Domain-Aware Prototype Network for Generalized Zero-Shot Learning.
IEEE Trans. Circuits Syst. Video Technol.(2024)
摘要
Generalized zero-shot learning(GZSL) aims to recognize images from seen and unseen classes with side information, such as manually annotated attribute vectors. Traditional methods focus on mapping images and semantics into a common latent space, thus achieving the visual-semantics alignment. Since the unseen classes are unavailable during training, there is a serious problem of recognition bias, which will tend to recognize unseen classes as seen classes. To solve this problem, we propose a Domain-aware Prototype Network(DPN), which splits the GZSL problem into the seen class recognition and unseen class recognition problem. For the seen classes, we design a domain-aware prototype learning branch with a dual attention feature encoder to capture the essential visual information, which aims to recognize the seen classes and discriminate the novel categories. To further recognize the fine-grained unseen classes, a visual-semantic embedding branch is designed, which aims to align the visual and semantic information for unseen-class recognition. Through the multi-task learning of the prototype learning branch and visual-semantic embedding branch, our model can achieve excellent performance on three popular GZSL datasets.
更多查看译文
关键词
Generalized Zero-Shot Learning,transformer-based dual attention,domain detection
AI 理解论文
溯源树
样例
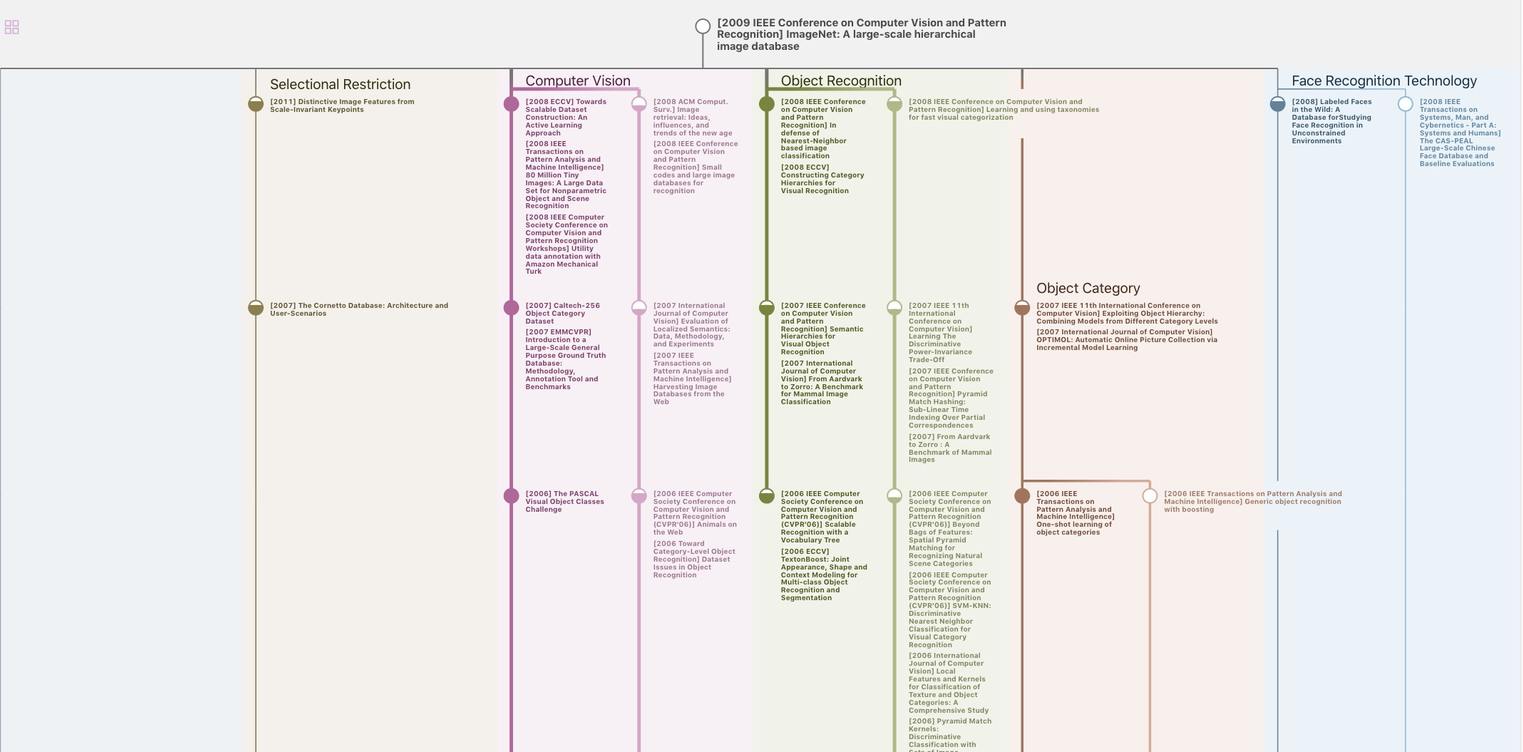
生成溯源树,研究论文发展脉络
Chat Paper
正在生成论文摘要