with 27 frames as input are better than the vast majority of recent SOTA methods with 81 and 243 frames as input, which indicates that the model can learn more useful information with smaller inputs.
更多查看译文
关键词
3D Human Pose Estimation,Transformer,Spatio-Temporal Encoder,parallel attention
AI 理解论文
溯源树
样例
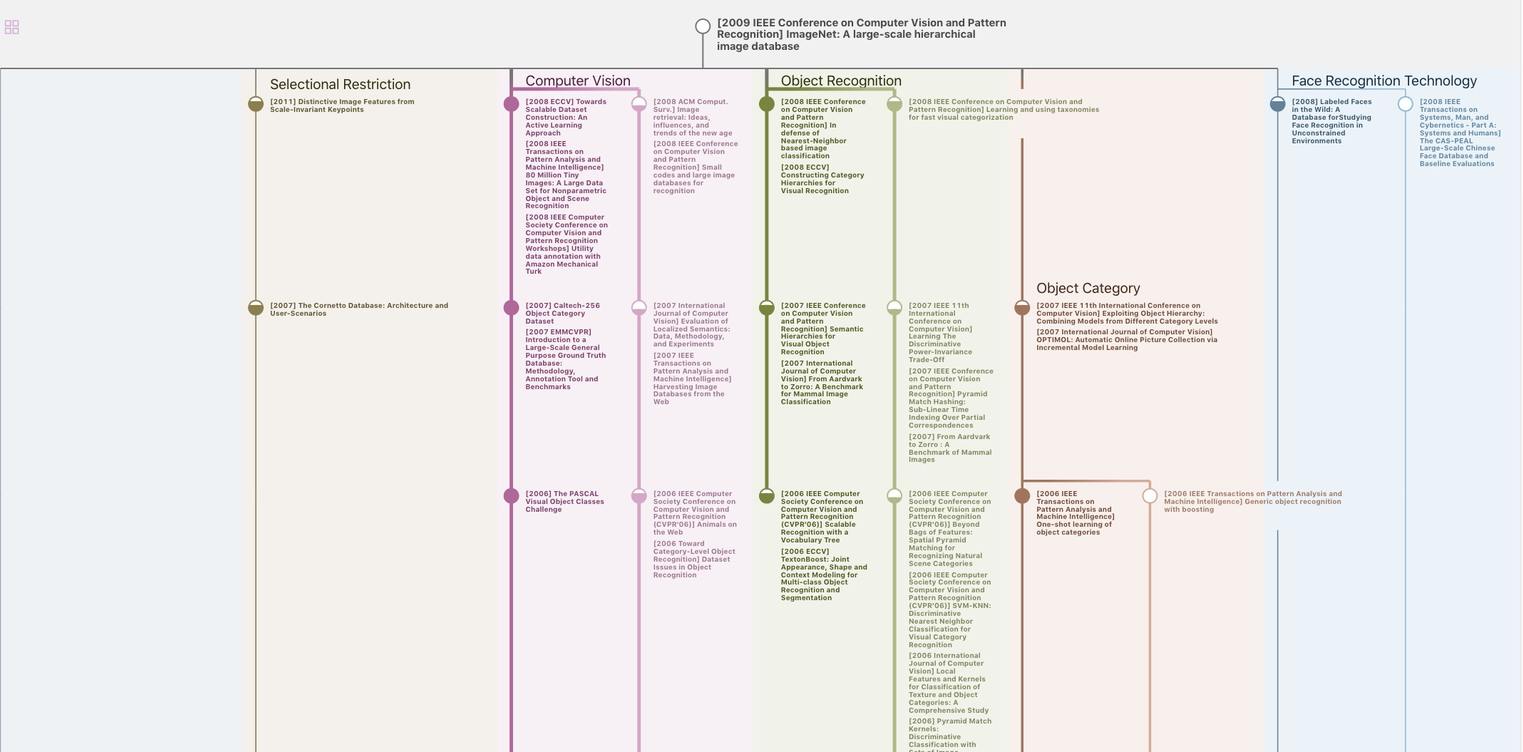
生成溯源树,研究论文发展脉络
Chat Paper
正在生成论文摘要