Time-lapse Seismic Matching for CO2 Plume Detection via Correlation-based Recurrent Attention Network
IEEE Transactions on Geoscience and Remote Sensing(2023)
摘要
Time-lapse seismic data analysis is an effective technique for monitoring reservoir changes and plays an important role in CO 2 plume detection. Theoretically, during CO 2 geological storage, time-lapse seismic data corresponding to non-reservoirs should be consistent. However, owing to changes in near-surface velocity, differences in the position of sources and receivers, and different acquisition parameters and instruments, the consistency of time-lapse data is poor, which seriously affects the application of time-lapse data. To solve this problem, a correlation-based recurrent attention network (CRAN) is proposed for consistency matching of time-lapse data. Cross-correlation is a direct measure of the correlation between seismic traces. The improved loss function of the network based on cross-correlation is beneficial for improving the matching accuracy. Recurrent neural networks can learn sequential relations and suitable for predicting time series. Therefore, recurrent neural networks are combined with convolutional neural networks to improve the network time sensitivity. Treating a large number of extracted features equally is not conducive to effective information recognition; thus, a channel attention mechanism is introduced to assign different weights to the features, thereby improving the contribution of useful information. The test results of the synthetic and field data show that the proposed CRAN has an excellent enhancing effect on the repeatability of time-lapse data, and the matched data clearly reveal a plume of CO 2 .
更多查看译文
关键词
plume detection,recurrent attention network,time-lapse,correlation-based
AI 理解论文
溯源树
样例
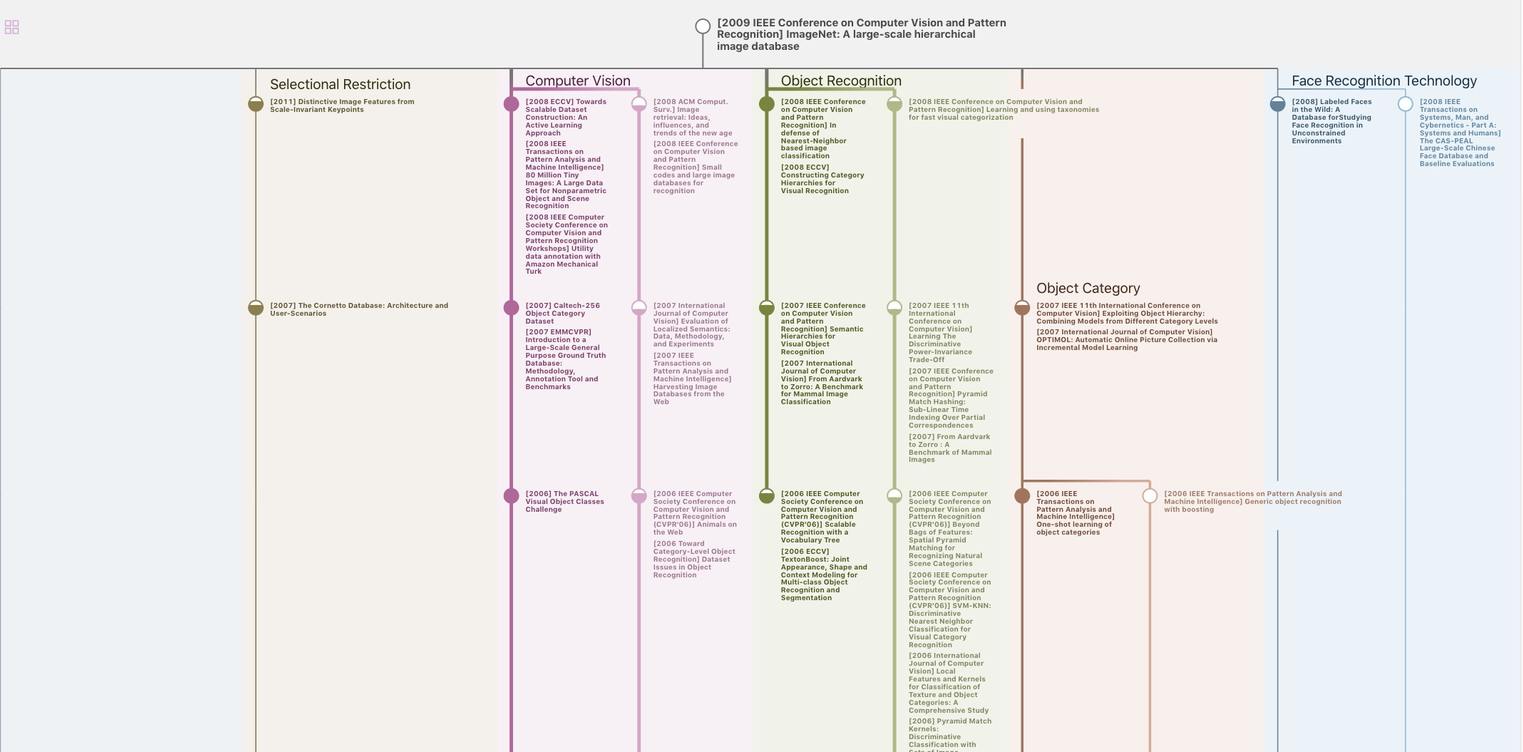
生成溯源树,研究论文发展脉络
Chat Paper
正在生成论文摘要