Research on Adaptive Network Recovery Method Based on Key Node Identification
Lecture notes in electrical engineering(2023)
Abstract
As the world becomes increasingly interconnected, network failures have emerged as a practical problem that organizations must tackle. While extensive research has been carried out on network recovery in the past decade, previous studies have often neglected attribute information such as node capacity and status, resulting in inaccurate identification of key nodes and suboptimal network performance recovery. We aims to optimize the representation of failed networks, embedding both node attribute information and topological information using graph neural network. A deep reinforcement learning approach is employed to find the optimal node recovery sequence, leading to the proposal of a network recovery method called R-GQN, based on graph neural network and deep Q-learning. The performance of network recovery speed is evaluated by the normalized cumulative recovery efficiency (NCRE). To evaluate the proposed method, experiments were conducted on Barábasi-Albert scale-free networks of different sizes and compared with four existing network recovery methods: degree centrality, betweenness centrality, closeness centrality, and PageRank. The results show that the R-GQN method outperforms these methods by 10.32% in terms of NCRE, demonstrating its effectiveness in network recovery.
MoreTranslated text
Key words
adaptive network recovery method,node
AI Read Science
Must-Reading Tree
Example
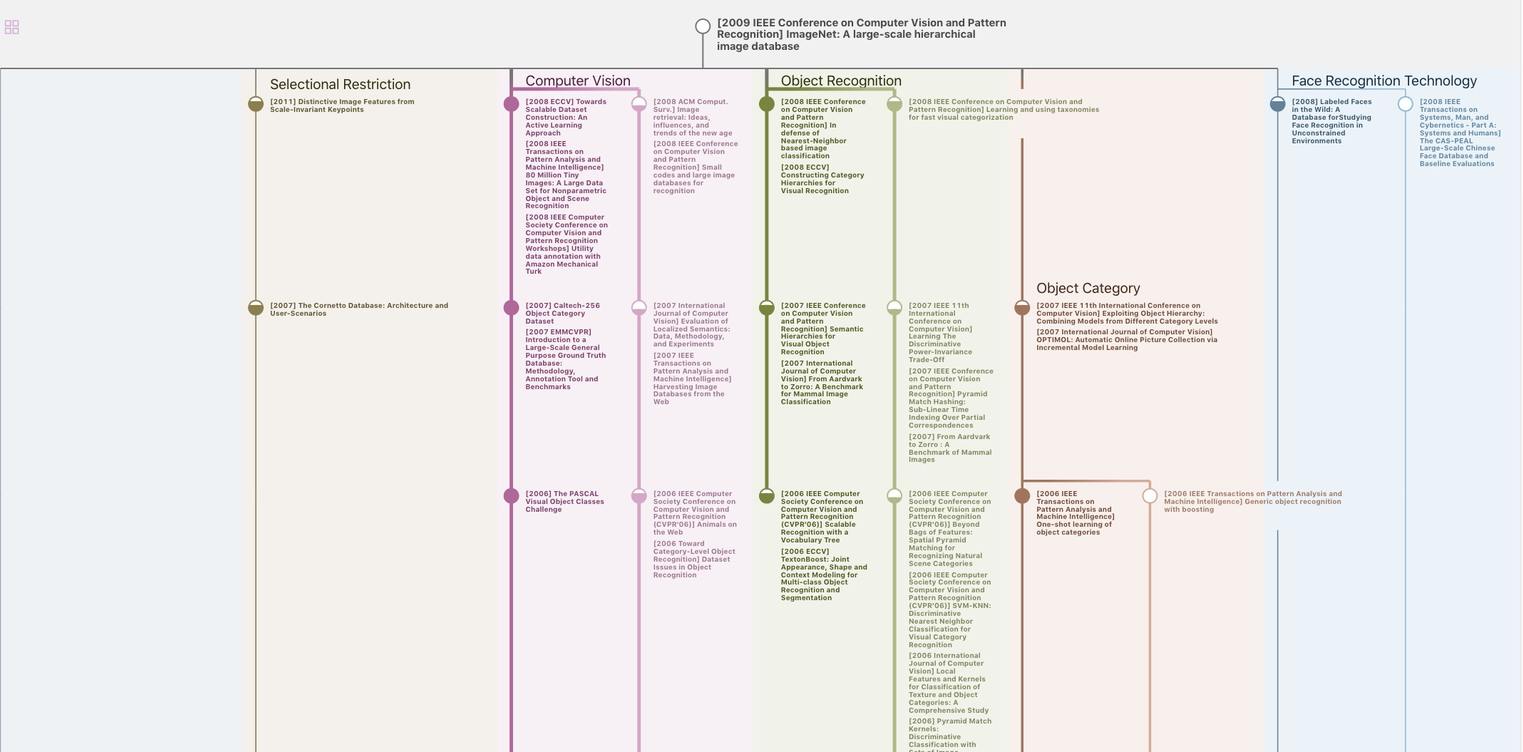
Generate MRT to find the research sequence of this paper
Chat Paper
Summary is being generated by the instructions you defined