Breaking On-device Training Memory Wall: A Systematic Survey
arXiv (Cornell University)(2023)
摘要
On-device training has become an increasingly popular approach to machine learning, enabling models to be trained directly on mobile and edge devices. However, a major challenge in this area is the limited memory available on these devices, which can severely restrict the size and complexity of the models that can be trained. In this systematic survey, we aim to explore the current state-of-the-art techniques for breaking on-device training memory walls, focusing on methods that can enable larger and more complex models to be trained on resource-constrained devices. Specifically, we first analyze the key factors that contribute to the phenomenon of memory walls encountered during on-device training. Then, we present a comprehensive literature review of on-device training, which addresses the issue of memory limitations. Finally, we summarize on-device training and highlight the open problems for future research. By providing a comprehensive overview of these techniques and their effectiveness in breaking memory walls, we hope to help researchers and practitioners in this field navigate the rapidly evolving landscape of on-device training.
更多查看译文
关键词
memory,wall,training,on-device
AI 理解论文
溯源树
样例
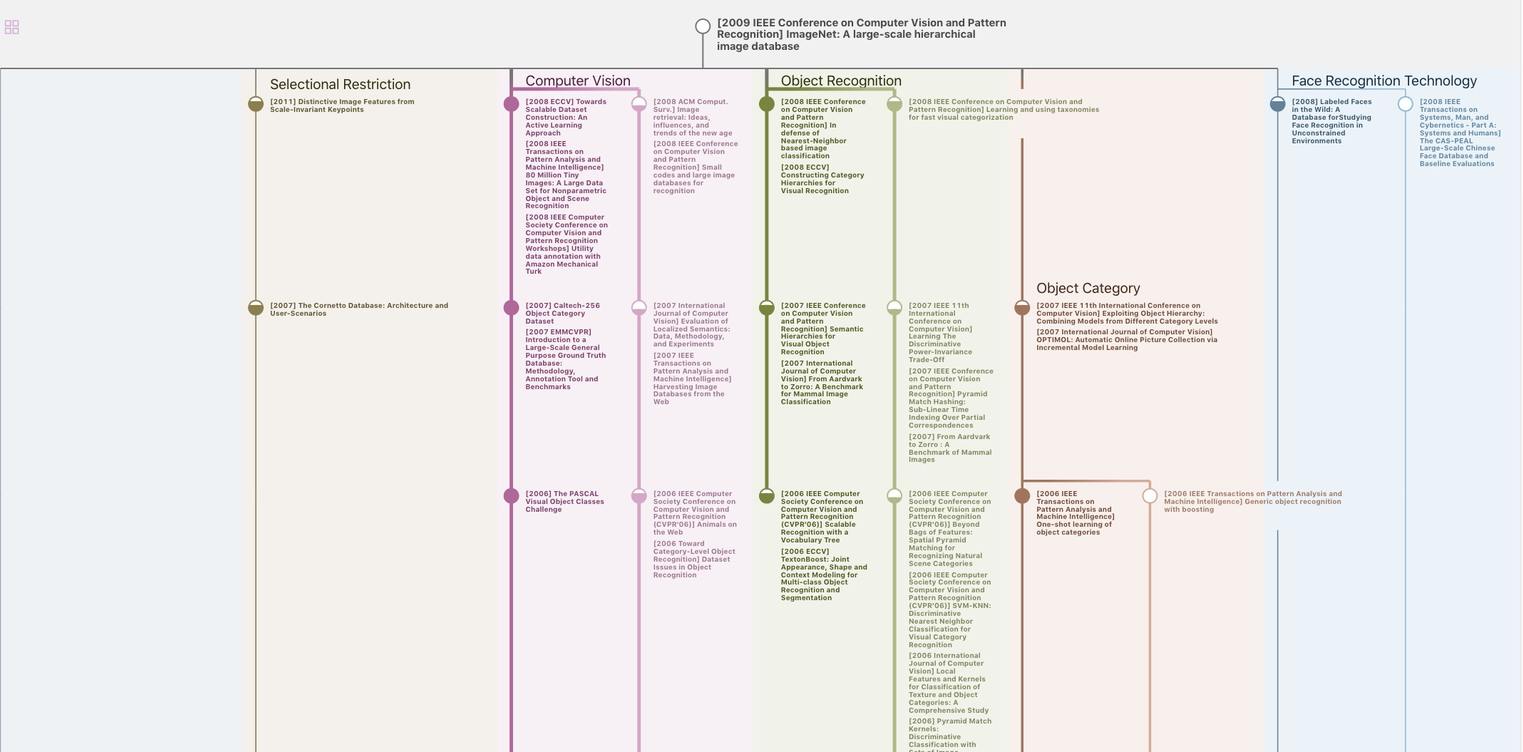
生成溯源树,研究论文发展脉络
Chat Paper
正在生成论文摘要