Multi-Scale Visual Perception Based Progressive Feature Interaction Network for Stereo Image Super-Resolution
IEEE TRANSACTIONS ON CIRCUITS AND SYSTEMS FOR VIDEO TECHNOLOGY(2024)
摘要
In recent years, stereo image super-resolution based on convolutional neural network has been extensively researched and achieved impressive performance by introducing complementary information from another view. However, most existing methods still cannot fully capture both intra- and cross-view information due to the neglect of multi-scale information perception, multi-scale binocular alignment and the excitation of large scale to small scale in human vision system. And they generated blurry results due to the consideration of irrelevant information in search for cross-view information. To address these issues, we propose a multi-scale visual perception based progressive feature interaction network (MS-PFINet) for stereo image super-resolution. Specifically, to exploit comprehensive intra- and cross-view information for image reconstruction, we design a two-stream network with multi-branch structure to extract multi-scale features and progressively use cross-view interaction at larger scales to guide that at smaller scales. Moreover, to explore more proper and accurate cross-view information, we propose a feature transformer module (FTM) to search and transfer the most relevant features from another view by hard attention maps and soft attention maps, which are calculated by patch-wise similarity rather than pixel-wise. In addition, in order to encourage a more effective way to transfer texture features for the target view, we propose a perceptual texture matching loss to supervise the accuracy of feature transformer modules. Experimental results show that our proposed method is superior to the state-of-the-art methods in most cases.
更多查看译文
关键词
Stereo image super-resolution,convolutional neural network,multi-scale,feature transformer,perceptual texture matching
AI 理解论文
溯源树
样例
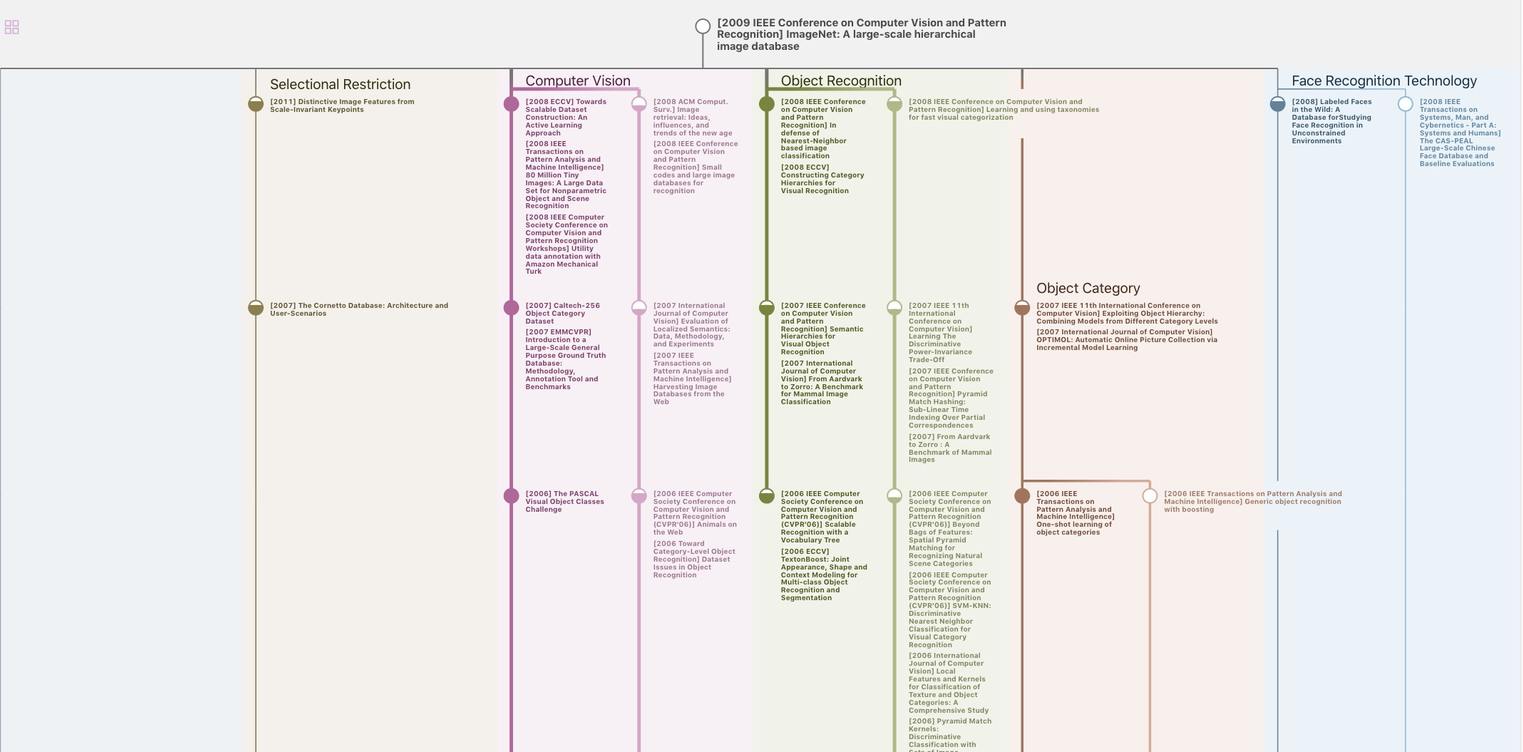
生成溯源树,研究论文发展脉络
Chat Paper
正在生成论文摘要