Payload Transporting With Two Quadrotors by Centralized Reinforcement Learning Method
IEEE TRANSACTIONS ON AEROSPACE AND ELECTRONIC SYSTEMS(2024)
摘要
Nowadays, quadrotors find applications in automation and artificial intelligence. Among diverse quadrotor studies, payload transport stands out, posing implementation challenges. Using multiple quadrotors reduces per-quadrotor load while increasing system complexity. Inspired by model-free reinforcement learning, we apply it to position control in a nonlinear two-quadrotor payload system. Our approach employs a reinforcement learning agent guided by the twin delay deep deterministic policy gradient (TD3) algorithm. Its goal is accurate cable-suspended payload delivery and system stabilization. We test the method's robustness by adding noise. Simulation results show that TD3 excels in ideal conditions and handles noise during training and testing, highlighting its effectiveness. This article's scope can be expanded to encompass scenarios involving three or more quadrotors, providing valuable insights for future endeavors.
更多查看译文
关键词
Quadrotors,Payloads,Reinforcement learning,Mathematical models,Angular velocity,Torque,Symbols,Continuous action space,continuous state space,reinforcement learning (RL),twin delayed deep deterministic policy gradient (TD3),two-quadrotor transporting payload system
AI 理解论文
溯源树
样例
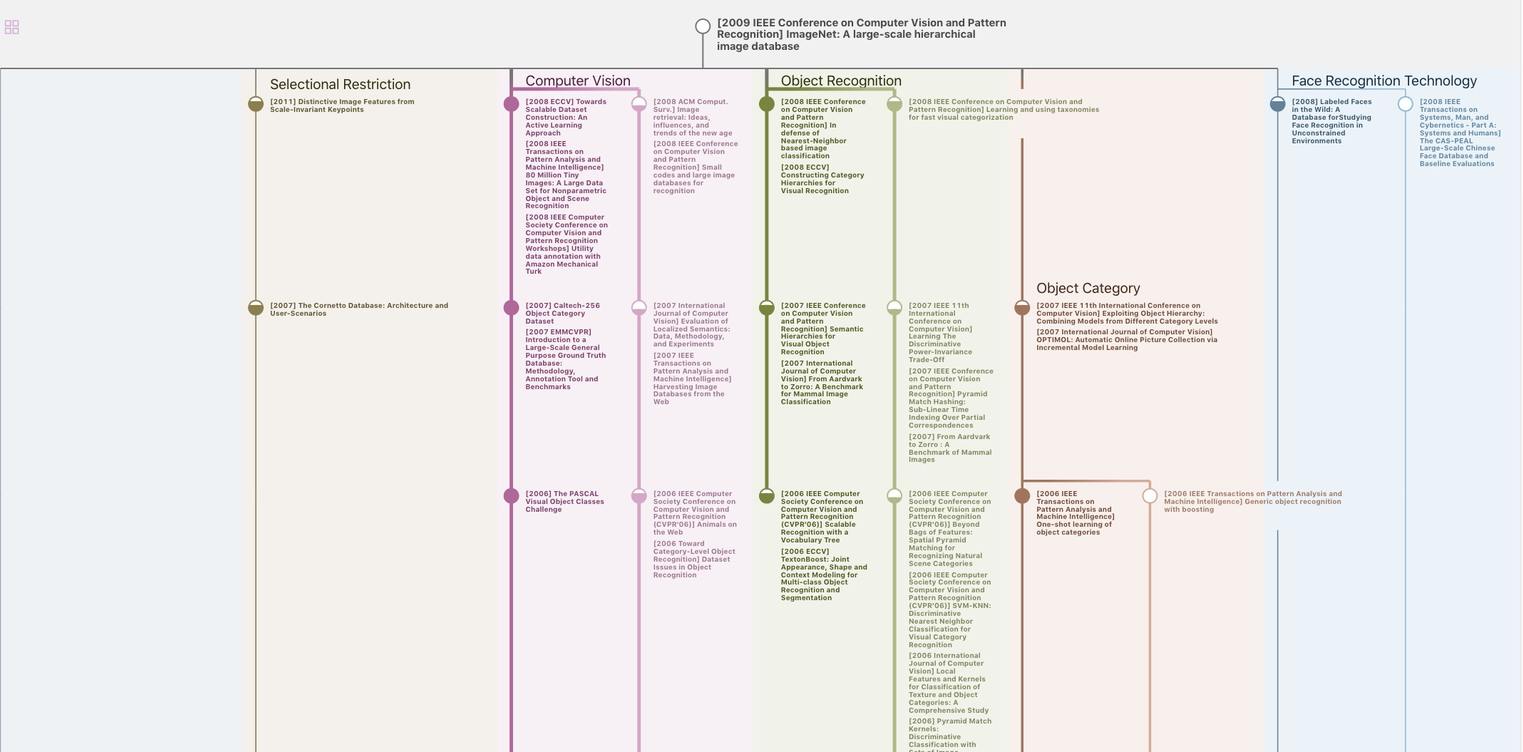
生成溯源树,研究论文发展脉络
Chat Paper
正在生成论文摘要