Graph l₁-Laplacians Regularized GMM for Hyperspectral Unmixing
IEEE Geoscience and Remote Sensing Letters(2023)
摘要
Hyperspectral unmixing (HU) based on linear mixing model (LMM) has received much attention in the remote sensing community over the past decades. However, many HU algorithms are based on the fixed endmember and do not fully exploit the inherent spatial characteristics of the hyperspectral image (HSI). In this letter, a new HU algorithm named GLR l1 -GMM is proposed to solve these problems. We use Gaussian mixture model (GMM) to represent the endmember variability. Then, considering the local smoothness in the abundance map, a graph Laplacian regularization based on l 1 -norm (GLR l1 ) is embedded in the prior of abundance. Under the Bayesian framework, the objective density function leads to a maximum a posterior (MAP) problem, which can be solved by a generalized expectation-maximization (GEM) algotithm. Experiments on two real datasets demonstrates the effectiveness of proposed algorithm compared with other state-of-the-art methods. The source code is available at https://github.com/lwdinwhu/GMM-GLRl1.
更多查看译文
关键词
hyperspectral unmixing,gmm
AI 理解论文
溯源树
样例
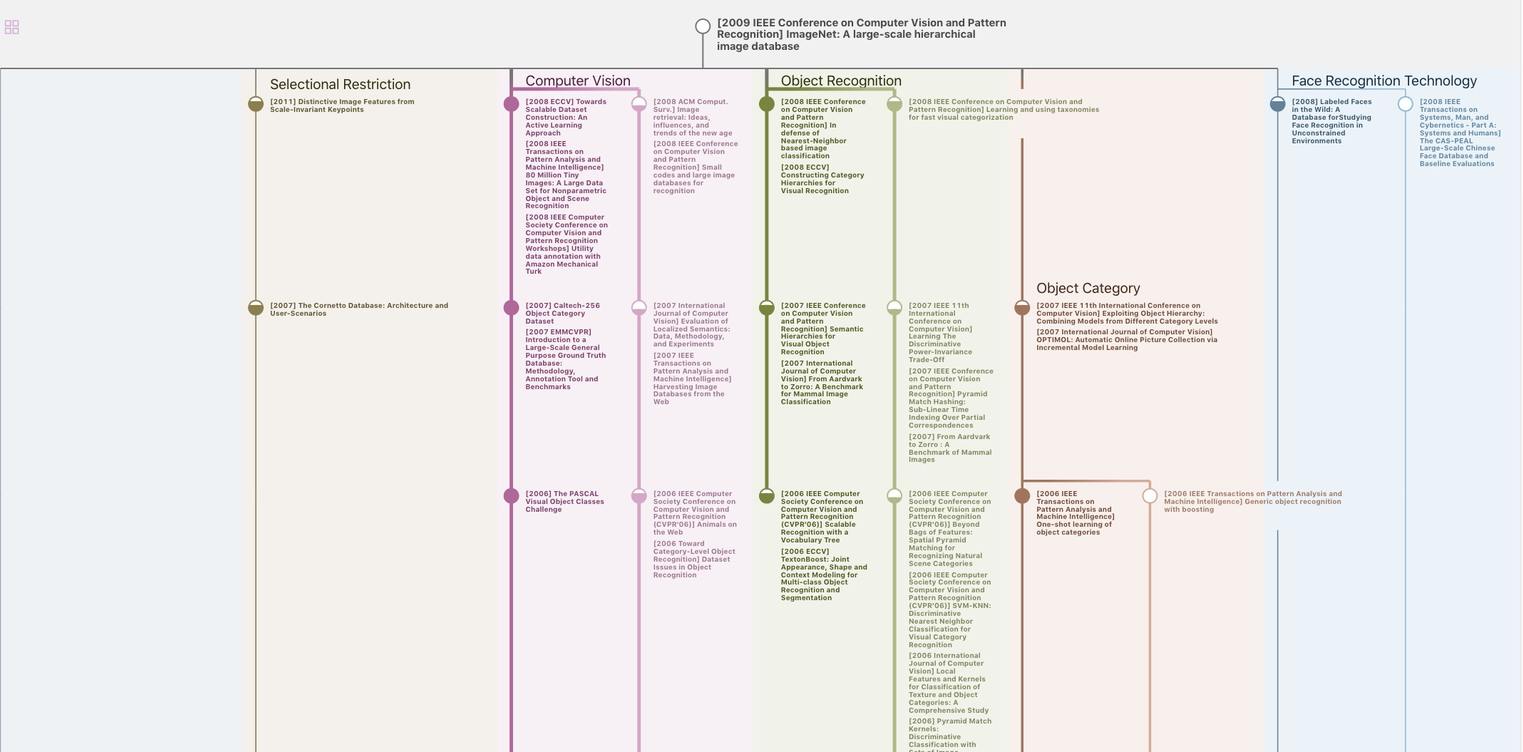
生成溯源树,研究论文发展脉络
Chat Paper
正在生成论文摘要