Deep Stereo Network With MRF-Based Cost Aggregation
IEEE TRANSACTIONS ON CIRCUITS AND SYSTEMS FOR VIDEO TECHNOLOGY(2024)
摘要
Despite the remarkable progress made in learning-based stereo-matching algorithms, it is an open challenge for stereo-matching in disparity discontinuities and textureless regions. In this paper, we propose the deep Markov Random Field based cost aggregation network (DMCA-Net) for stereo matching, which is an end-to-end model-driven network architecture. This architecture introduces an efficient feature extraction network to extract richer textual and contextual feature information for stereo feature similarity representation at multi-stages and levels. Furthermore, with the aim of alleviating the edge-fattening phenomenon at disparity discontinuities and generating accurate disparities in textureless regions, we proposed the differentiable Markov Random Field model for cost aggregation, where the model's data term utilizes image detail information, such as boundary and contour features, to guide matching cost aggregation, and the model's smoothness term penalizes the adjacency similarity of the cost between the four-nearest neighboring pixel pairs to predict the disparity in textureless regions. The detailed experiment demonstrates that DMCA network achieves competitive performance on the SceneFlow, KITTI 2012, KITTI 2015, and Middlebury 2014 datasets.
更多查看译文
关键词
Costs,Feature extraction,Estimation,Data models,Pipelines,Network architecture,Computer architecture,Stereo matching,MRF framework,unary feature maps,cost aggregation
AI 理解论文
溯源树
样例
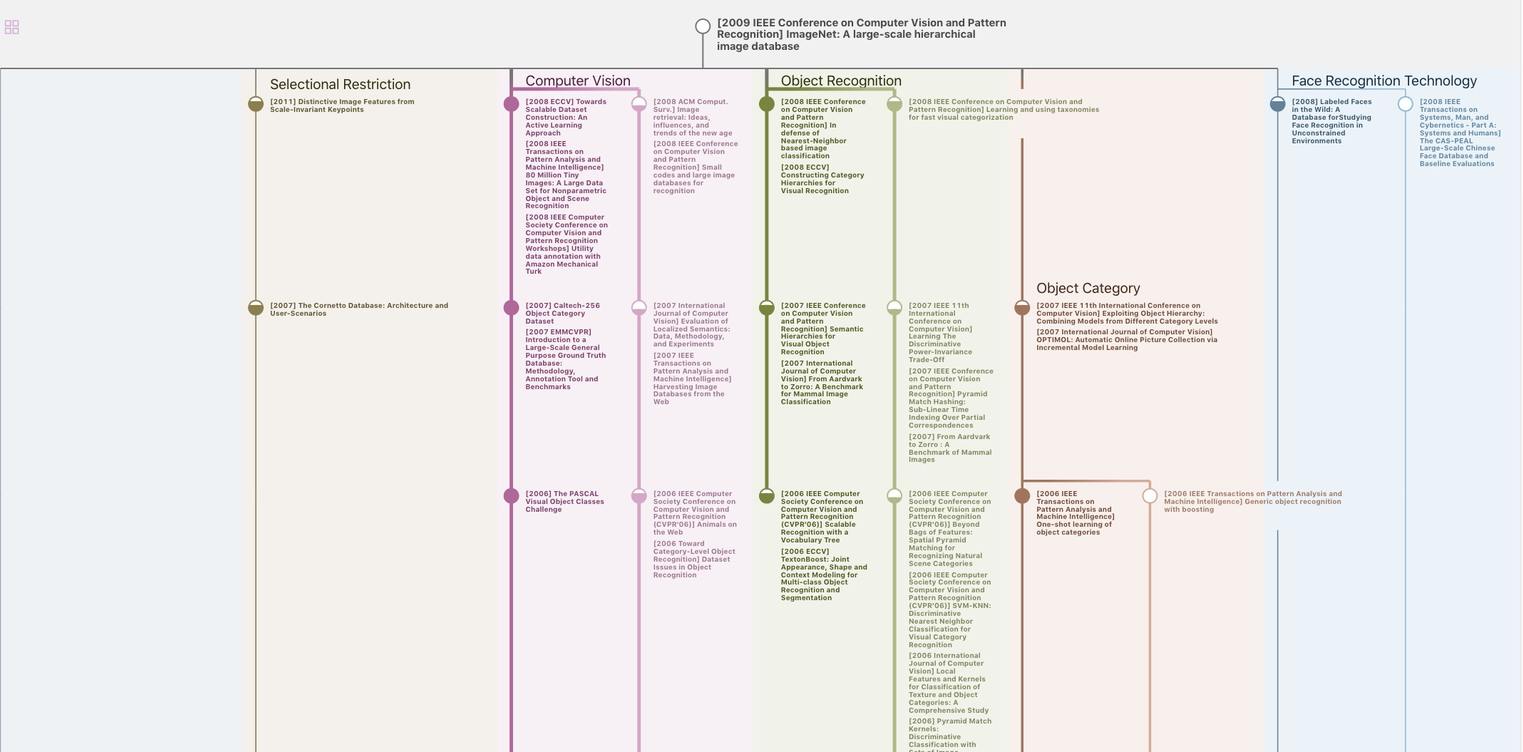
生成溯源树,研究论文发展脉络
Chat Paper
正在生成论文摘要