Dual-GAN Complementary Learning for Real-World Image Denoising
IEEE SENSORS JOURNAL(2024)
摘要
The imaging process of real-world images is inevitably polluted by noise, which affects the visual quality and subsequent processing of images. How to restore image details while removing noise has always been a challenging problem. The existing complementary learning strategies combine the advantages of both denoised image learning and noise learning and have good denoised effects. However, these methods that are based on a single generative adversarial network (GAN) suffer from complex network structure, difficulty in training, and further improvement. Therefore, we propose the dual-GAN complementary learning (DGCL) strategy based on modular complementary learning strategy. The method based on this strategy has been verified on the real-world image denoising datasets [PolyU and smartphone image denoising dataset (SIDD)]. The results show that this strategy has a better performance compared with similar denoising algorithm in terms of visual quality and quantitative measurement, and this strategy shows the potential to further improve the performance by improving a module in the strategy.
更多查看译文
关键词
Complementary learning,deep learning,generative adversarial network (GAN),image denoising
AI 理解论文
溯源树
样例
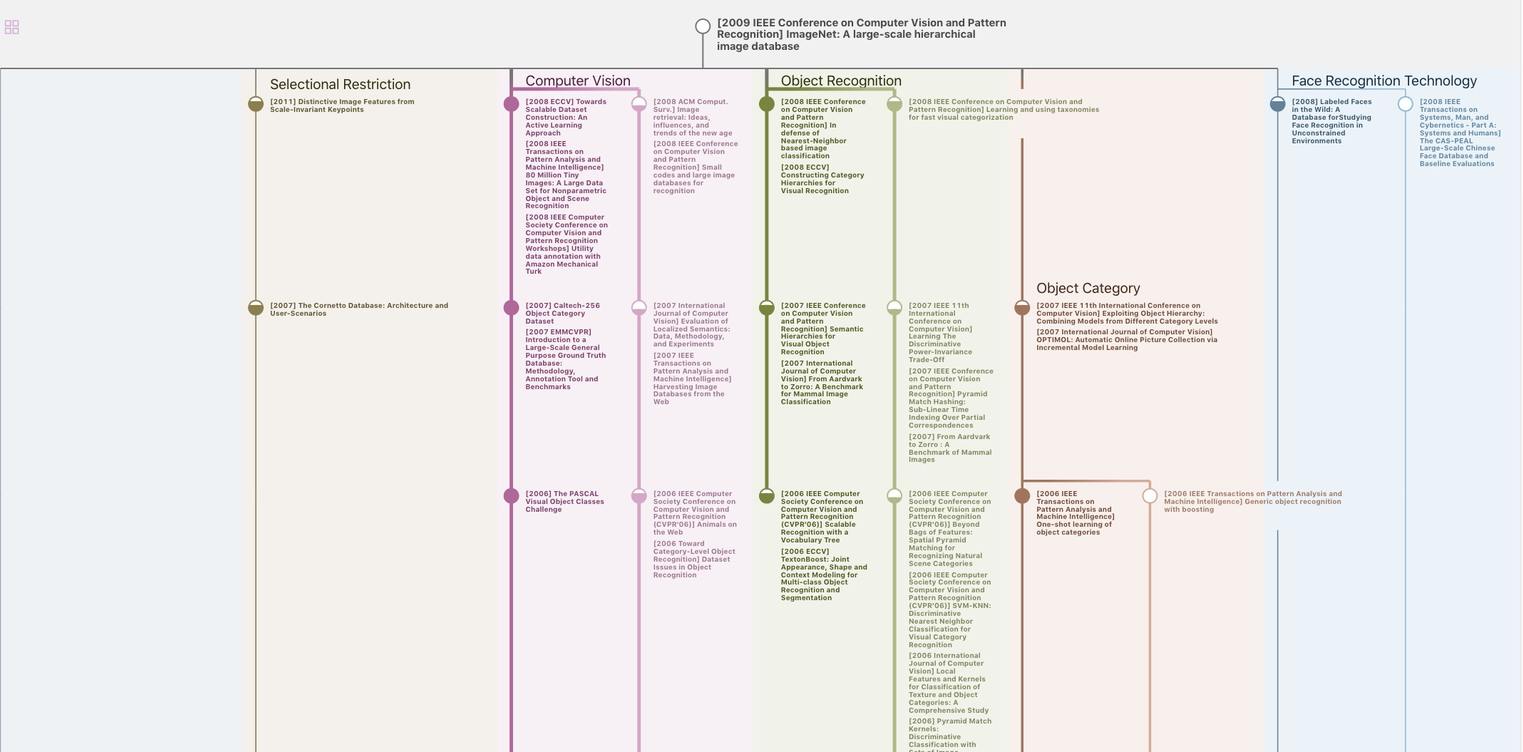
生成溯源树,研究论文发展脉络
Chat Paper
正在生成论文摘要