SGCRNN: A ChebNet-GRU fusion model for eeg emotion recognition
JOURNAL OF INTELLIGENT & FUZZY SYSTEMS(2023)
摘要
The paper proposes a deep learning model based on Chebyshev Network Gated Recurrent Units, which is called Spectral Graph Convolution Recurrent Neural Network, for multichannel electroencephalogram emotion recognition. First, in this paper, an adjacency matrix capturing the local relationships among electroencephalogram channels is established based on the cosine similarity of the spatial locations of electroencephalogram electrodes. The training efficiency is improved by utilizing the computational speed of the cosine distance. This advantage enables our method to have the potential for real-time emotion recognition, allowing for fast and accurate emotion classification in real-time application scenarios. Secondly, the spatial and temporal dependence of the Spectral Graph Convolution Recurrent Neural Network for capturing electroencephalogram sequences is established based on the characteristics of the Chebyshev network and Gated Recurrent Units to extract the spatial and temporal features of electroencephalogram sequences. The proposed model was tested on the publicly accessible dataset DEAP. Its average recognition accuracy is 88%, 89.5%, and 89.7% for valence, arousal, and dominance, respectively. The experiment results demonstrated that the Spectral Graph Convolution Recurrent Neural Network method performed better than current models for electroencephalogram emotion identification. This model has broad applicability and holds potential for use in real-time emotion recognition scenarios.
更多查看译文
关键词
Electroencephalogram,emotion recognition,chebyshev network gated recurrent units,spectral graph convolution recurrent neural network,adjacency matrix
AI 理解论文
溯源树
样例
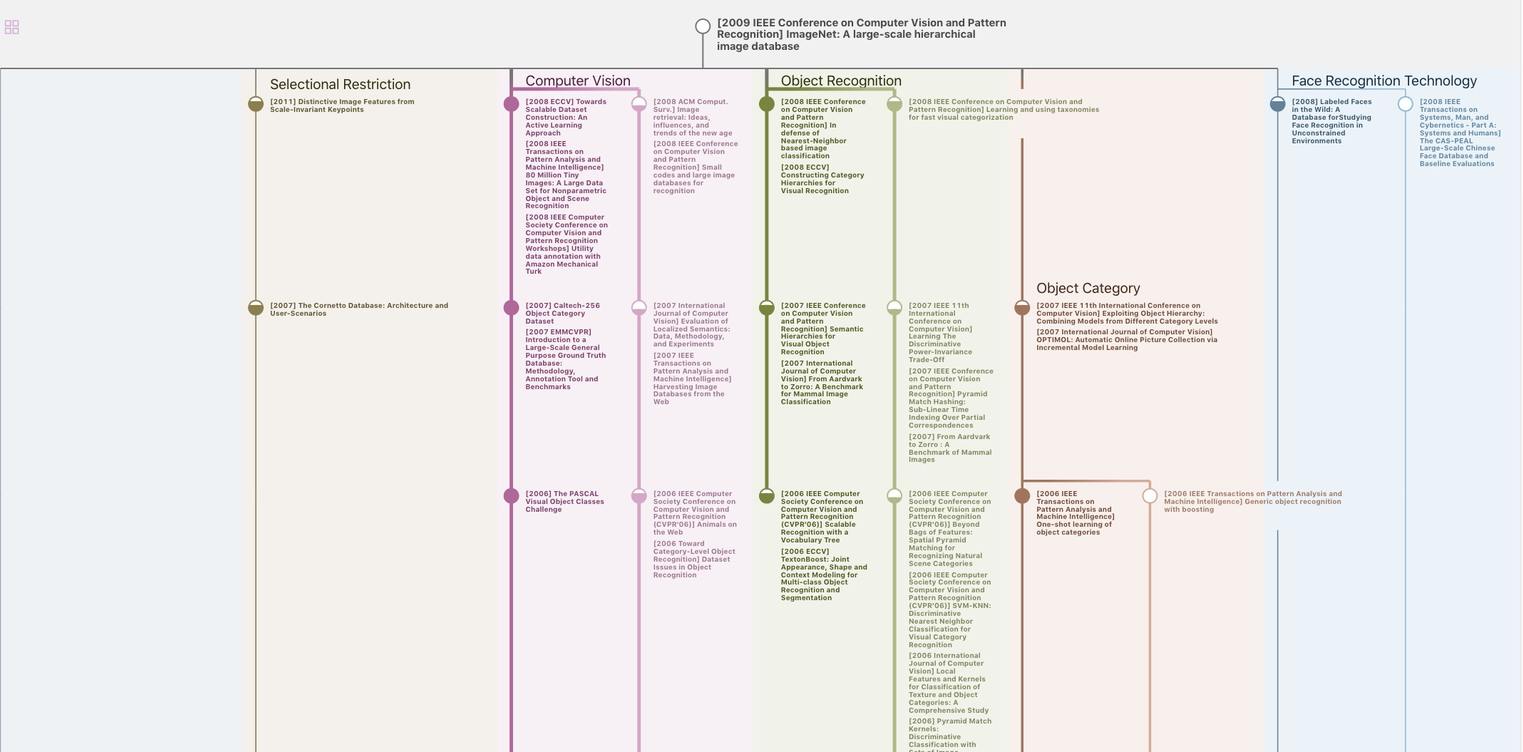
生成溯源树,研究论文发展脉络
Chat Paper
正在生成论文摘要