Multiple Source Localization in IoT: A Conditional GAN and Image-Processing-Based Framework.
IEEE Internet Things J.(2024)
摘要
This paper addresses the problem of multiple source localization (MSL) using the Internet of Things (IoT) sensors. MSL entails determining the locations of multiple unknown sources by fusing sensory data within a designated area of interest (AoI). Existing solutions suffer from limitations such as increased algorithmic complexity as the number of sources increases and degraded performance in sparse sensor placement scenarios. This paper proposes a novel source-independent approach resilient to sparse sensor placements based on conditional generative adversarial networks (cGANs) and image processing-based peak-finding with sub-pixel peak refinement to address the MSL problem. The proposed approach formulates the MSL problem into two sub-problems: (1) image-to-image translation and (2) two-dimensional peak-finding. The cGAN translates the raw measurement data to an intensity field through image-to-image translation. Then, a peak-finding algorithm based on persistent homology with sub-pixel peak refinement is applied to localize the unknown sources accurately. The proposed approach is tested through radioactive source localization experiments, benchmark comparisons, and adaptability evaluation in unseen environments.
更多查看译文
关键词
multiple sources,localization,sensor fusion,machine learning,deep learning,GANs,image processing,peak-finding,IoT
AI 理解论文
溯源树
样例
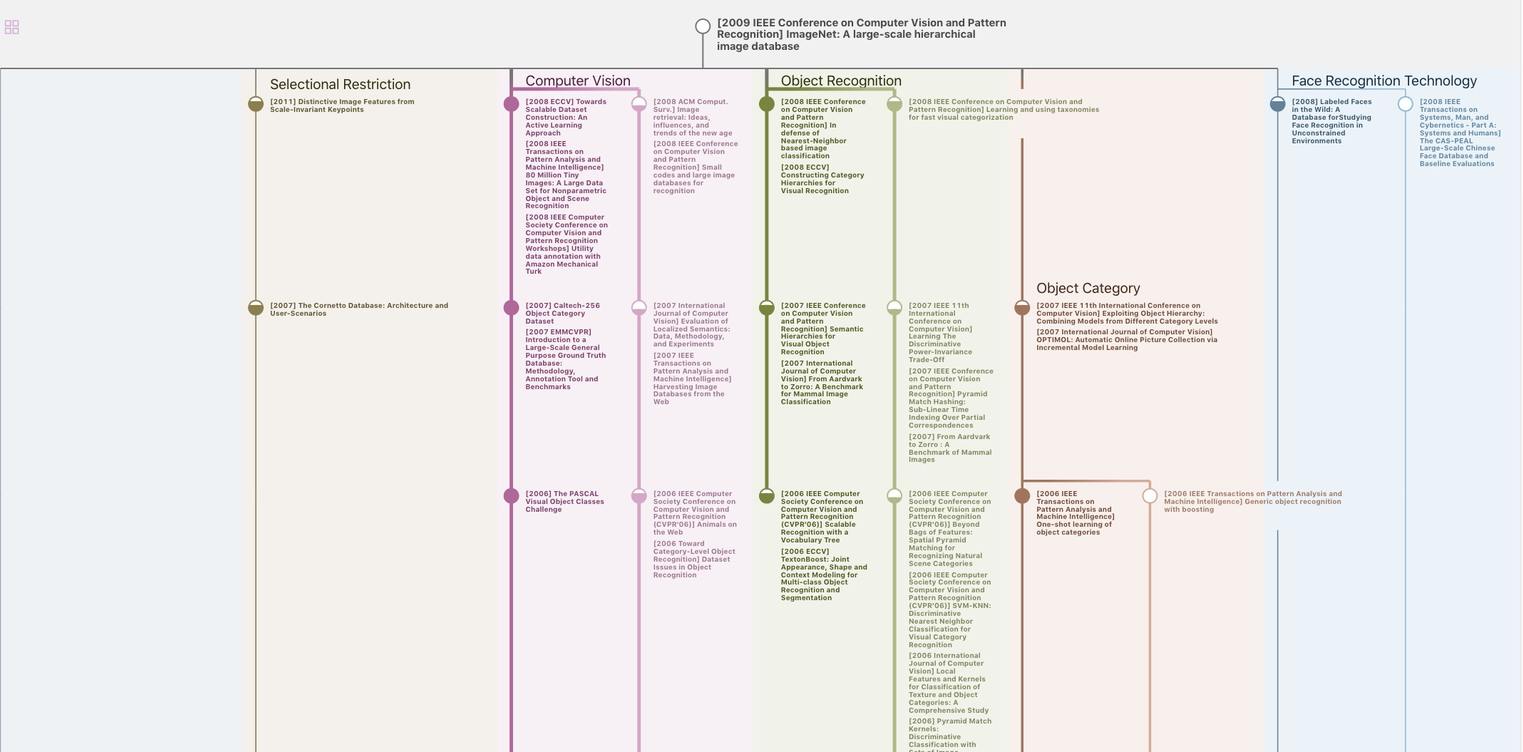
生成溯源树,研究论文发展脉络
Chat Paper
正在生成论文摘要