Depth-selective method for time-domain diffuse reflectance measurements: validation study of the dual subtraction technique
BIOMEDICAL OPTICS EXPRESS(2023)
摘要
Research on the spatial distribution of sensitivity of time-domain near infrared diffuse reflectance measurement is reported in this paper. The main objective of the investigation is to validate theoretically calculated sensitivity profiles for a measurement geometry with two detectors and two sources in which sensitivity profiles of statistical moments of distributions of time of flight of photons (DTOFs) are spatially restricted to a region underneath the detectors. For this dual subtraction method, smaller sensitivities to changes appearing in the superficial layer of the medium were observed compared to the single distance and single subtraction methods. Experimental validation of this approach is based on evaluation of changes in the statistical moments of DTOFs measured on a liquid phantom with local absorption perturbations. The spatial distributions of sensitivities, depth-related sensitivity and depth selectivities were obtained from the dual subtraction method and compared with those from single distance and single subtraction approaches. Also, the contrast to noise ratio (CNR) was calculated for the dual subtraction technique and combined with depth selectivity in order to assess the overall performance (product of CNR and depth selectivity) of the method. Spatial sensitivity profiles from phantom experiments are in a good agreement with the results of theoretical studies and feature more locally restricted sensitivity volume with the point of maximal sensitivity located deeper. The highest value of overall performance was obtained experimentally for the second statistical moment in the dual subtraction method (-10.8) surpassing that of the single distance method (-8.7). This confirms the advantage of dual subtraction measurement geometries in the suppression of optical signals originated in the superficial layer of the medium.
更多查看译文
AI 理解论文
溯源树
样例
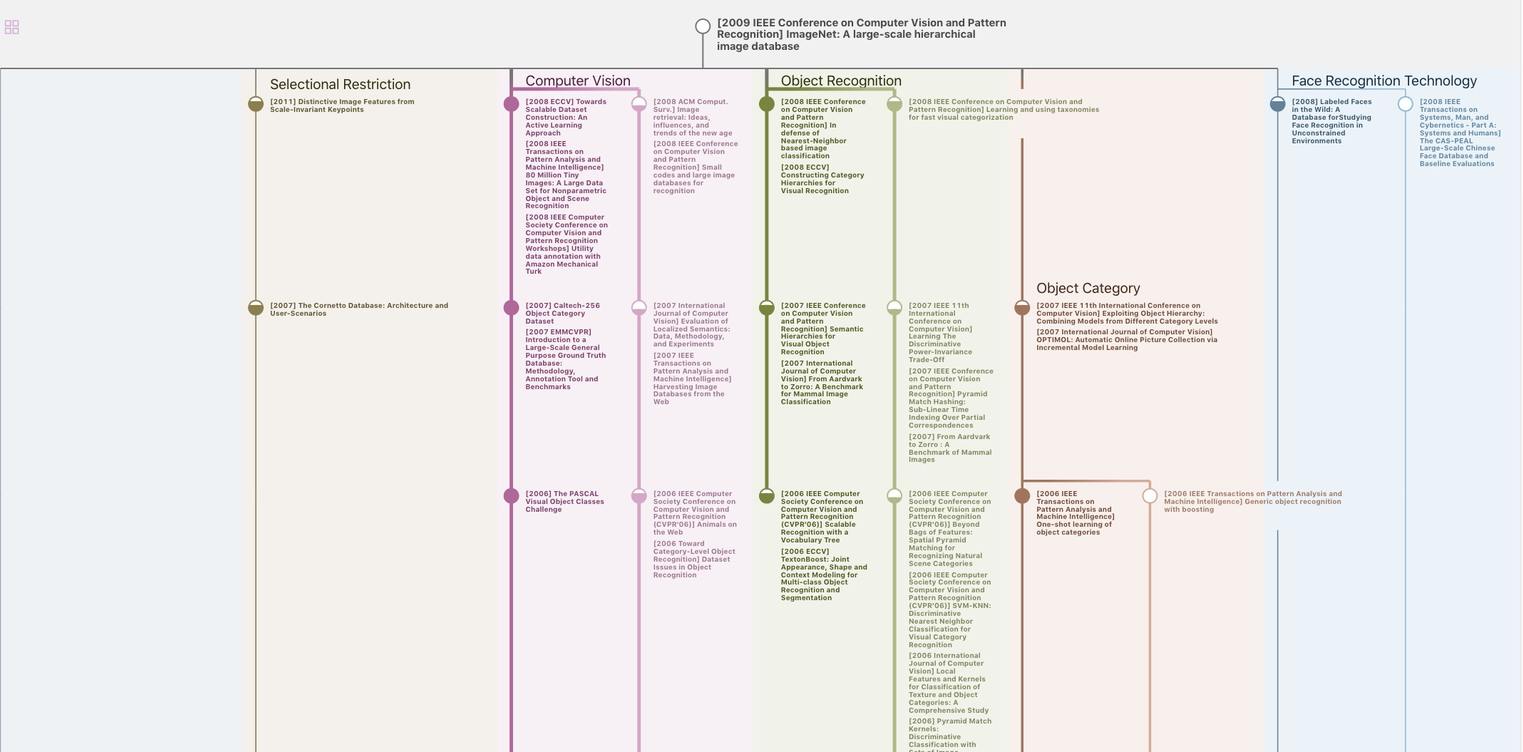
生成溯源树,研究论文发展脉络
Chat Paper
正在生成论文摘要