Comparative Evaluation of Machine Learning Algorithms for Alzheimer’s Disease Classification using Synthetic Transcriptomics Dataset
Trends in Sciences(2023)
摘要
Recent technological advancements have enabled the understanding of multi-omics data, including transcriptomics, proteomics, and metabolomics. Machine learning algorithms have shown promising results in classifying multi-omics data. The objective of this paper is to evaluate the performance of machine learning algorithms in classifying transcriptomics data for Alzheimer’s disease (AD) patients and healthy control (HC) individuals. A Synthetic dataset of varying sample sizes, dimensionalities, effect sizes, and correlations was generated based on actual transcriptomics data for AD patients. The dataset consisted of 22,254 markers for 92 AD patients and 92 HC individuals. Four machine learning classifiers: naïve Bayes (NB), k-nearest neighbour (k-NN), support vector machine (SVM), and random forest (RF), were used to classify the data. The simulation was conducted using a parallel processing approach on a high-performance machine. Based on the error rate and F-measure, NB outperformed k-NN, SVM, and RF for high-dimensional data. However, SVM with a radial basis kernel (RBF) kernel performed better than NB only when the sample size was greater than 100 per group for all dimensions. The result suggests that machine learning algorithms, specifically NB, can effectively classify transcriptomics data for AD patients. SVM with an RBF kernel is a better option for large sample sizes. This study provides valuable insights for future research in the classification of transcriptomics data using machine learning algorithms. HIGHLIGHTS The performance of machine learning algorithms in classifying transcriptomics data for Alzheimer’s disease (AD) patients and healthy control (HC) individuals A synthetic dataset of varying sample sizes, dimensionalities, effect sizes, and correlations was generated based on actual transcriptomics data for AD patients Four machine learning classifiers: Naïve Bayes (NB), k-nearest neighbour (k-NN), support vector machine (SVM), and random forest (RF), were used to classify the data Based on the error rate and F-measure, NB outperformed k-NN, SVM and RF for high dimensional data. SVM with a radial basis kernel (RBF) kernel performed better than NB only when the sample size was greater than 100 per group for all dimensions GRAPHICAL ABSTRACT
更多查看译文
关键词
synthetic transcriptomics dataset,machine learning algorithms,disease classification,machine learning
AI 理解论文
溯源树
样例
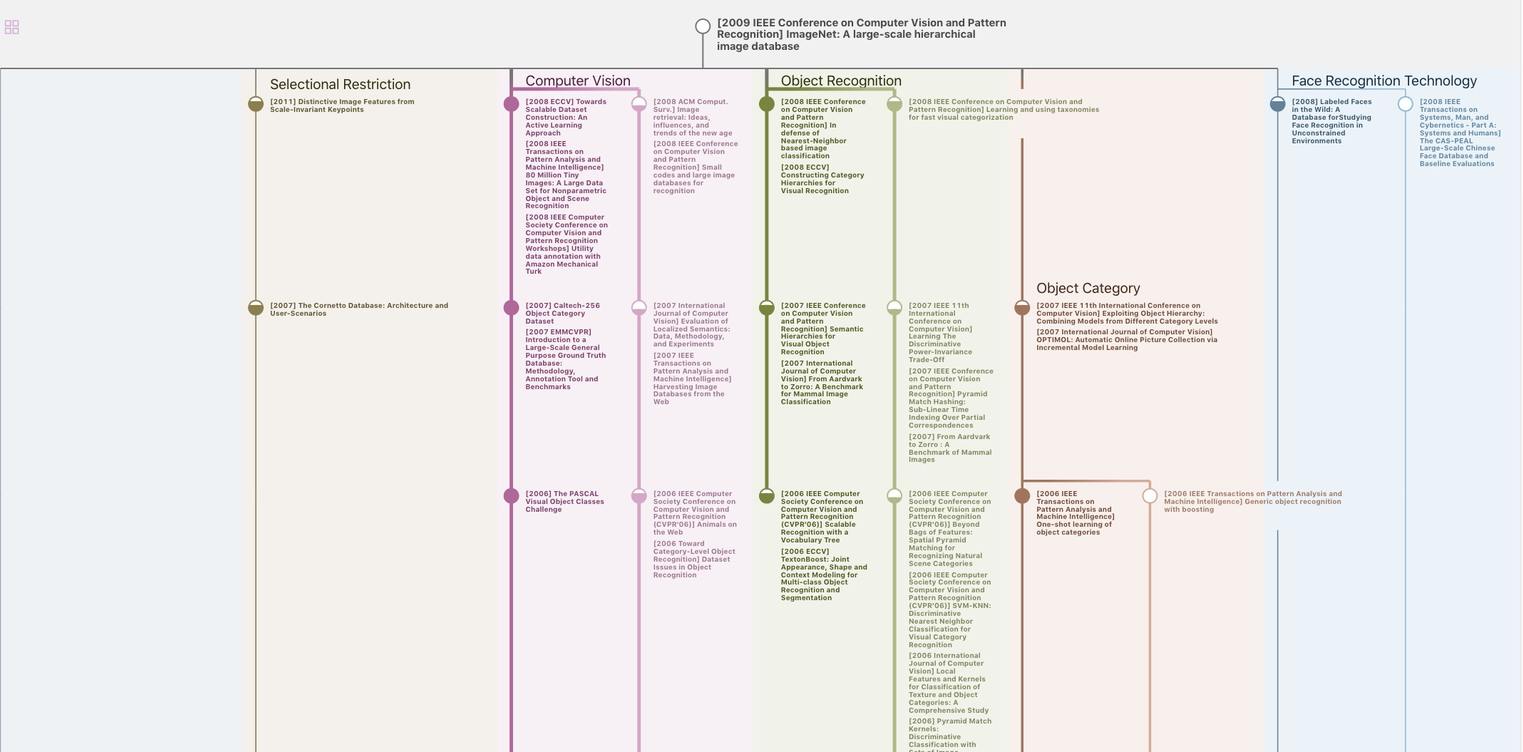
生成溯源树,研究论文发展脉络
Chat Paper
正在生成论文摘要