Label-Guided Graphormer for Hierarchy Text Classification
ARTIFICIAL NEURAL NETWORKS AND MACHINE LEARNING, ICANN 2023, PT V(2023)
摘要
Hierarchical text classification (HTC), a subtask of multilabel classification, remains a challenging problem due to its complex label topology hierarchy. Although various methods have been proposed for modeling hierarchical information in recent research, less attention has been paid to techniques that utilize label information hidden in the training data, which can be potentially beneficial for hierarchical classification. In this paper, we focus on enhancing label representations from the perspective of contextual interactions and semantic complements, which refer to label co-occurrences and keywords respectively. And a Label-guided Graphormer (LGraphormer) is proposed for the purpose of integrating these promising features. The final prediction is performed by feeding its output embeddings into a text encoder in a hierarchyaware manner. Our extensive experiments show that the proposed approach achieves state-of-the-art performance on three widely used public datasets, demonstrating the effectiveness of our methodology. Our code is available at: https://github.com/ContentTech/LGraphormerHTC.git.
更多查看译文
关键词
hierarchical text classification,data information,label represent enhancement,Graphormer net
AI 理解论文
溯源树
样例
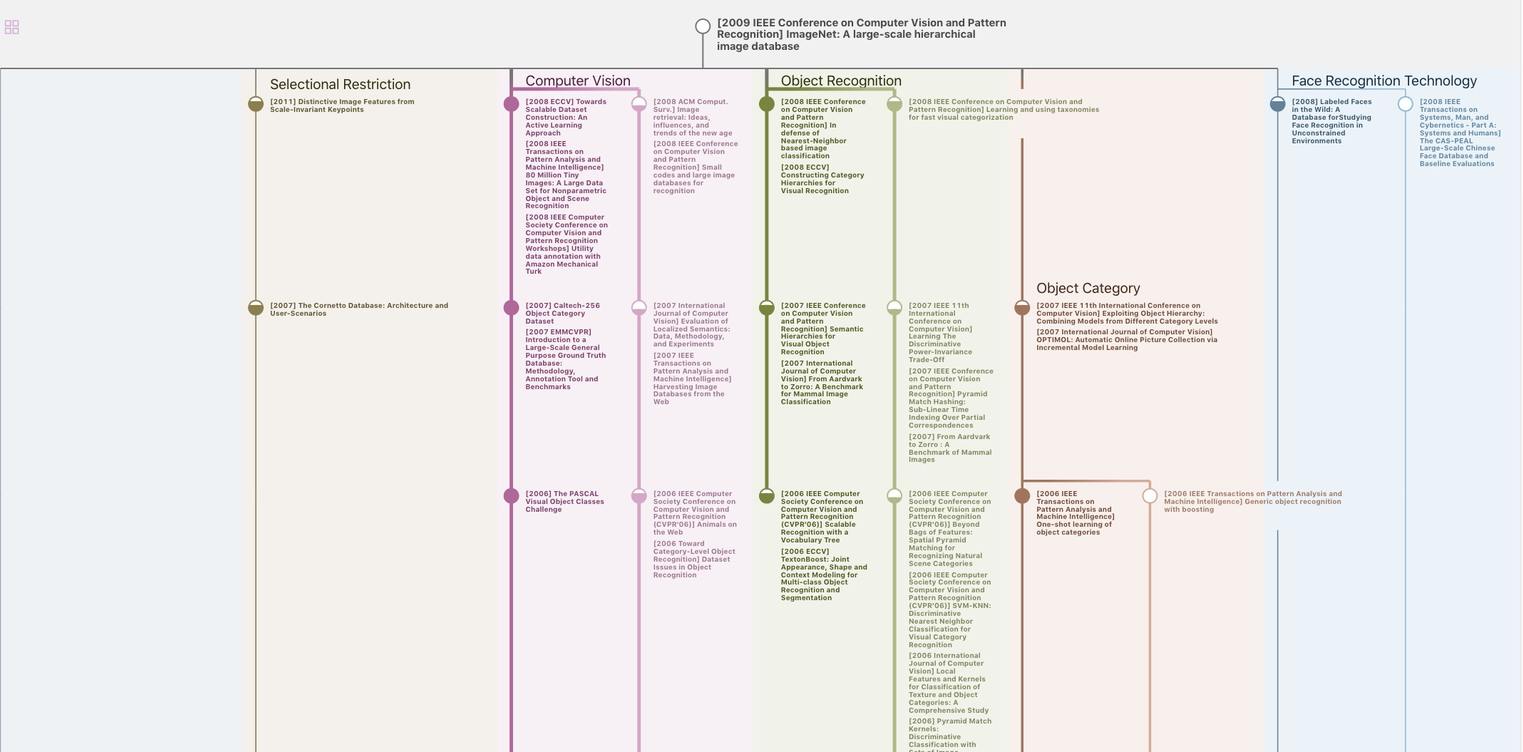
生成溯源树,研究论文发展脉络
Chat Paper
正在生成论文摘要