A Novel Multi-fidelity Surrogate Model with Two-Stage Ensemble
Lecture notes in electrical engineering(2023)
摘要
In the engineering optimization, High-fidelity (HF) information is typically more precise than low-fidelity (LF) information. Nevertheless, LF tends to be more computationally efficient than HF. In order to fully integrate the benefits of both HF and LF information, this work proposes a novel multi-fidelity surrogate (MFS) model with two-stage ensemble. In the first stage, multiple scale factors are determined by the feasible region to further build the MFS model library. Then, in the second stage, the weight coefficient is determined based on cross validation error, so as to realize the construction of ensemble MFS model. The performance of proposed MFS model is evaluated across twenty test functions. The findings indicate that the proposed MFS model exhibits excellent prediction accuracy and robustness. More importantly, in comparison to other MFS models, the developed model demonstrates a higher priority. Moreover, the study examines the impact of critical parameters on the proposed model's performance. The research presents a novel approach to reduce simulation cost in the engineering design and optimization.
更多查看译文
关键词
model,multi-fidelity,two-stage
AI 理解论文
溯源树
样例
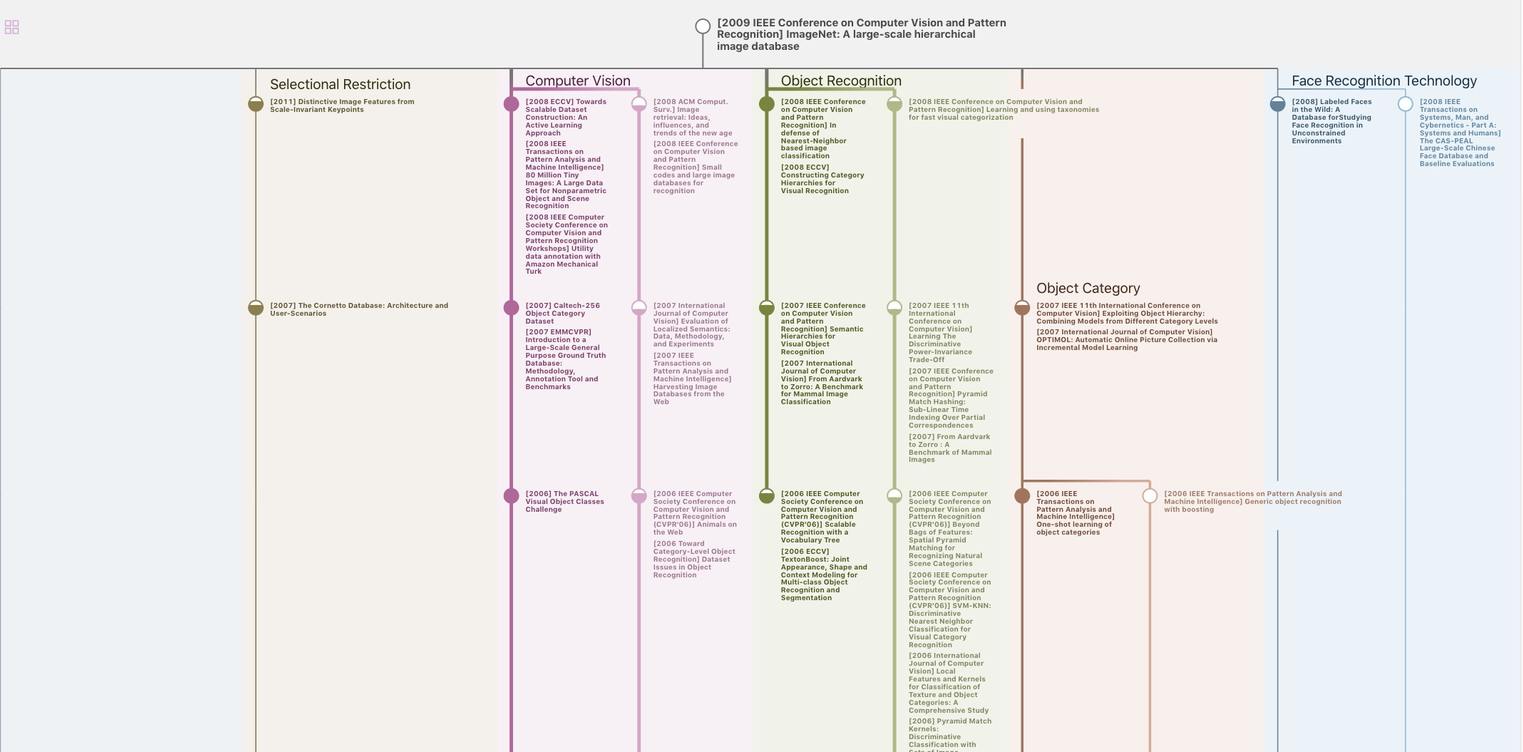
生成溯源树,研究论文发展脉络
Chat Paper
正在生成论文摘要