Predicting MACE from [82Rb] PET: Can AI outperformmore traditional quantitative assessment of themyocardial perfusion ?
Research Square (Research Square)(2023)
摘要
Abstract Assessing the individual risk of Major Adverse Cardiac Events (MACE) is of major importance as cardiovascular diseases remain the leading cause of death worldwide. Quantitative Myocardial Perfusion Imaging (MPI) parameters such as stress Myocardial Blood Flow (sMBF) or Myocardial Flow Reserve (MFR) constitutes the gold standard for prognosis assessment. We propose a systematic investigation of the value of Artificial Intelligence (AI) to leverage [82Rb] Silicon PhotoMultiplier (SiPM) PET MPI for MACE prediction. We establish a general pipeline for AI model validation to assess and compare the performance of global (i.e. average of the entire MPI signal), regional (17 segments), radiomics and Convolutional Neural Network (CNN) models leveraging various MPI signals on a dataset of 234 patients. Results showed that all regional AI models significantly outperformed the global model (p < 0.001), where the best AUC of 73.9% (CI: 72.5-75.3) was obtained with a CNN model. A regional AI model based on MBF averages from 17 segments fed to a Logistic Regression (LR) constituted an excellent trade-off between model simplicity and performance, achieving an AUC of 73.4% (CI: 72.3-74.7). A radiomics model based on intensity features revealed that the global average was the least important feature when compared to other aggregations of the MPI signal over the myocardium. We conclude that AI models can allow better personalized prognosis assessment for MACE.
更多查看译文
关键词
themyocardial perfusion,mace,pet,traditional quantitative assessment
AI 理解论文
溯源树
样例
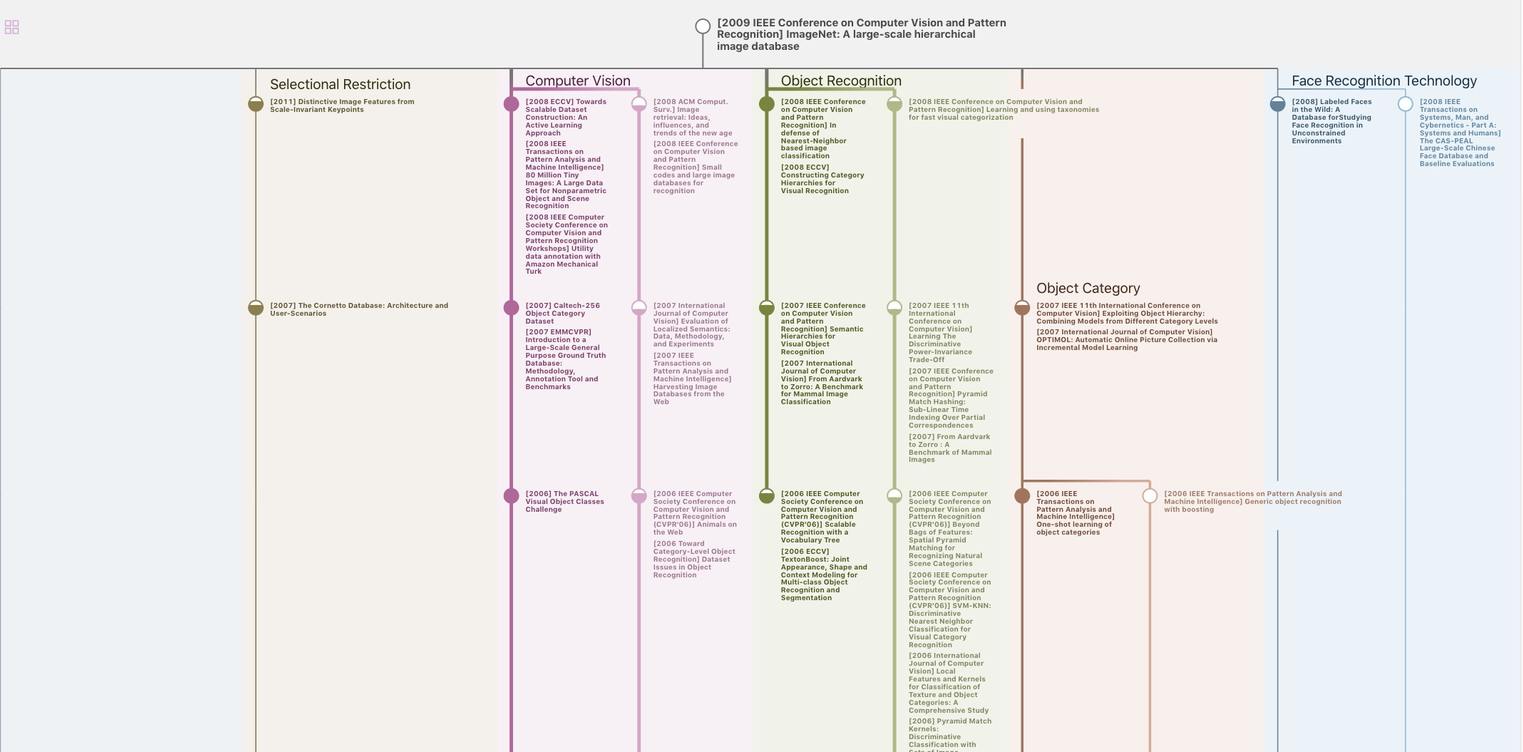
生成溯源树,研究论文发展脉络
Chat Paper
正在生成论文摘要