Posterior Contraction Rates for Mat\'ern Gaussian Processes on Riemannian Manifolds
arXiv (Cornell University)(2023)
摘要
Gaussian processes are used in many machine learning applications that rely on uncertainty quantification. Recently, computational tools for working with these models in geometric settings, such as when inputs lie on a Riemannian manifold, have been developed. This raises the question: can these intrinsic models be shown theoretically to lead to better performance, compared to simply embedding all relevant quantities into $\mathbb{R}^d$ and using the restriction of an ordinary Euclidean Gaussian process? To study this, we prove optimal contraction rates for intrinsic Mat\'ern Gaussian processes defined on compact Riemannian manifolds. We also prove analogous rates for extrinsic processes using trace and extension theorems between manifold and ambient Sobolev spaces: somewhat surprisingly, the rates obtained turn out to coincide with those of the intrinsic processes, provided that their smoothness parameters are matched appropriately. We illustrate these rates empirically on a number of examples, which, mirroring prior work, show that intrinsic processes can achieve better performance in practice. Therefore, our work shows that finer-grained analyses are needed to distinguish between different levels of data-efficiency of geometric Gaussian processes, particularly in settings which involve small data set sizes and non-asymptotic behavior.
更多查看译文
关键词
gaussian,contraction
AI 理解论文
溯源树
样例
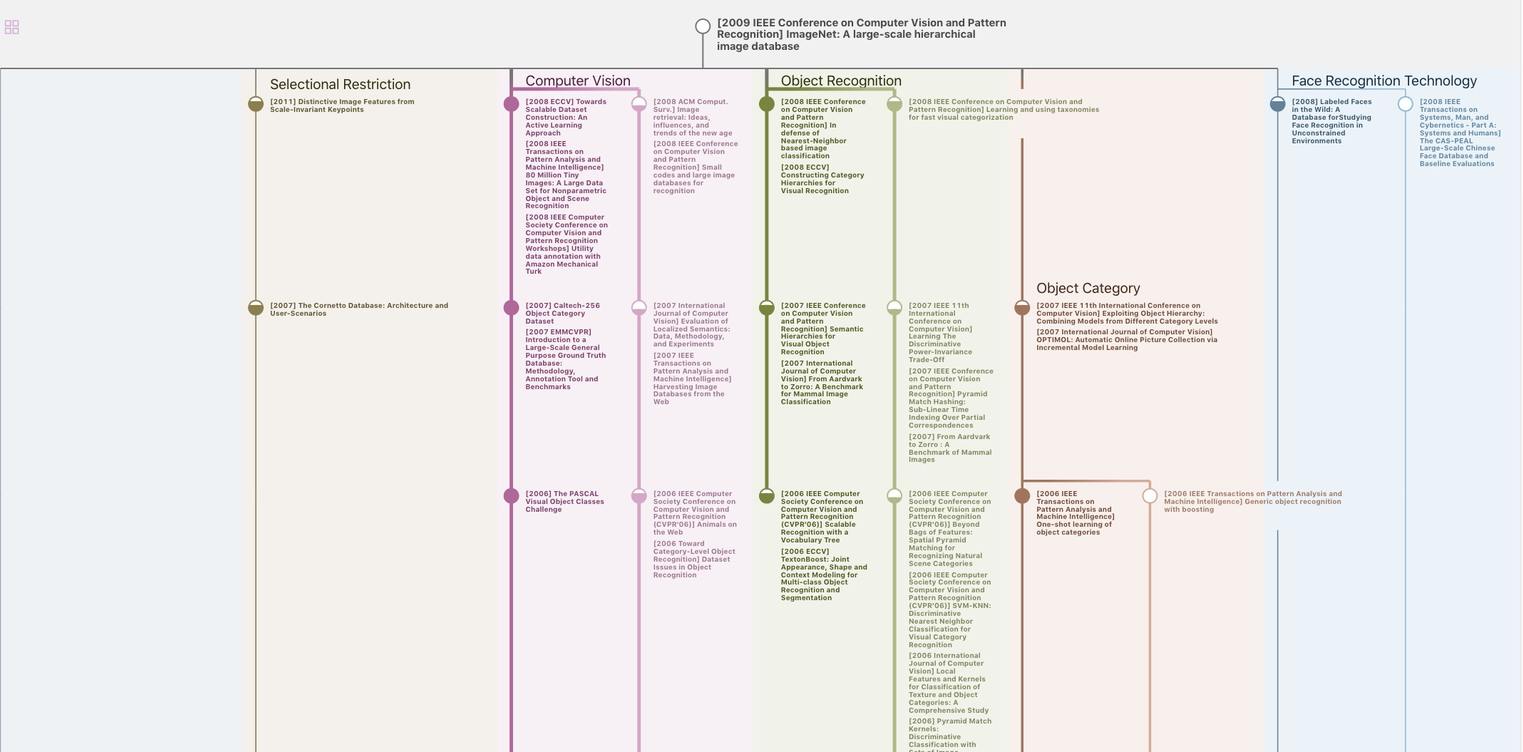
生成溯源树,研究论文发展脉络
Chat Paper
正在生成论文摘要