Multilayer random dot product graphs: Estimation and online change point detection
arXiv (Cornell University)(2023)
摘要
We study the multilayer random dot product graph (MRDPG) model, an extension of the random dot product graph to multilayer networks. By modelling a multilayer network as an MRDPG, we deploy a tensor-based method and demonstrate its superiority over existing approaches. Moving to dynamic MRDPGs, we focus on online change point detection problems. At every time point, we observe a realisation from an MRDPG. Across layers, we assume shared common node sets and latent positions but allow for different connectivity matrices. We propose efficient algorithms for both fixed and random latent position cases, minimising detection delay while controlling false alarms. Notably, in the random latent position case, we devise a novel nonparametric change point detection algorithm with a kernel estimator in its core, allowing for the case when the density does not exist, accommodating stochastic block models as special cases. Our theoretical findings are supported by extensive numerical experiments, with the code available online https://github.com/MountLee/MRDPG.
更多查看译文
关键词
random dot product graphs,online change point
AI 理解论文
溯源树
样例
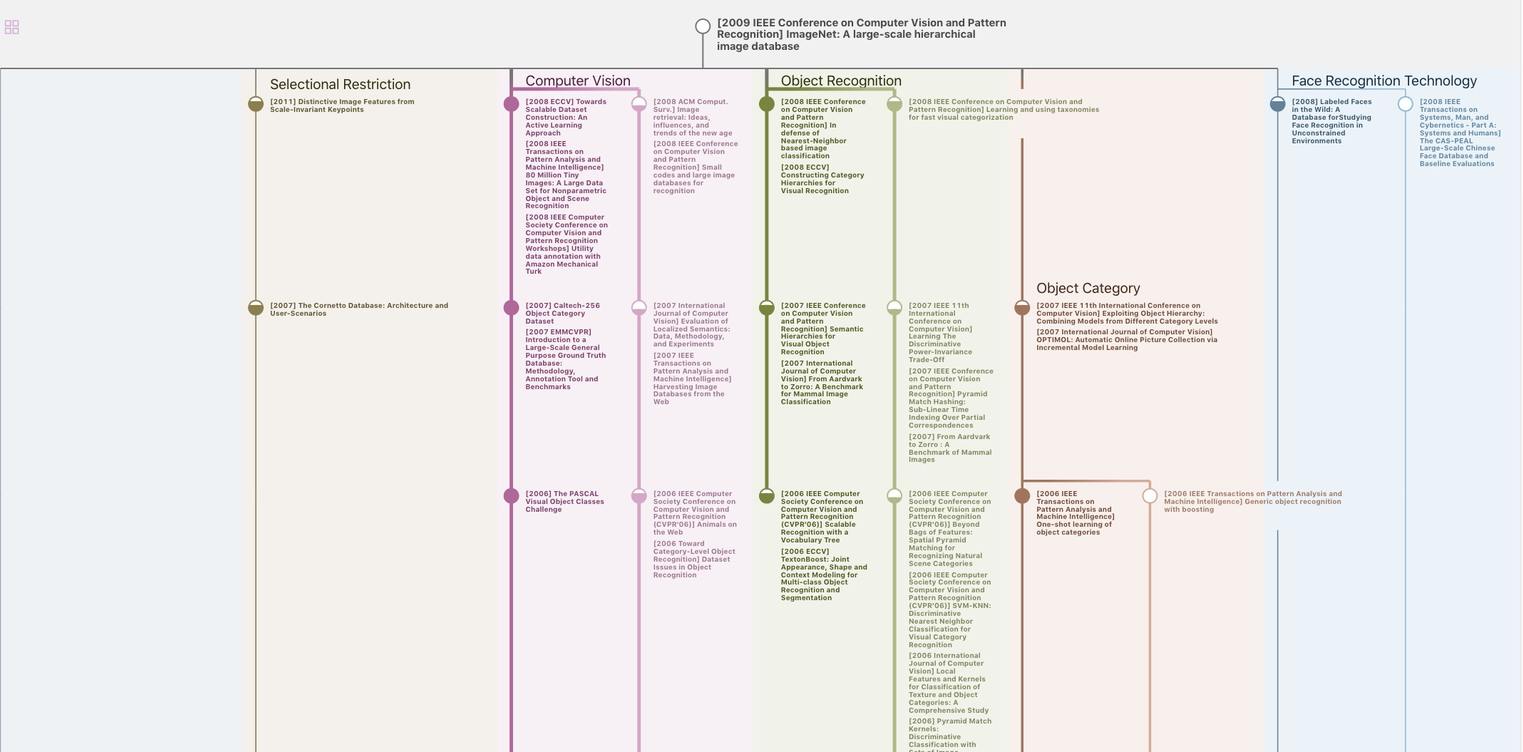
生成溯源树,研究论文发展脉络
Chat Paper
正在生成论文摘要