Energy Demand and Renewable Energy Generation Forecasting for Optimizing Dispatching Strategies of Virtual Power Plants Using Time Decomposition-Based DLinear
Lecture notes in networks and systems(2023)
Abstract
The massive carbon dioxide emission leads to the greenhouse effect and is one of the main causes of climate change. To reduce the use of fossil energy and carbon dioxide emissions, more and more renewable energy power stations, such as solar and wind power, are being connected to the grid to replace part of the fuel power stations. However, due to their uncertain and intermittent nature, grid integrations significantly impact grid stability and security. Therefore, forecasting energy demand and renewable energy generation is beneficial for virtual power plants (VPP) to plan and optimize energy dispatching strategies to meet user power demand. This paper uses the latest prediction algorithm decomposition linear (DLinear) and compares it with the traditional method and the transformer-based algorithm proposed in recent years. Unlike previous algorithms, DLinear has a simple structure and can better extract trend and seasonal features through time decomposition. Experiments show that the forecast mean squared error of DLinear is 5.04% in energy demand forecasting and 5.78% in solar power generation lower than traditional ones.
MoreTranslated text
Key words
renewable energy generation forecasting,virtual power plants,dispatching strategies,energy demand,decomposition-based
AI Read Science
Must-Reading Tree
Example
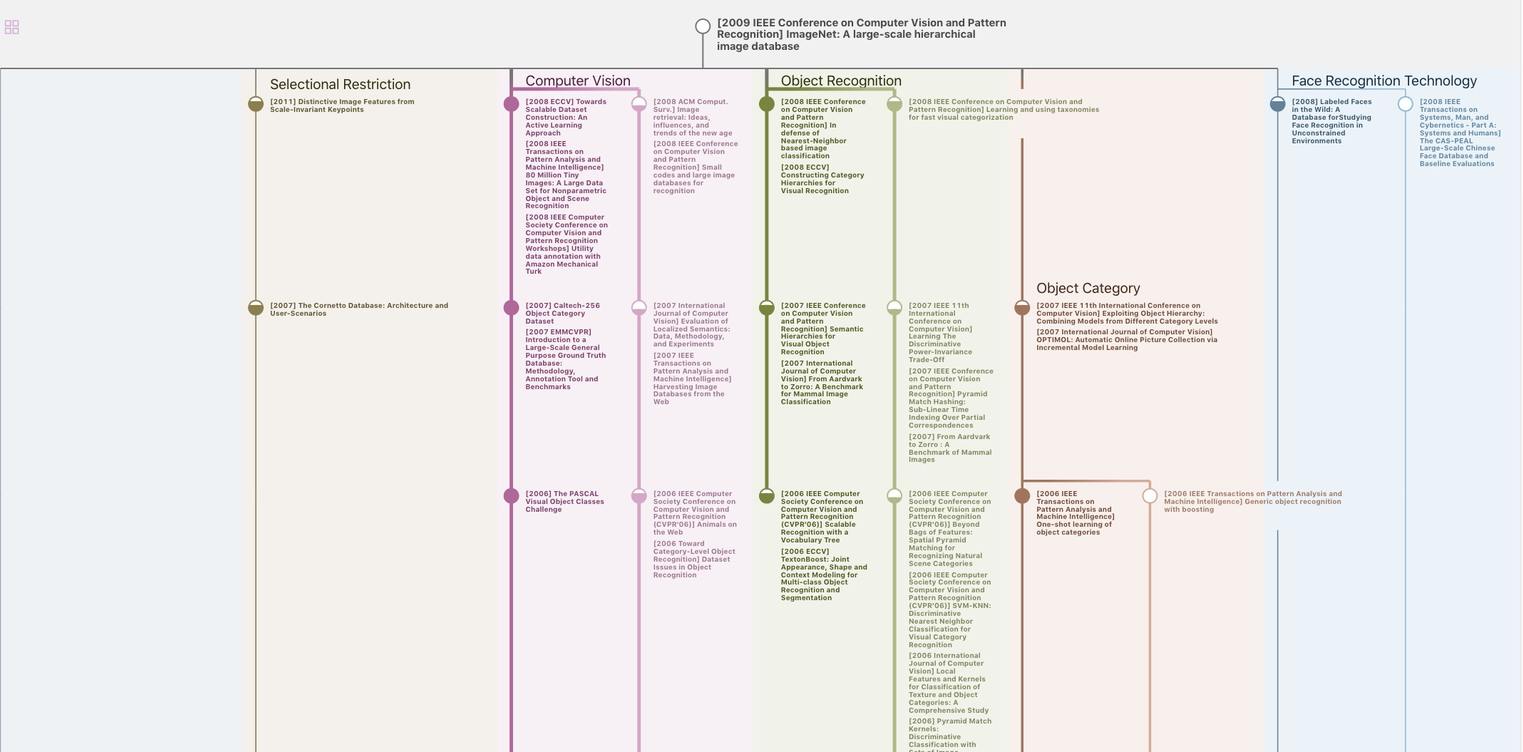
Generate MRT to find the research sequence of this paper
Chat Paper
Summary is being generated by the instructions you defined