Improving the Post-Training Neural Network Quantization by Prepositive Feature Quantization
IEEE TRANSACTIONS ON CIRCUITS AND SYSTEMS FOR VIDEO TECHNOLOGY(2024)
Abstract
Post-training neural network quantization (PTQ) is an effective model compression technology that has revolutionized the deployment of deep neural networks on various edge devices. It provides easy-to-use characteristics and allows for generating a quantized model based on a pre-trained counterpart without re-training. Typical PTQ approaches maintain output consistency through layer-wise calibration. However, these approaches still suffer from performance degradation primarily caused by feature quantization in ultra-low bitwidth conditions. To address this issue, we propose a prepositive feature quantization framework that decouples adjacent layers and calibrates the interaction between feature and parameter quantization perturbations. Additionally, we present a feature-loss-aware optimization strategy to solve the corresponding calibration problem. To validate the effectiveness of our method, we conducted extensive experiments on the ImageNet benchmark dataset. Our approach demonstrates a noticeable improvement in PTQ performance under the 2-bit condition.
MoreTranslated text
Key words
Model compression,neural networks,post-training quantization
AI Read Science
Must-Reading Tree
Example
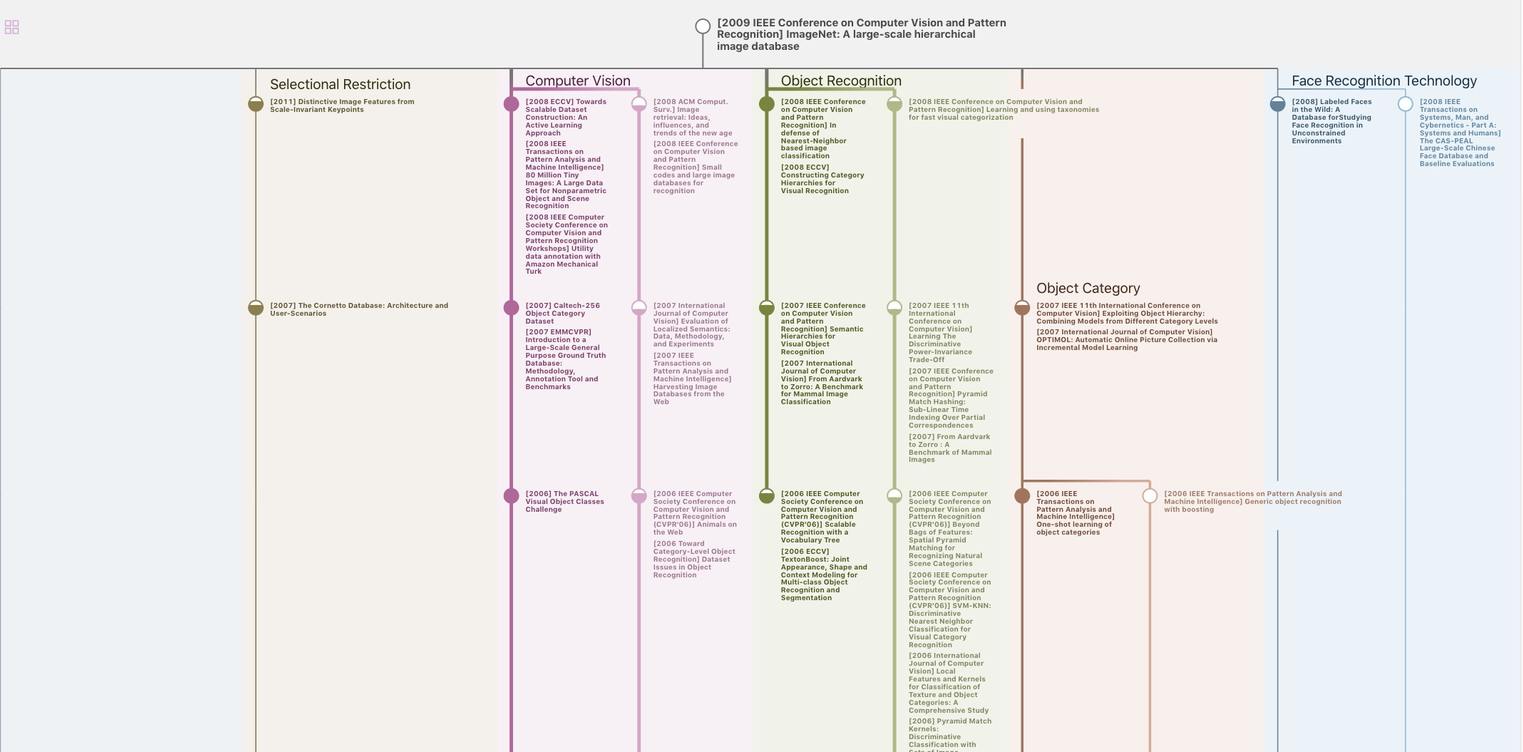
Generate MRT to find the research sequence of this paper
Chat Paper
Summary is being generated by the instructions you defined